Bayesian Hyperparameter Estimation Using Gaussian Process And Bayesian Optimization
JOURNAL OF THE PHYSICAL SOCIETY OF JAPAN(2019)
摘要
To reduce the computational cost of Bayesian inference, we propose a method to estimate the distribution of the Bayesian posterior probability from a small number of samples using a Gaussian process and Bayesian optimization. Our aim was to estimate the hyperparameter distribution of a Markov random field (MRF) model that corresponds to a lattice gas model. We used a small number of samples for the Gaussian process and estimated the distribution. In addition, we applied Bayesian optimization as a sampler for the Gaussian process and estimated the distribution from fewer sampled points than random sampling. Numerical experiments showed that our method can estimate the distribution of the Bayesian posterior probability accurately while reducing the computational cost.
更多查看译文
AI 理解论文
溯源树
样例
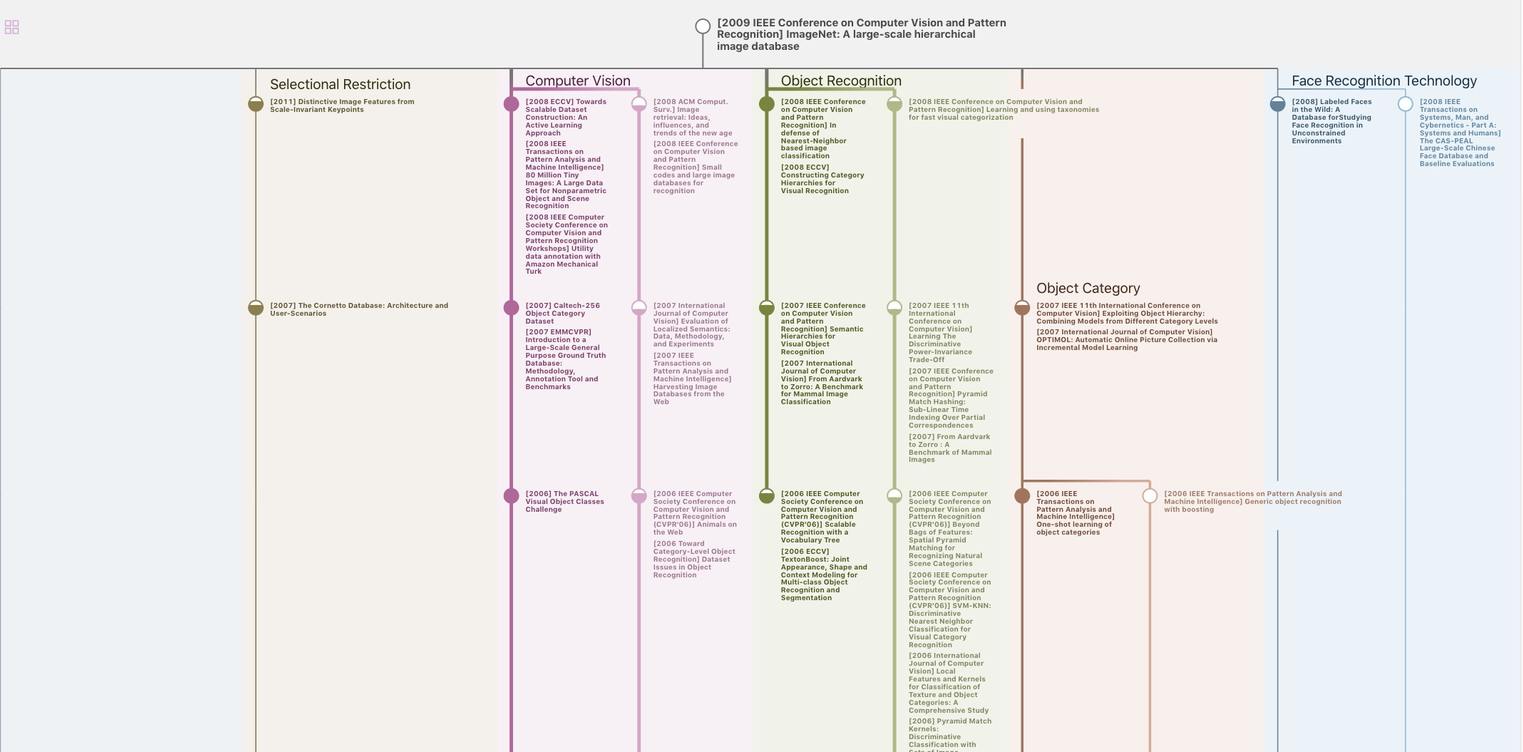
生成溯源树,研究论文发展脉络
Chat Paper
正在生成论文摘要