Evaluation of data-driven models (SVR and ANN) for groundwater-level prediction in confined and unconfined systems
Environmental Earth Sciences(2019)
摘要
Modeling the behavior of groundwater levels is necessary to implement sustainable groundwater resource management. Groundwater is a non-linear and complex system, which can be modeled by data-driven models. This study evaluates the performances of data-driven models, support vector machine regression (SVR) and artificial neural network (ANN), for forecasting groundwater levels of confined and unconfined systems at 1-, 2-, and 3-month ahead. This is the first time that confined and unconfined aquifers have been compared using data-driven models. In addition, to identify the optimal input combination, a hybrid gamma test (GT) and genetic algorithm (GA) was used. The coefficient of correlation ( R ), Mean Absolute Error (MAE), root mean squared error (RMSE), Nash–Sutcliffe efficiency (NSE), and developed discrepancy ratio (DDR) were applied to evaluate the SVR and ANN models. Results showed that the SVR and ANN models were more accurate for the unconfined system than the confined system for forecasts up to 3-month ahead. In both hydrogeological systems for 1-month ahead, the models performed better than for 2- and 3-month ahead forecasts, and the accuracy of the models decreased as the months ahead increased. The SVR model performed better than the ANN model for 1-, 2-, and 3-month ahead groundwater-level forecasting. The SVR model could be successfully used in predicting monthly groundwater in confined and unconfined systems.
更多查看译文
关键词
Groundwater level,Data-driven models,Unconfined,Confined,Gamma test
AI 理解论文
溯源树
样例
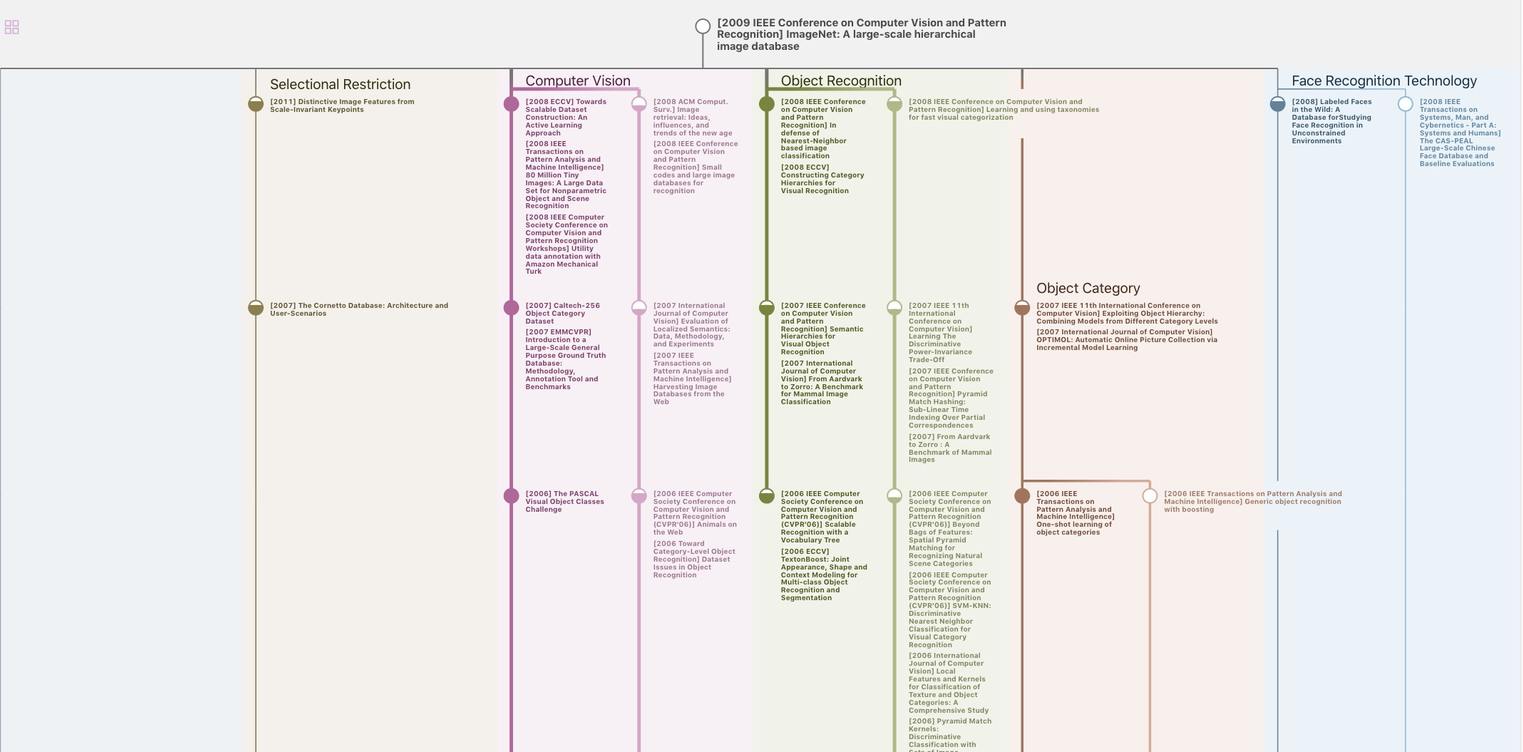
生成溯源树,研究论文发展脉络
Chat Paper
正在生成论文摘要