A Hybrid Deep Reinforcement Learning Algorithm For Intelligent Manipulation
INTELLIGENT ROBOTICS AND APPLICATIONS, ICIRA 2019, PT IV(2019)
Abstract
Conventional collaborative robots can solve complex problems through programming approaches. But the current tasks are different and non-repetitive, many problems cannot be solved by conventional programming methods. Deep reinforcement learning provides a framework for solving robotic control tasks using machine learning techniques. However, the existing model-free deep reinforcement learning algorithms lack unified framework for comparing sample efficiency with final performance. In this paper, a hybrid deep reinforcement learning framework and its application in robot control are proposed based on the existing model-free deep reinforcement learning algorithms. In the acting process, the distributed actors acting with the environment are used to acquire the data, while prior actors are used to solve the cold boot problem of the algorithm. In the learning process, prioritized experience replay and multistep learning are designed for the improvement on the final performance. Simulations are represented to show the practicality and potential of the proposed algorithm. Results show that the hybrid deep reinforcement learning algorithm in this paper has a significant improvement on the final performance and sample efficiency while it can ensure the stability and convergence.
MoreTranslated text
Key words
Deep reinforcement learning, Robot control, Data flow, Hybrid deep reinforcement learning
AI Read Science
Must-Reading Tree
Example
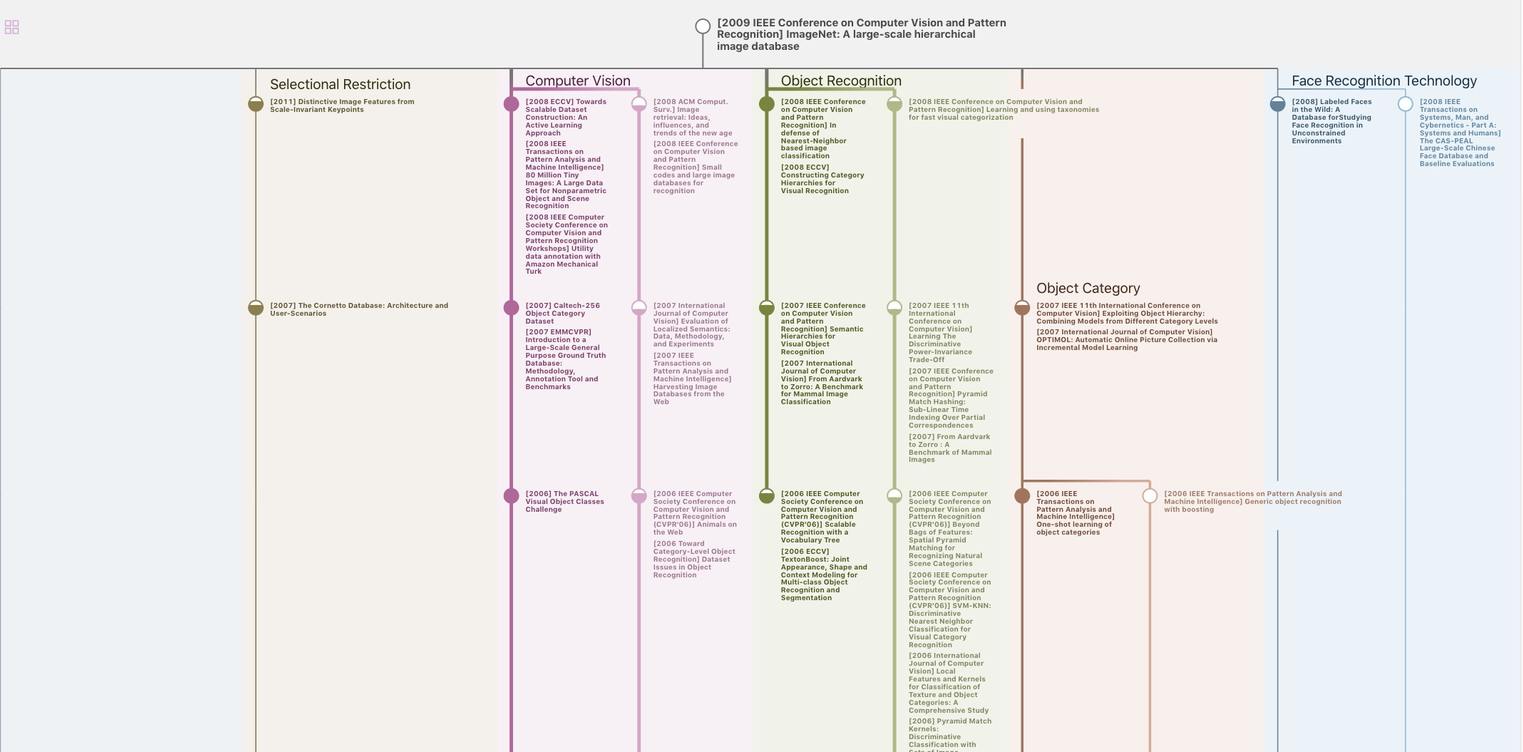
Generate MRT to find the research sequence of this paper
Chat Paper
Summary is being generated by the instructions you defined