A non-canonical hybrid metaheuristic approach to adaptive data stream classification
Future Generation Computer Systems(2020)
摘要
Data stream classification techniques have been playing an important role in big data analytics recently due to their diverse applications (e.g. fraud and intrusion detection, forecasting and healthcare monitoring systems) and the growing number of real-world data stream generators (e.g. IoT devices and sensors, websites and social network feeds). Streaming data is often prone to evolution over time. In this context, the main challenge for computational models is to adapt to changes, known as concept drifts, using data mining and optimisation techniques. We present a novel ensemble technique called RED-PSO that seamlessly adapts to different concept drifts in non-stationary data stream classification tasks. RED-PSO is based on a three-layer architecture to produce classification types of different size, each created by randomly selecting a certain percentage of features from a pool of features of the target data stream. An evolutionary algorithm, namely, Replicator Dynamics (RD), is used to seamlessly adapt to different concept drifts; it allows good performing types to grow and poor performing ones to shrink in size. In addition, the selected feature combinations in all classification types are optimised using a non-canonical version of the Particle Swarm Optimisation (PSO) technique for each layer individually. PSO allows the types in each layer to go towards local (within the same type) and global (in all types) optimums with a specified velocity. A set of experiments are conducted to compare the performance of the proposed method to state-of-the-art algorithms using real-world and synthetic data streams in immediate and delayed prequential evaluation settings. The results show a favourable performance of our method in different environments.
更多查看译文
关键词
Ensemble learning,Data stream mining,Concept drifts,Bio-inspired algorithms,Non-stationary environments,Particle swarm optimisation,Replicator dynamics
AI 理解论文
溯源树
样例
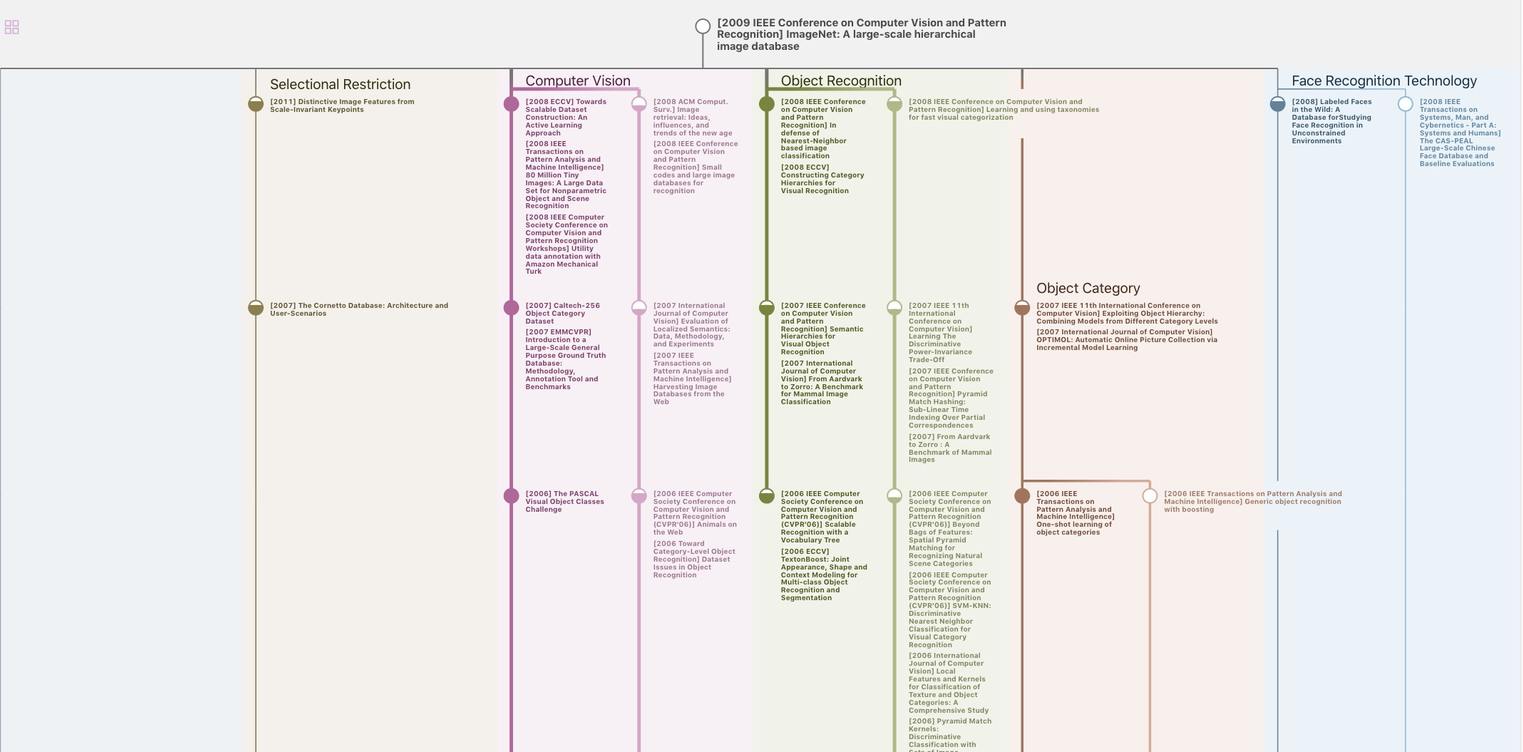
生成溯源树,研究论文发展脉络
Chat Paper
正在生成论文摘要