Fully portable and wireless universal brain–machine interfaces enabled by flexible scalp electronics and deep learning algorithm
NATURE MACHINE INTELLIGENCE(2019)
摘要
Variation in human brains creates difficulty in implementing electroencephalography into universal brain–machine interfaces. Conventional electroencephalography systems typically suffer from motion artefacts, extensive preparation time and bulky equipment, while existing electroencephalography classification methods require training on a per-subject or per-session basis. Here, we introduce a fully portable, wireless, flexible scalp electronic system, incorporating a set of dry electrodes and a flexible membrane circuit. Time-domain analysis using convolutional neural networks allows for accurate, real-time classification of steady-state visually evoked potentials in the occipital lobe. Compared to commercial systems, the flexible electronics show the improved performance in detection of evoked potentials due to significant reduction of noise and electromagnetic interference. The two-channel scalp electronic system achieves a high information transfer rate (122.1 ± 3.53 bits per minute) with six human subjects, allowing for wireless, real-time, universal electroencephalography classification for an electric wheelchair, a motorized vehicle and a keyboard-less presentation.
更多查看译文
关键词
Engineering,Materials for devices,general
AI 理解论文
溯源树
样例
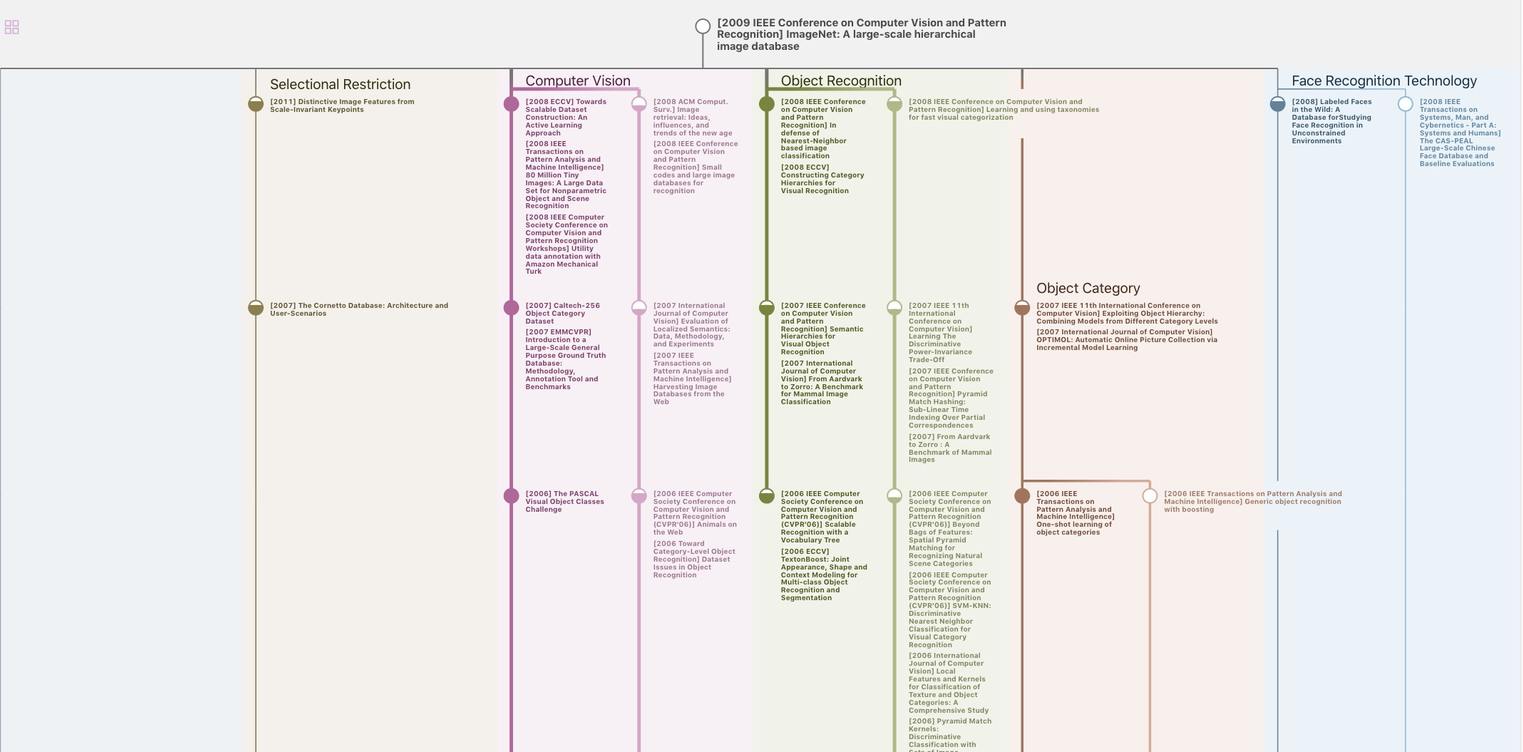
生成溯源树,研究论文发展脉络
Chat Paper
正在生成论文摘要