Reinforcement Learning for Improving the Accuracy of PM2.5 Pollution Forecast Under the Neural Network Framework.
IEEE Access(2020)
摘要
Air pollution seriously damages human health on a large scale. Although earlier works have improved a variety of predictive models of air pollution, the ability to accurately predict air pollution indices remains elusive. Time series prediction plays an important role in many fields. Some predecessors have experimented with artificial neural networks (NNs), combining linear autoregressive integrated moving average (ARIMA) models with nonlinear NN models. The typical assumption is that time series has a long signal with no white noise. However, a real-time short signal with white noise is common. The methods in the literature also do not guarantee that the prediction error of an NN model is minimized. Therefore, we propose the use of reinforcement learning (RL) to predict future PM2.5 values. First, we use the -learning algorithm in RL based on its state characteristics on the NN model. Second, we select the input with different input dimensions and values of time delay, calculate the best strategy, and evaluate the computational complexity of our RL algorithm. Finally, we show that we effectively reduce the prediction error of the NN models.
更多查看译文
关键词
Predictive models,Atmospheric modeling,Artificial neural networks,Autoregressive processes,Reinforcement learning,Data models,Smart city,smart environments,urban computing,autoregressive integrated moving average,time series,neural network,reinforcement learning,Q-learning
AI 理解论文
溯源树
样例
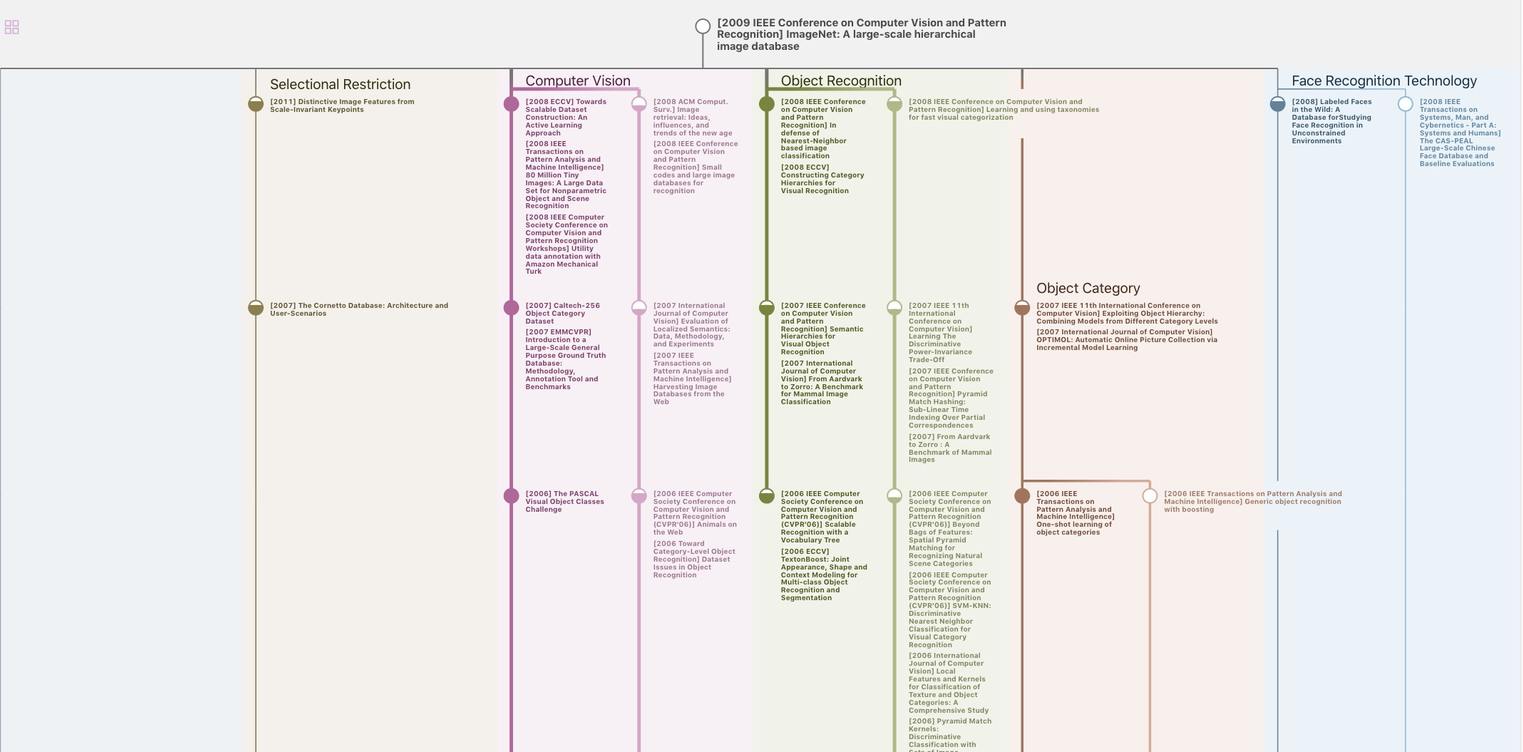
生成溯源树,研究论文发展脉络
Chat Paper
正在生成论文摘要