Experience-Centric Mobile Video Scheduling Through Machine Learning
IEEE ACCESS(2019)
摘要
Providing a high quality video streaming experience in a mobile data network via the ubiquitous HTTP Adaptive Streaming (HAS) protocol is challenging. This is largely because HAS traffic arrives as regular Internet Protocol (IP) packets, indistinguishable from those of other data services. This paper presents real-time network-based Machine Learning (ML) classifiers incurring low overhead and capable of (a) detecting the service type of different flows including HAS, and (b)detecting the player status for users with HAS flows. We utilize random forests, an ensemble classifier, relying only upon standard unencrypted packet headers. By applying the ML classifier outputs to derive scheduling metrics, we show how existing LTE basestation schedulers can improve video Quality-of-Experience (QoE) while incurring minimal overhead. For a simulated LIE cellular network, we present quantitative performance results that include misclassification errors. Our classification and scheduling framework is shown to provide an improved video QoE with tolerable impact on other non-video best effort services. These design insights can be applied to optimize video delivery in current and future wireless networks.
更多查看译文
关键词
Scheduling algorithms,streaming media,supervised learning
AI 理解论文
溯源树
样例
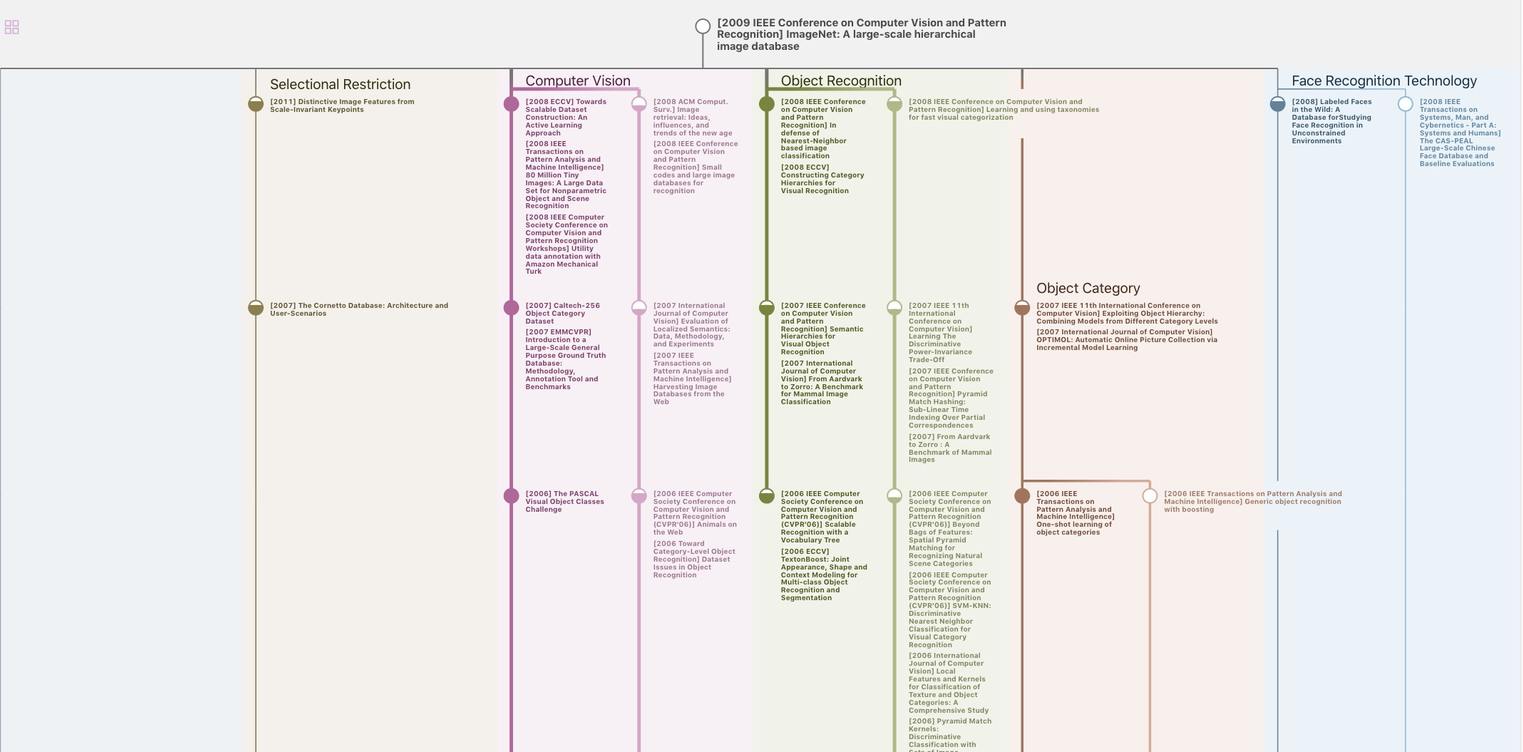
生成溯源树,研究论文发展脉络
Chat Paper
正在生成论文摘要