Reduced-Dimension Wiener Filter Based On Perpendicular Partition
IEEE ACCESS(2019)
Abstract
This paper addresses the reduced-dimension Wiener filter based on perpendicular partition. Two algorithms, i.e., cyclic per-weight (CPW) and batch CPW (BCPW) are presented, which can efficiently perform reduced-dimension adaptive beamforming. In particular, the CPW is suitable for the slow changing environments that have large snapshots to train the weight vector. The BCPW is designed for fast changing scenarios that have low training sequences and sometimes the number of snapshots is less than the number of sensors. In these two algorithms, the solutions of the weight vectors are circularly solved one by one, which only refer to scalar optimization problem then the matrix inversion is avoided. There is no intermediate transformation or orthogonal/non-orthogonal decomposition requirement in our algorithms, so their implementations are relatively simple. Convergence analysis and the computational complexities comparison are provided. Simulation results show the superiorities of the proposed algorithms. Specifically, the CPW has better convergence properties than previous schemes, and the BCPW has low computational complexity and good output SINR performance in low snapshots scenarios.
MoreTranslated text
Key words
MMSE, Wiener filter, adaptive beamforming, cyclical optimization, perpendicular partition
AI Read Science
Must-Reading Tree
Example
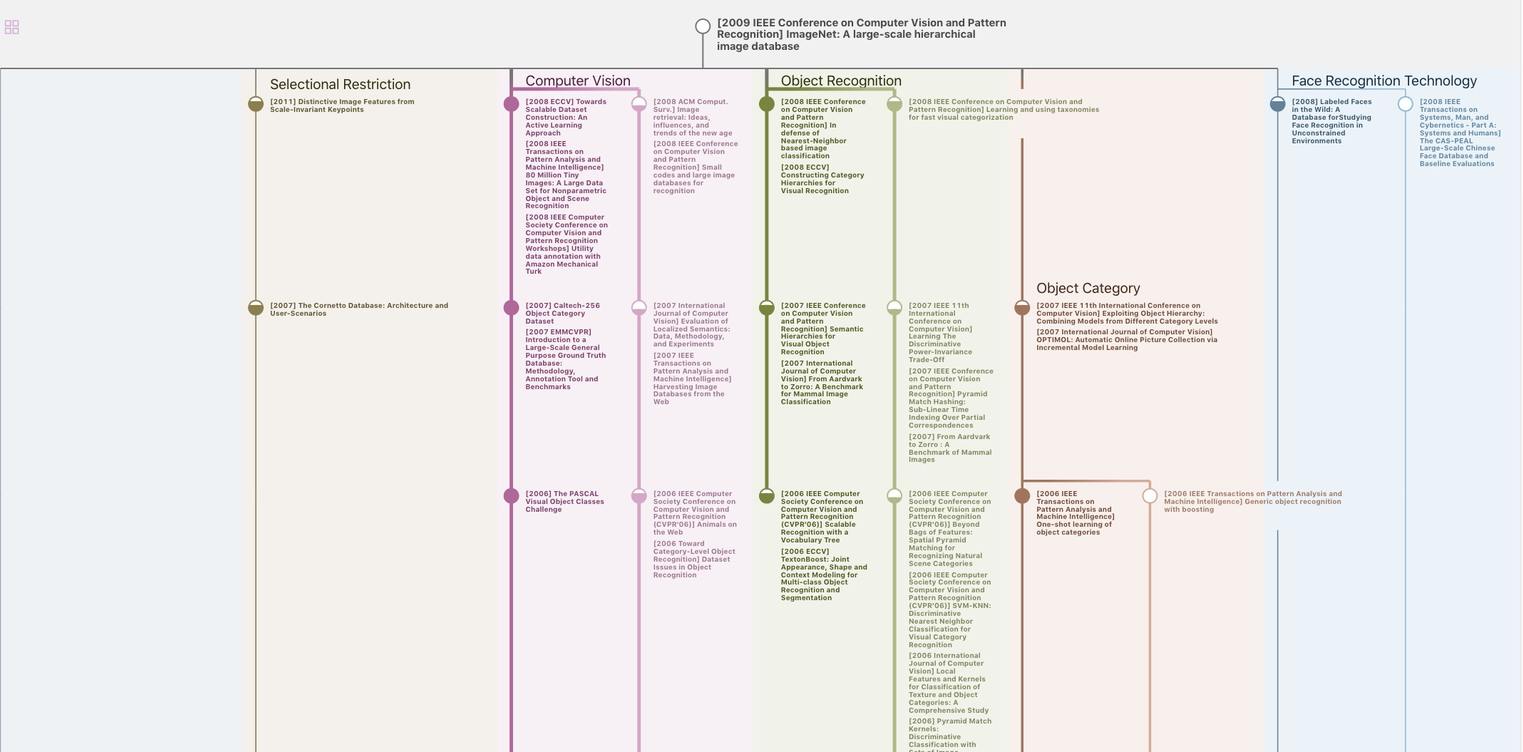
Generate MRT to find the research sequence of this paper
Chat Paper
Summary is being generated by the instructions you defined