Building The 3-1) Boundary Zones For Predicting Cellular Metabolic Phenotypes By Computation Of Time Sensitivity Factor (S) Using F-18 Fog Pet Potential Preamble Ai Algorithm For Evaluation Of Pulmonary Nodules And Lung Cancer Radiotherapy
JOURNAL OF NUCLEAR MEDICINE(2019)
摘要
1330 Aim: To build 3-D boundary zones for predicting cellular metabolic phenotypes (CMP) of pulmonary nodules (PNs) using F-18 FDG PET by computation of a time sensitivity (S) factor and implement into potential AI algorithm for diagnosis (Dx) and radiotherapy (RT) evaluation of lung cancers (CA). Methods: The initial computation was from 97 PNs (81 patients [pts]) from a US cohort (USC) referred for variable dual-time F-18 FDG PET-CT with definite pathological diagnosis or 1-year CT follow-up (gold standard, GS). S=d{ln[SUV]]/d{ln[T]} was obtained by logarithmic regression using scan times, Ti (i=0, 1, 2), and standard uptake value (SUVj) (j=0, 1, 2) with SUV0 =0.5. The actual T1 and T2 were 72+14 and 135+18 min (Skewness=2.076 and 1.356 respectively) and T0=0.5 min. The subsequent computation was from 41 pts for an Asian cohort (AC) with single PNs imaged by F-18 FDG PET at multiple exact fixed time points (T0=0.5 min; T1-3=1, 2 and 3 hr) with the same GS and technique as in USC for comparison and obtaining the weighted average S into AI algorithm for analysis. The bioinformatics from S computation was finally used to build 3D graph of boundary zones separating the underlying CMP by the zonal plane equation, z= (1+y/x)S , where x= T1, y= T2-x; SUV2 = z[asterisk]SUV1. To help CA Dx, it is necessary to know how long (y) the delayed imaging (DI) needs to be after T1 depending on initial SUV and z by y=x{exp[ln(z)/S]-1}. Without a prior clinical knowledge, the algorithm has to start with a crude PET likelihood of CMP (SUV1>2.5, S at 2 boundary planes) to determine the most useful T2 (x+y) in AI algorithm for PET Dx. Results: There were significant differences in USC between the nonmalignant (NM, n=45) and CA (n=52) groups in S (0.17±0.16 vs. 0.48±0.18, p 2.5 and S=0.45 or SUV1 1.100 (Dx cut off for CA from ROC analysis for AC), then final Dx was reached (group A). Otherwise further DI at 3 hr was needed (Group B). It was determined that if x=60 min(T1) in the AC, 24 out of 41 pts only need T2 at 98.52 min ( 3 hr; so SUV3h, last data point was used for analysis) at the boundary conditions. However, 10 out of initial 24 pts intended for 1.100. The combined group A and B contingency table was generated as: [TP: 26(A=14;B=12)/27], [TN: 13 (A=0;B=13)/14], [FN: 1(A=0;B=1)/27], [FP: 1(A=0;B=1)/14], yielding the overall sensitivity, specificity and accuracy 96, 93 and 95% respectively using this simple AI algorithm. The same algorithm may be useful in evaluation to residual CA cells after RT. As S and its corrected retention index, RIs=[(T2/T1)S- 1] [asterisk] 100%) had been validated previously on micro-PET data during weekly evaluation after hypo-fractionated RT of human lung cancer xenograft to predict tumor control. If one extends this translational research to clinical applications, the initial pre-treatment PET scan may be done at the standard 1- and 2-hr interval at the expected mean RIs = (20.45 - 1) x 100% =37% for CA. After RT, the dual time PET may need to prolong at least or beyond 3 hr to bring out the fundamental changes in CMP from CA cells to fibroblasts and/inflammatory cells due to potentially low SUV and S according to the results of current study with similar AI algorithm. Conclusions: 3-D boundary zones from S computation are useful in AI algorithm for evaluation of CMP of PNs and RT of CA lung.
更多查看译文
关键词
predicting cellular metabolic phenotypes,lung cancer,lung cancer radiotherapy,time sensitivity factor,algorithm
AI 理解论文
溯源树
样例
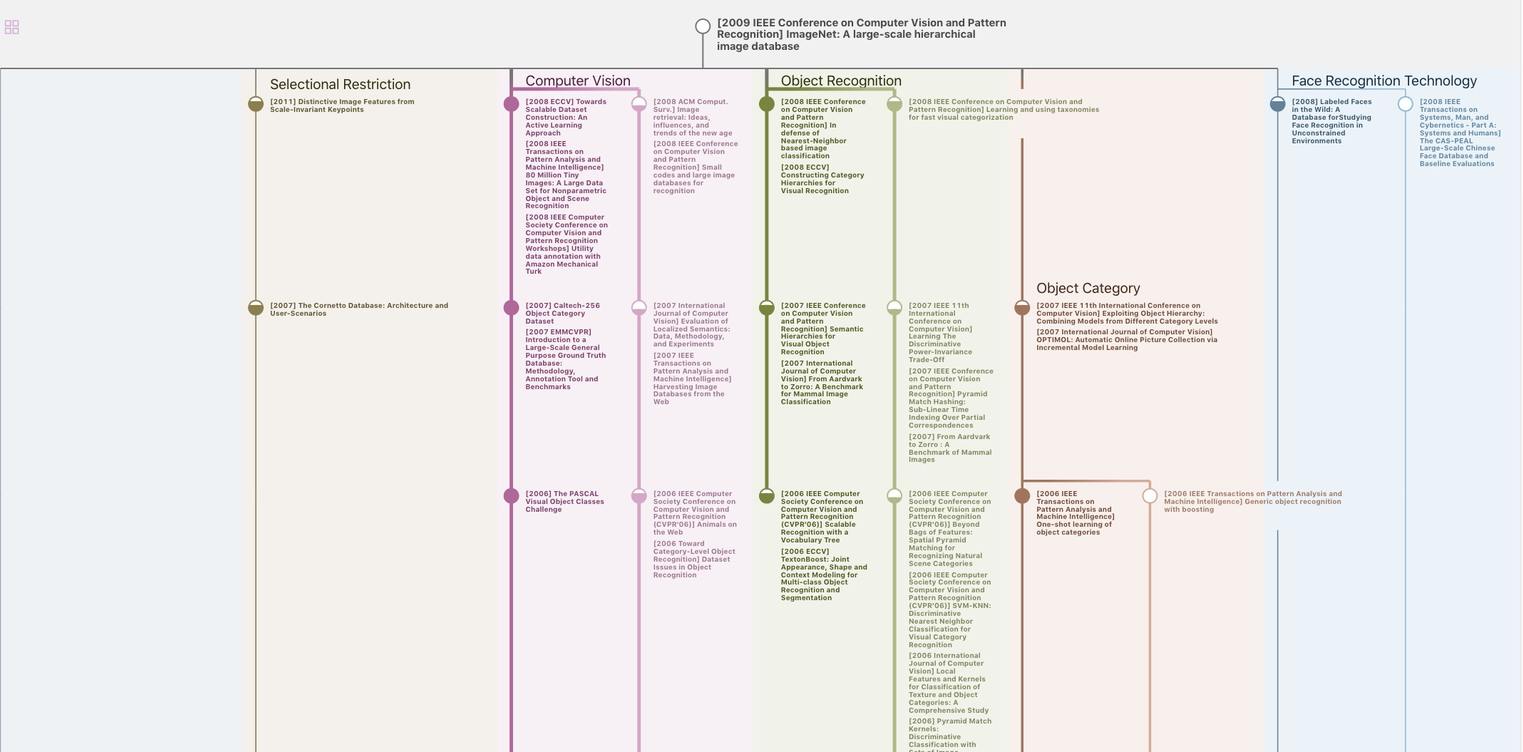
生成溯源树,研究论文发展脉络
Chat Paper
正在生成论文摘要