Representation Learning In Heterogeneous Professional Social Networks With Ambiguous Social Connections
2019 IEEE INTERNATIONAL CONFERENCE ON BIG DATA (BIG DATA)(2019)
摘要
Network representations have been shown to improve performance within a variety of tasks, including classification, clustering, and link prediction. However, most models either focus on moderate-sized, homogeneous networks or require a significant amount of auxiliary input to be provided by the user. Moreover, few works have studied network representations in real-world heterogeneous social networks with ambiguous social connections and arc often incomplete. In the present work, we investigate the problem of learning low-dimensional node representations in heterogeneous professional social networks (HPSNs), which are incomplete and have ambiguous social connections. We present a general heterogeneous network representation learning model called Star2Vec that learns entity and person embeddings jointly using a social connection strength-aware biased random walk combined with a node-structure expansion function. Experiments on LinkedIn's Economic Graph and publicly available snapshots of Facebook's network show that Star2Vec outperforms existing methods on members' industry and social circle classification, skill and title clustering, and member-entity link predictions. We also conducted large-scale case studies to demonstrate practical applications of the Star2Vec embeddings trained on LinkedIn's Economic Graph such as next career move, alternative career suggestions, and general entity similarity searches.
更多查看译文
关键词
Network representation, Heterogeneous professional social networks
AI 理解论文
溯源树
样例
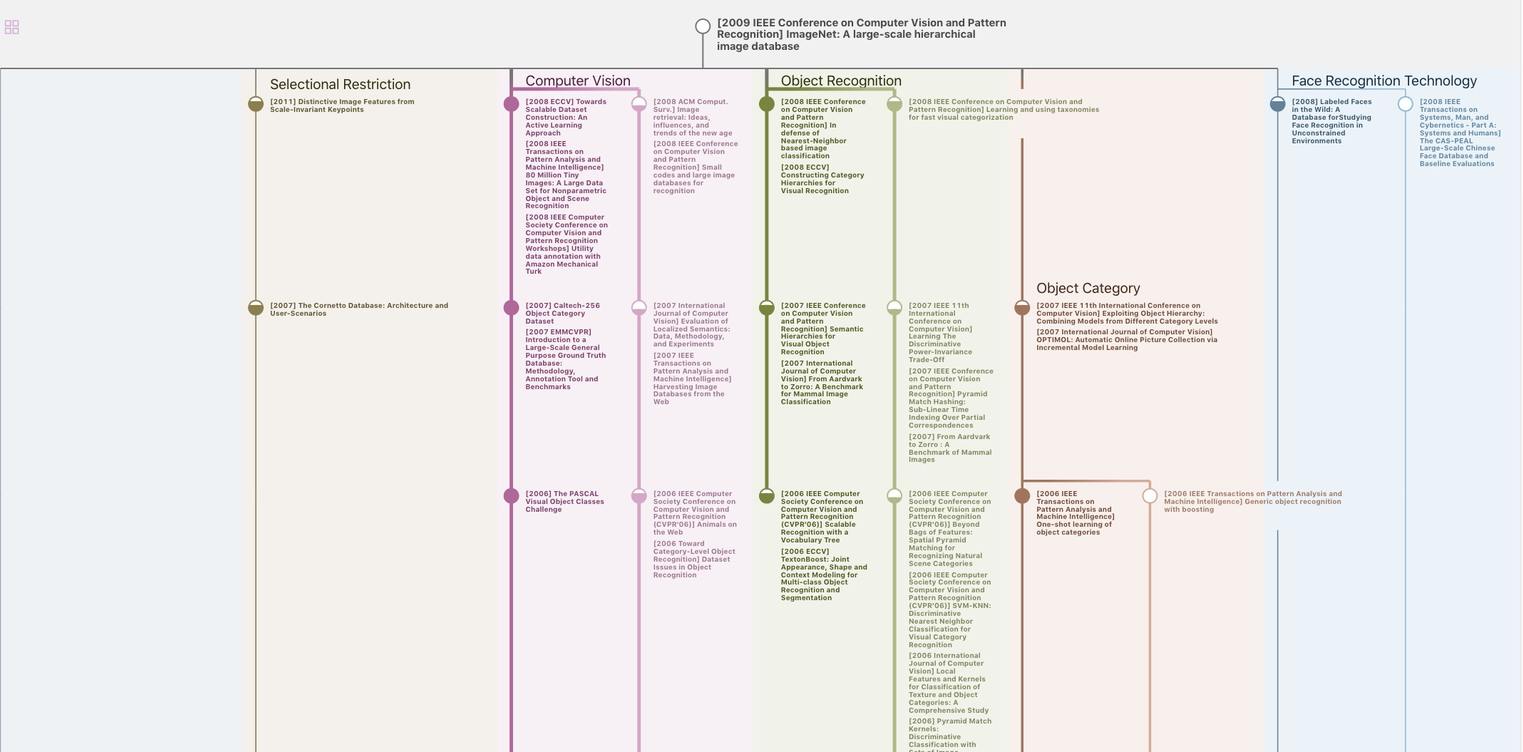
生成溯源树,研究论文发展脉络
Chat Paper
正在生成论文摘要