Establishing an evaluation metric to quantify climate change image realism *
MACHINE LEARNING-SCIENCE AND TECHNOLOGY(2020)
Abstract
With success on controlled tasks, deep generative models are being increasingly applied to humanitarian applications (Nie et al 2017 Int. Conf. on Medical Image Computing and Computer-Assisted Intervention (Berlin: Springer) pp 417-25, Yanardag et al 2017 Deep Empathy). In this paper, we focus on the evaluation of a conditional generative model that illustrates the consequences of climate change-induced flooding to encourage public interest and awareness on the issue. Because metrics for comparing the realism of different modes in a conditional generative model do not exist, we propose several automated and human-based methods for evaluation. To do this, we adapt several existing metrics and assess the automated metrics against gold standard human evaluation. We find that using Frechet Inception Distance with embeddings from an intermediary Inception-v3 layer that precedes the auxiliary classifier produces results most correlated with human realism. While insufficient alone to establish a human-correlated automatic evaluation metric, we believe this work begins to bridge the gap between human and automated generative evaluation procedures, and to generate more realistic images of the future consequences of climate change.
MoreTranslated text
Key words
deep learning,generative adversarial networks,climate change,generative network evaluation,domain transfer
AI Read Science
Must-Reading Tree
Example
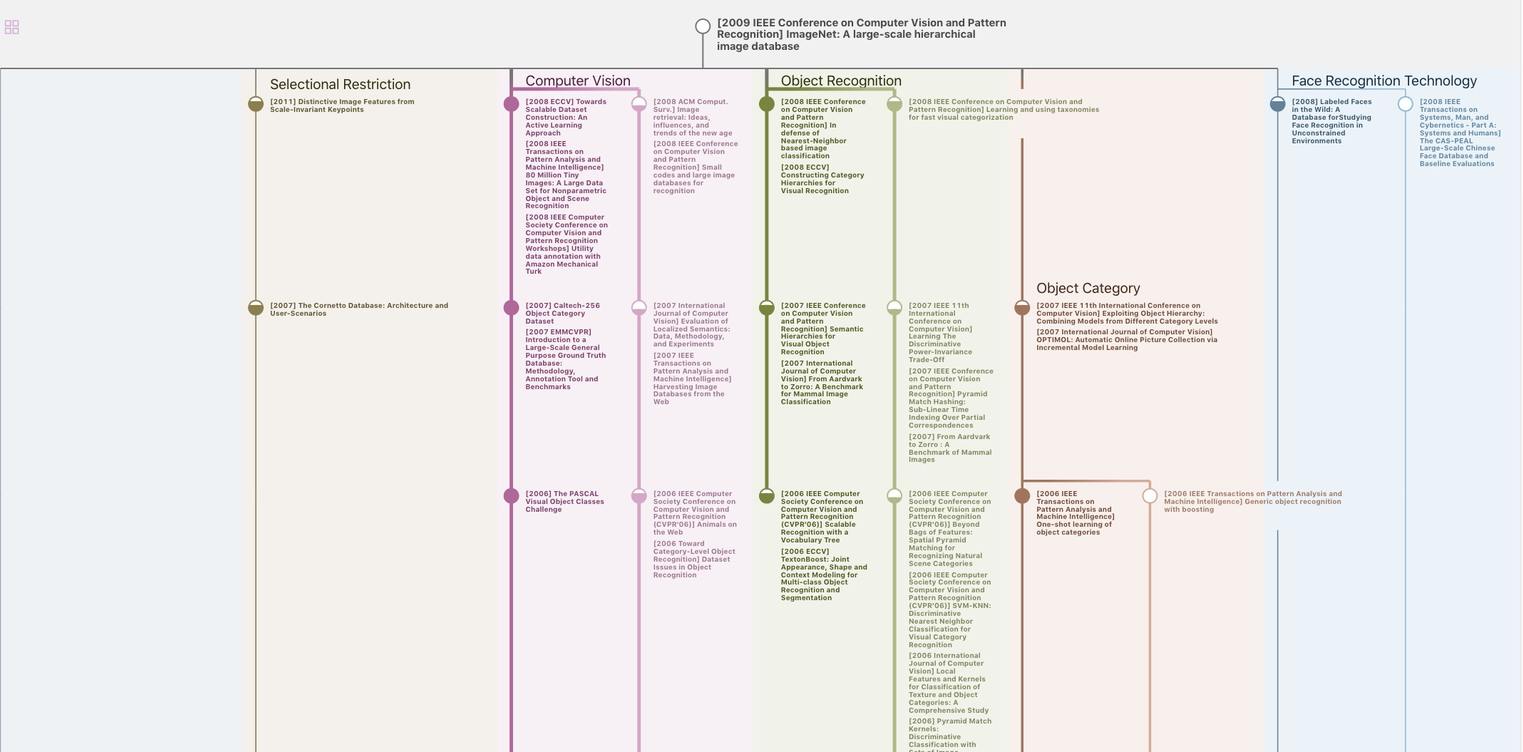
Generate MRT to find the research sequence of this paper
Chat Paper
Summary is being generated by the instructions you defined