Characterizing magnetic reconnection regions using Gaussian mixture models on particle velocity distributions
ASTROPHYSICAL JOURNAL(2019)
摘要
We present a method based on unsupervised machine learning to identify regions of interest using particle velocity distributions as a signature pattern. An automatic density estimation technique is applied to particle distributions provided by PIC simulations to study magnetic reconnection. The key components of the method involve: i) a Gaussian mixture model determining the presence of a given number of subpopulations within an overall population, and ii) a model selection technique with Bayesian Information Criterion to estimate the appropriate number of subpopulations. Thus, this method identifies automatically the presence of complex distributions, such as beams or other non-Maxwellian features, and can be used as a detection algorithm able to identify reconnection regions. The approach is demonstrated for specific double Harris sheet simulations but it can in principle be applied to any other type of simulation and observational data on the particle distribution function.
更多查看译文
关键词
Solar magnetic reconnection,Gaussian mixture model,Plasma astrophysics
AI 理解论文
溯源树
样例
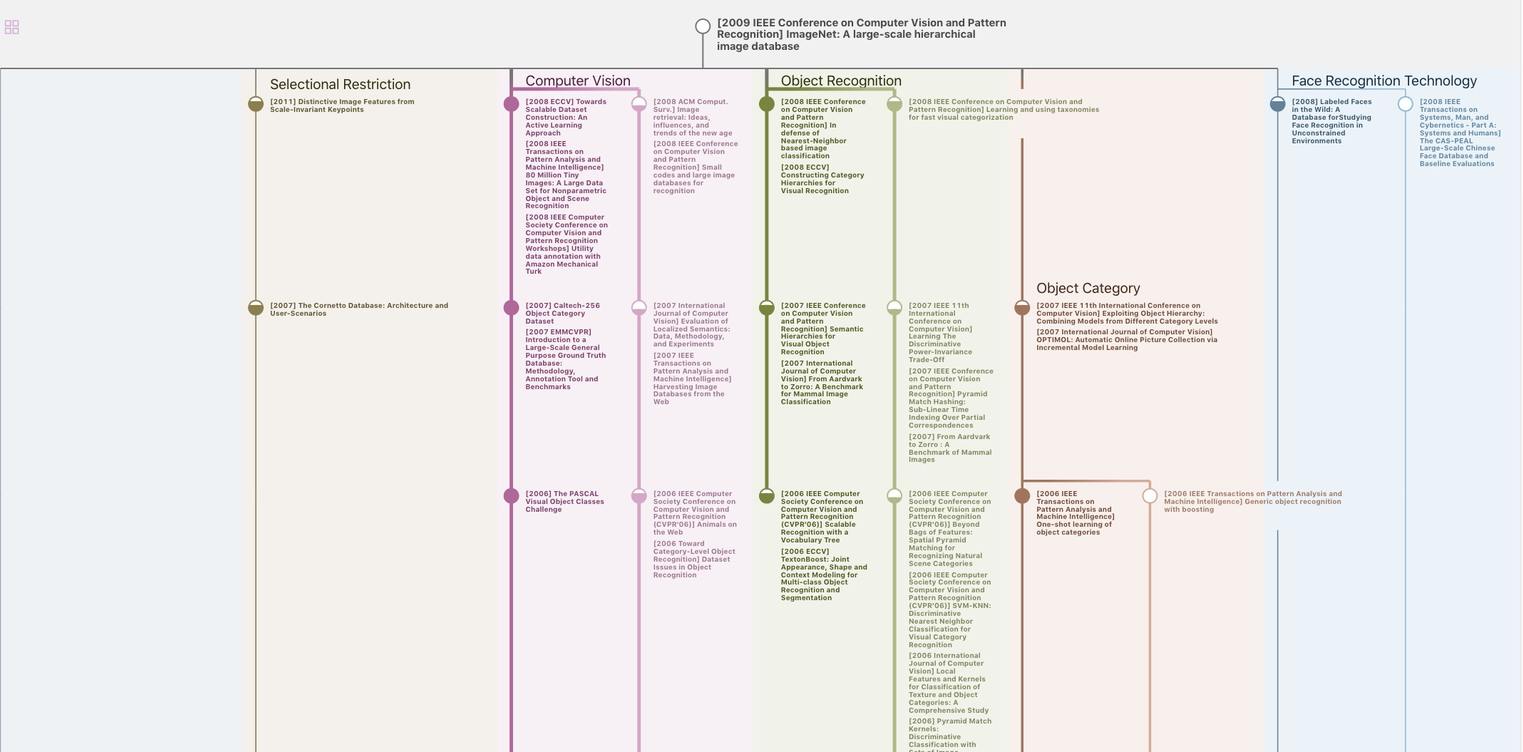
生成溯源树,研究论文发展脉络
Chat Paper
正在生成论文摘要