Scalable Neural Dialogue State Tracking
2019 IEEE AUTOMATIC SPEECH RECOGNITION AND UNDERSTANDING WORKSHOP (ASRU 2019)(2019)
摘要
A Dialogue State Tracker (DST) is a key component in a dialogue system aiming at estimating the beliefs of possible user goals at each dialogue turn. Most of the current DST trackers make use of recurrent neural networks and are based on complex architectures that manage several aspects of a dialogue, including the user utterance, the system actions, and the slot-value pairs defined in a domain ontology. However, the complexity of such neural architectures incurs into a considerable latency in the dialogue state prediction, which limits the deployments of the models in real-world applications, particularly when task scalability (i.e. amount of slots) is a crucial factor. In this paper, we propose an innovative neural model for dialogue state tracking, named Global encoder and Slot-Attentive decoders (G-SAT), which can predict the dialogue state with a very low latency time, while maintaining high-level performance. We report experiments on three different languages (English, Italian, and German) of the WOZ2.0 dataset, and show that the proposed approach provides competitive advantages over state-of-art DST systems, both in terms of accuracy and in terms of time complexity for predictions, being over 15 times faster than the other systems.
更多查看译文
关键词
Dialogue state tracking, deep learning, dialogue systems
AI 理解论文
溯源树
样例
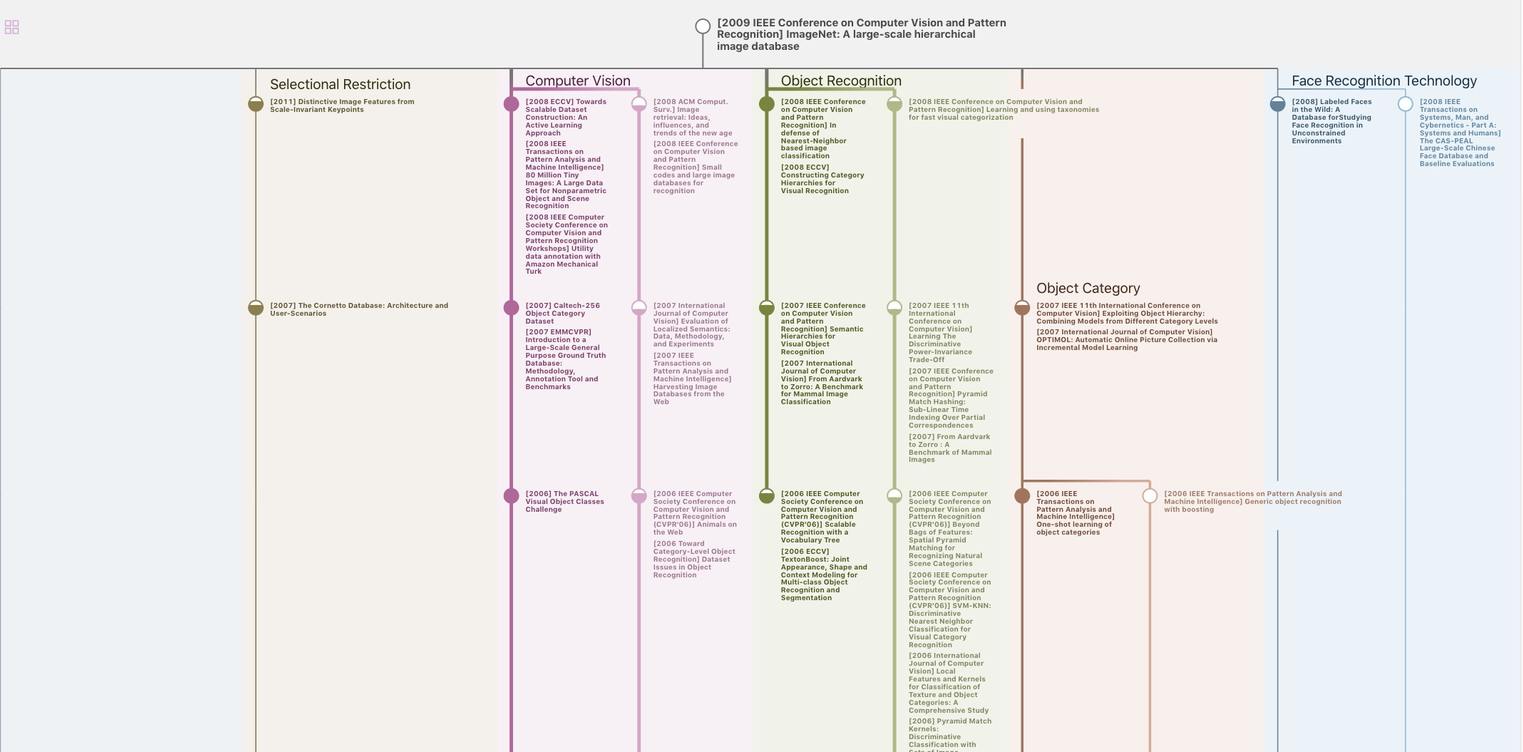
生成溯源树,研究论文发展脉络
Chat Paper
正在生成论文摘要