CRA-Net: Composed Relation Attention Network for Visual Question Answering
Proceedings of the 27th ACM International Conference on Multimedia(2019)
摘要
The task of Visual Question Answering (VQA) is to answer a natural language question tied to the content of a visual image. Most existing VQA models either apply attention mechanism to locate the relevant object regions and/or utilize the off-the-shelf methods of the relation reasoning to detect object relations. However, they 1) mostly encode the simple relations which cannot sufficiently provide sophisticated knowledge for answering complicated visual questions; 2) seldom leverage the harmony cooperation of the object appearance feature and relation feature. To address these problems, we propose a novel end-to-end VQA model, termed Composed Relation Attention Network (CRA-Net ). In specific, we devise two question-adaptive relation attention modules that can extract not only the fine-grained and precise binary relations but also the more sophisticated trinary relations. Both kinds of question-related relations can reveal deeper semantics, thereby enhancing the reasoning ability in question answering. Furthermore, our CRA-Net also combines the object appearance feature with the relation feature under the guidance of the corresponding question, which can reconcile the two types of features effectively. Extensive experiments on two large benchmark datasets, VQA-1.0 and VQA-2.0, demonstrate that our proposed model outperforms state-of-the-art approaches.
更多查看译文
关键词
attention mechanism, relation attention, visual question answering, visual relation
AI 理解论文
溯源树
样例
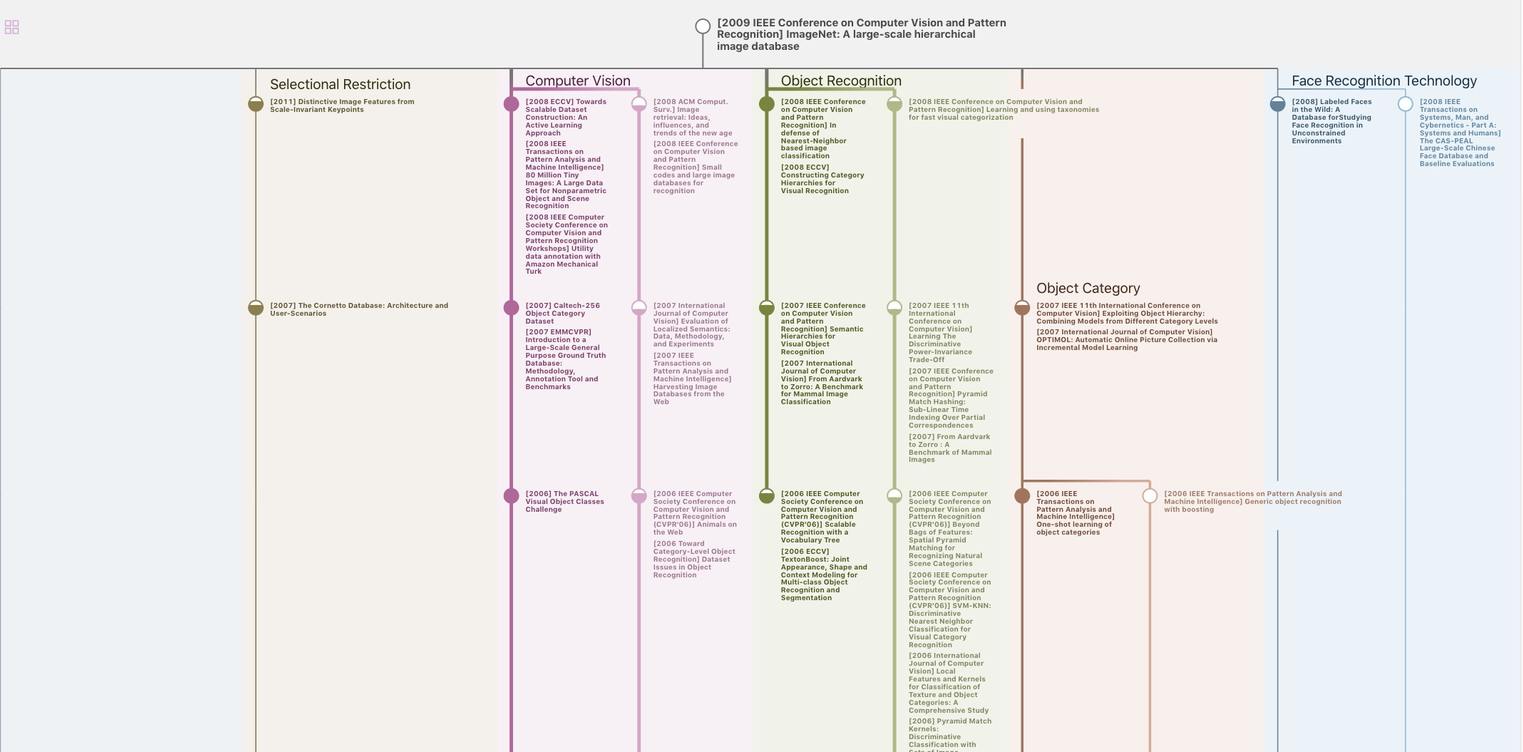
生成溯源树,研究论文发展脉络
Chat Paper
正在生成论文摘要