Using Balanced Training to Minimize Biased Classification
Proceedings of the 5th International Workshop on Historical Document Imaging and Processing(2019)
摘要
In this paper, we classify semantic zone in a document image and observe how a balanced training influences the classification performance. Unlike holistic document which normally distinguishes in content and structural layout, semantic zone introduces stronger inter-class ambiguity as it loses layout feature. Zone extraction from documents often results in unbalanced class distribution. Our experiment shows that training on such data leads to a biased classification. We classify semantic zone by using AlexNet which is a Convolutional Neural Network (CNN). It works on 3 corpora: University of Washington (UW) III, German historical document images (OCRD), and combination of both data sets. Because zone distribution is heavily unbalanced, we augment the data and balance the training distribution to prevent over expression by major classes. To maintain accuracy, we adopt transfer learning from larger document corpus (RVLCDIP). Besides deep learning, we also use heuristic approach to compare performance between balanced and unbalanced training. The result shows that balanced training can alleviate biased performance.
更多查看译文
关键词
balanced training, biased performance, document zone
AI 理解论文
溯源树
样例
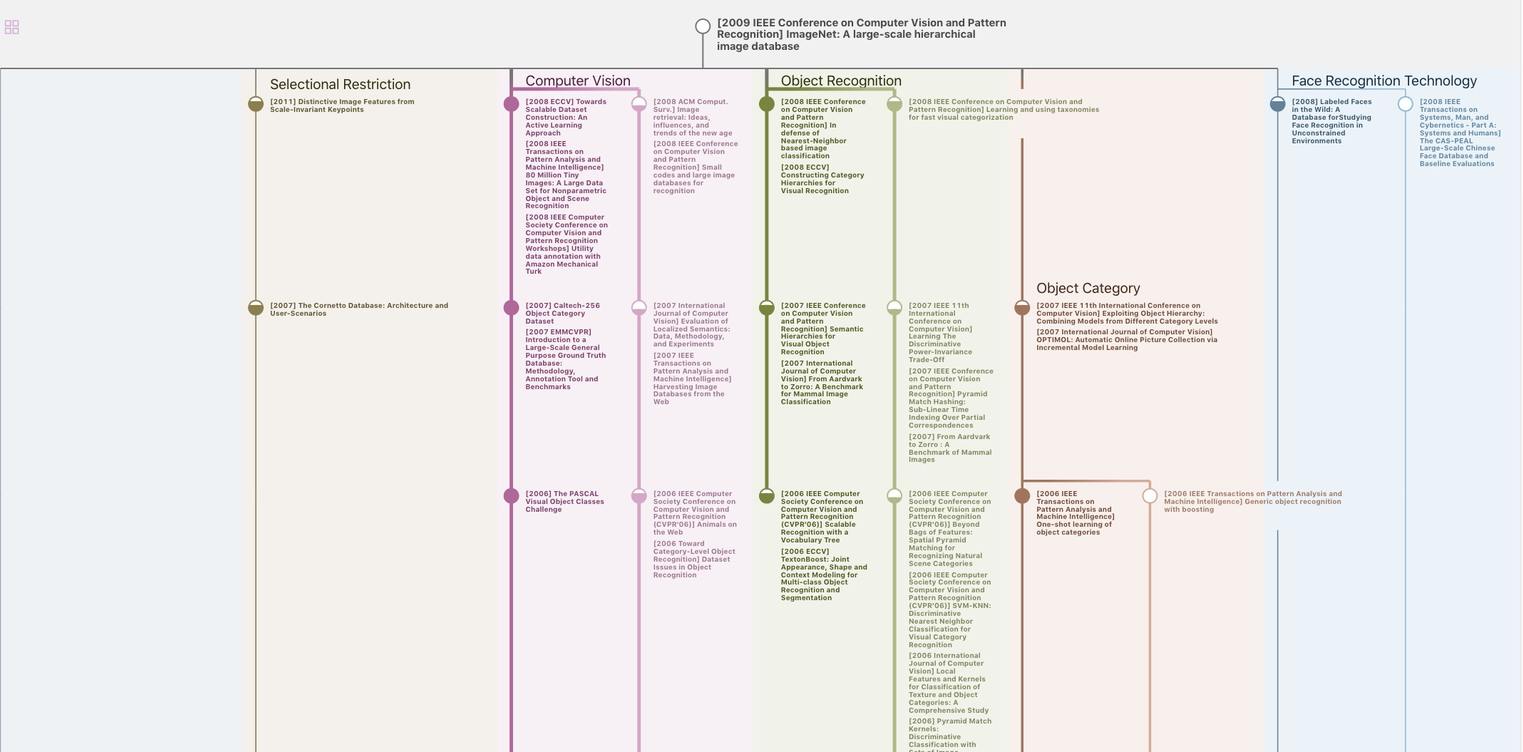
生成溯源树,研究论文发展脉络
Chat Paper
正在生成论文摘要