Non-linear unmixing of hyperspectral images using multiple-kernel self-organising maps
IET Image Processing(2019)
Abstract
The spatial pixel resolution of common multispectral and hyperspectral sensors is generally not sufficient to avoid that multiple elementary materials contribute to the observed spectrum of a single pixel. To alleviate this limitation, spectral unmixing is a by-pass procedure which consists in decomposing the observed spectra associated with these mixed pixels into a set of component spectra, or endmembers, and a set of corresponding proportions, or abundances, that represent the proportion of each endmember in these pixels. In this study, a spectral unmixing technique is proposed to handle the challenging scenario of non-linear mixtures. This algorithm relies on a dedicated implementation of multiple-kernel learning using self-organising map proposed as a solver for the non-linear unmixing problem. Based on a priori knowledge of the endmember spectra, it aims at estimating their relative abundances without specifying the non-linear model under consideration. It is compared to state-of-the-art algorithms using synthetic yet realistic and real hyperspectral images. Results obtained from experiments conducted on synthetic and real hyperspectral images assess the potential and the effectiveness of this unmixing strategy. Finally, the relevance and potential parallel implementation of the proposed method is demonstrated.
MoreTranslated text
Key words
geophysical image processing,hyperspectral imaging,remote sensing,spectral analysis,geophysical signal processing,image processing,self-organising feature maps,learning (artificial intelligence)
AI Read Science
Must-Reading Tree
Example
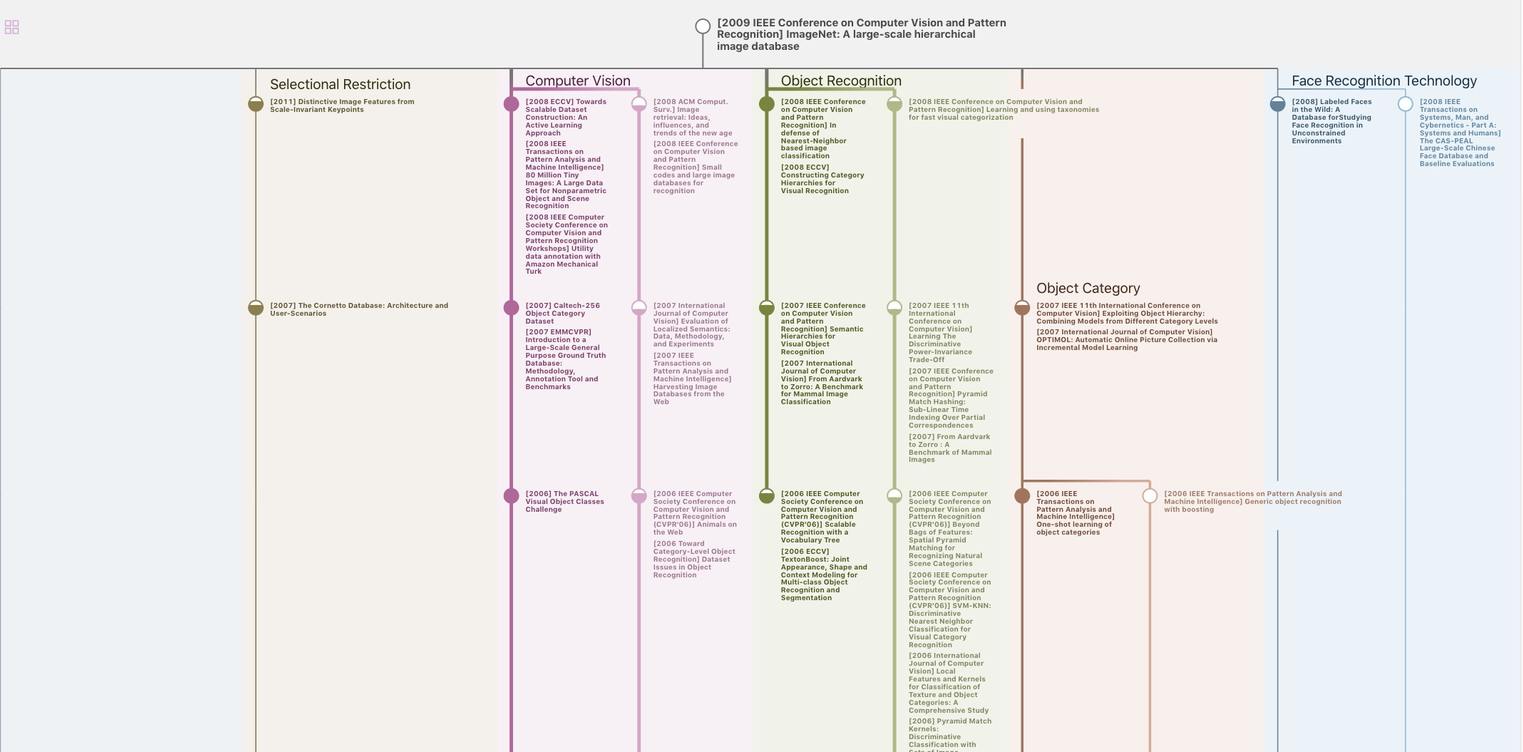
Generate MRT to find the research sequence of this paper
Chat Paper
Summary is being generated by the instructions you defined