Improving A Syntactic Graph Convolution Network For Sentence Compression
CHINESE COMPUTATIONAL LINGUISTICS, CCL 2019(2019)
摘要
Sentence compression is a task of compressing sentences containing redundant information into short semantic expressions, simplifying the text structure and retaining important meanings and information. Neural network-based models are limited by the size of the window and do not perform well when using long-distance dependent information. To solve this problem, we introduce a version of the graph convolutional network (GCNs) to utilize the syntactic dependency relations, and explore a new way to combine GCNs with the Sequence-to-Sequence model (Seq2Seq) to complete the task. The model combines the advantages of both and achieves complementary effects. In addition, in order to reduce the error propagation of the parse tree, we dynamically adjust the dependency arc to optimize the construction process of GCNs. Experiments show that the model combined with the graph convolution network is better than the original model, and the performance in the Google sentence compression dataset has been effectively improved.
更多查看译文
关键词
Sentence compression, Graph convolution network, Sequence-to-Sequence
AI 理解论文
溯源树
样例
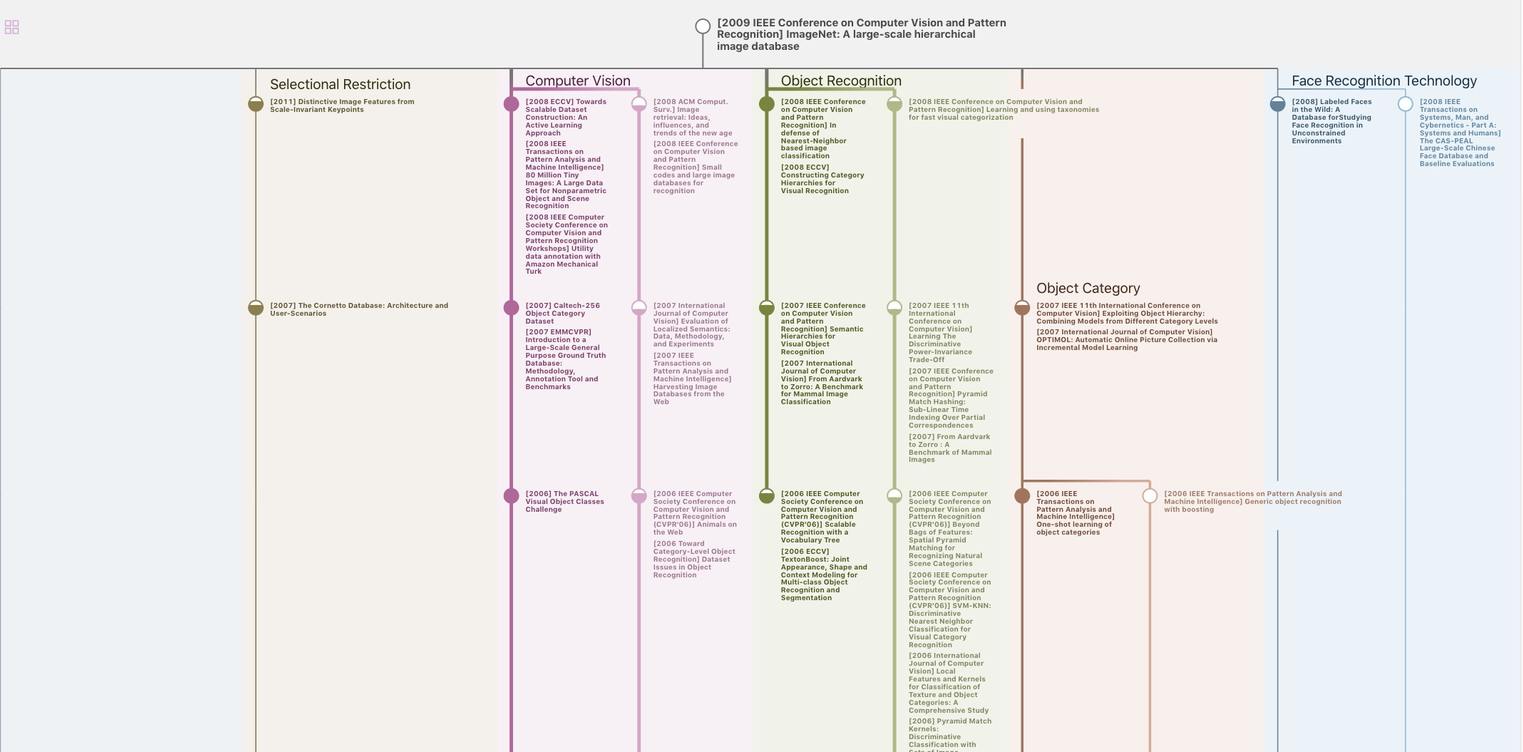
生成溯源树,研究论文发展脉络
Chat Paper
正在生成论文摘要