Neural Ctr Prediction For Native Ad
CHINESE COMPUTATIONAL LINGUISTICS, CCL 2019(2019)
摘要
Native ad is an important kind of online advertising which has similar form with the other content in the same platform. Compared with search ad, predicting the click-through rate (CTR) of native ad is more challenging, since there is no explicit user intent. Learning accurate representations of users and ads that can capture user interests and ad characteristics is critical to this task. Existing methods usually rely on single kind of user behavior for user modeling and ignore the textual information in ads and user behaviors. In this paper, we propose a neural approach for native ad CTR prediction which can incorporate different kinds of user behaviors to model user interests, and can fully exploit the textual information in ads and user behaviors to learn accurate ad and user representations. The core of our approach is an ad encoder and a user encoder. In the ad encoder we learn representations of ads from their titles and descriptions. In the user encoder we propose a mult-view framework to learn representations of users from both their search queries and their browsed webpages by regarding different kinds of behaviors as different views of users. In each view we learn user representations using a hierarchical model and use attention to select important words, search queries and webpages. Experiments on a real-world dataset validate that our approach can effectively improve the performance of native ad CTR prediction.
更多查看译文
关键词
Native ad, User modeling, CTR prediction
AI 理解论文
溯源树
样例
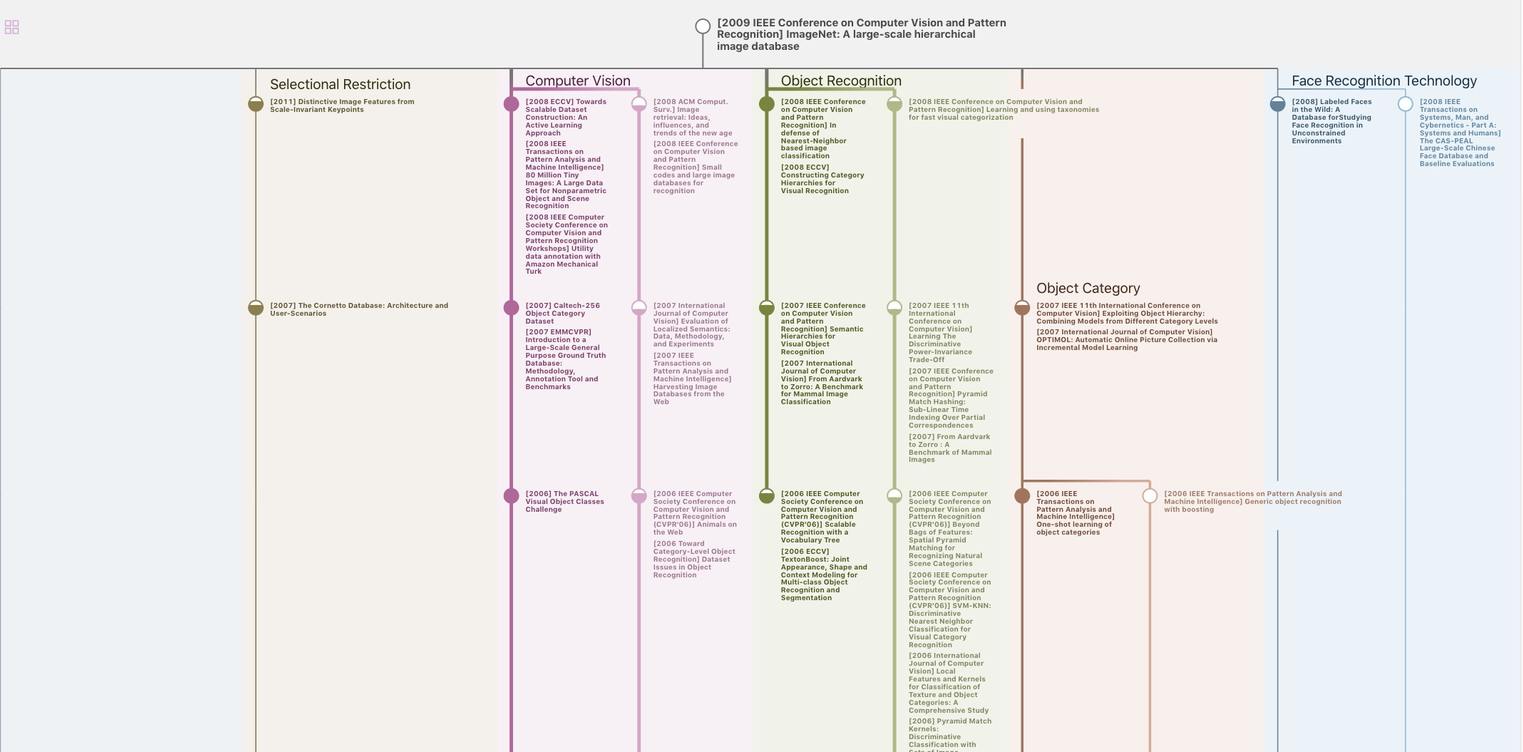
生成溯源树,研究论文发展脉络
Chat Paper
正在生成论文摘要