Deep clustering with concrete k-means
2020 IEEE INTERNATIONAL CONFERENCE ON ACOUSTICS, SPEECH, AND SIGNAL PROCESSING(2019)
摘要
We address the problem of simultaneously learning a k-means clustering and deep feature representation from unlabelled data, which is of interest due to the potential of deep k-means to outperform traditional two-step feature extraction and shallow-clustering strategies. We achieve this by developing a gradient-estimator for the non-differentiable k-means objective via the Gumbel-Softmax reparameterisation trick. In contrast to previous attempts at deep clustering, our concrete k-means model can be optimised with respect to the canonical k-means objective and is easily trained end-to-end without resorting to alternating optimisation. We demonstrate the efficacy of our method on standard clustering benchmarks.
更多查看译文
关键词
Deep Clustering, Unsupervised Learning, Gradient Estimation
AI 理解论文
溯源树
样例
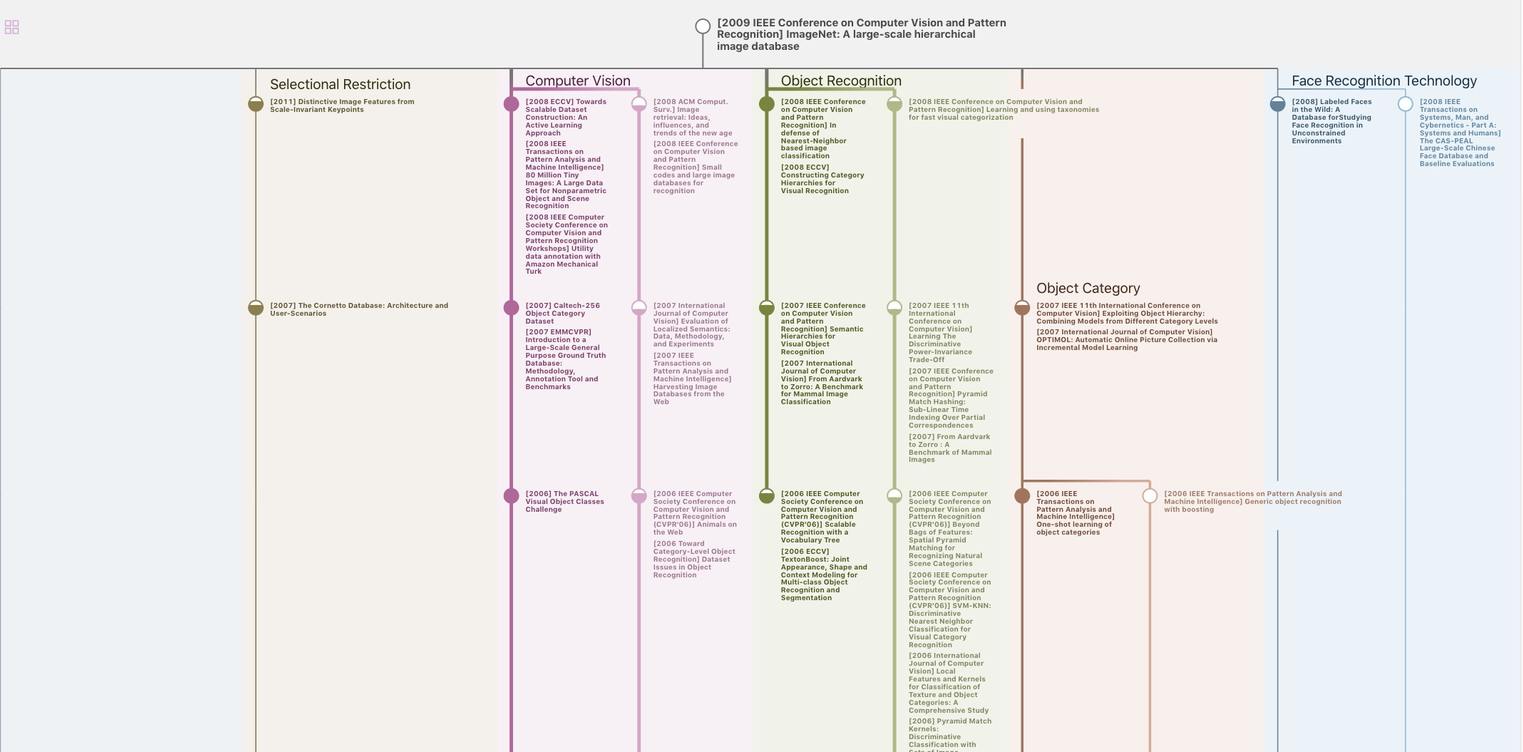
生成溯源树,研究论文发展脉络
Chat Paper
正在生成论文摘要