Towards a Precipitation Bias Corrector against Noise and Maldistribution
arxiv(2019)
摘要
With broad applications in various public services like aviation management and urban disaster warning, numerical precipitation prediction plays a crucial role in weather forecast. However, constrained by the limitation of observation and conventional meteorological models, the numerical precipitation predictions are often highly biased. To correct this bias, classical correction methods heavily depend on profound experts who have knowledge in aerodynamics, thermodynamics and meteorology. As precipitation can be influenced by countless factors, however, the performances of these expert-driven methods can drop drastically when some un-modeled factors change. To address this issue, this paper presents a data-driven deep learning model which mainly includes two blocks, i.e. a Denoising Autoencoder Block and an Ordinal Regression Block. To the best of our knowledge, it is the first expert-free models for bias correction. The proposed model can effectively correct the numerical precipitation prediction based on 37 basic meteorological data from European Centre for Medium-Range Weather Forecasts (ECMWF). Experiments indicate that compared with several classical machine learning algorithms and deep learning models, our method achieves the best correcting performance and meteorological index, namely the threat scores (TS), obtaining satisfactory visualization effect.
更多查看译文
关键词
precipitation bias corrector,noise,maldistribution
AI 理解论文
溯源树
样例
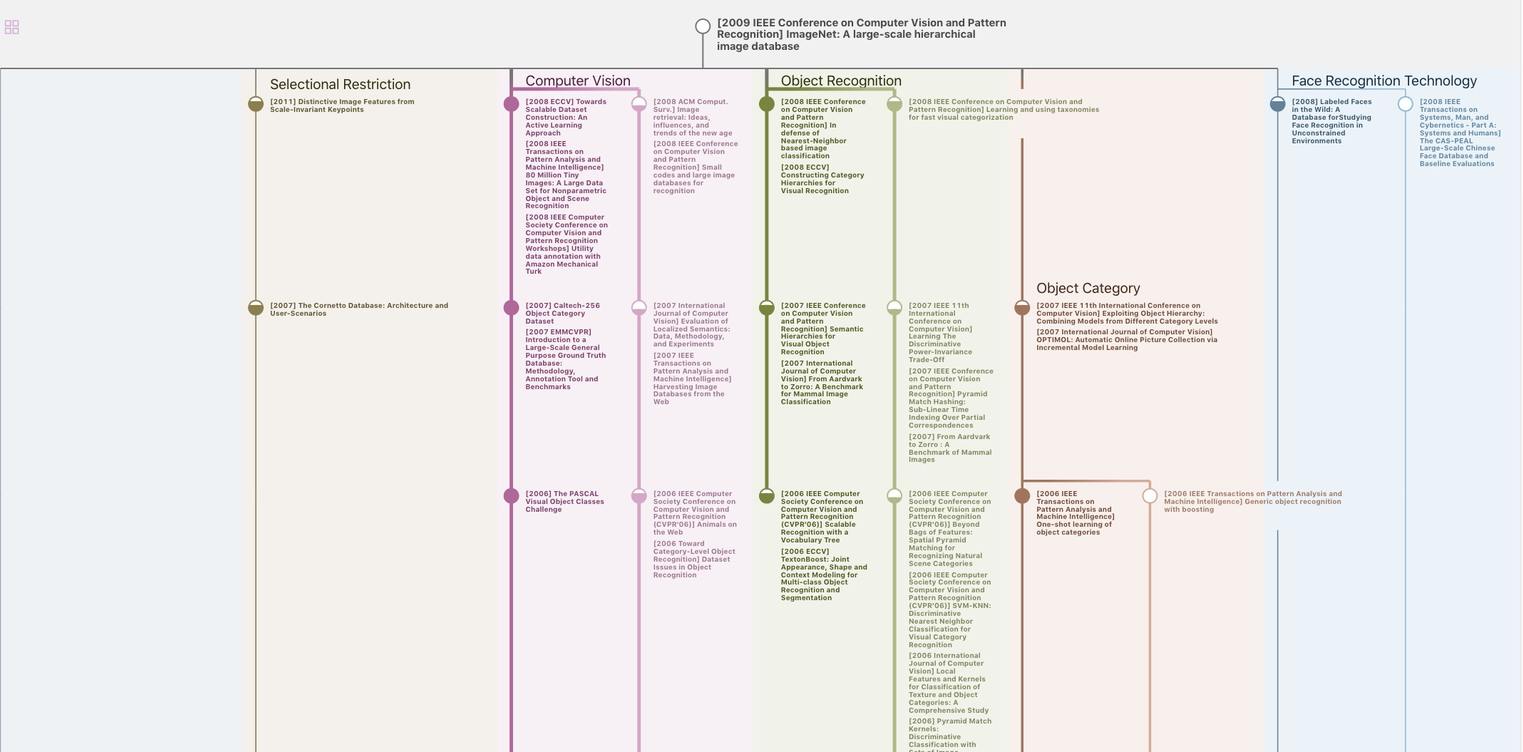
生成溯源树,研究论文发展脉络
Chat Paper
正在生成论文摘要