Global Saliency: Aggregating Saliency Maps to Assess Dataset Artefact Bias
CoRR(2019)
Abstract
In high-stakes applications of machine learning models, interpretability methods provide guarantees that models are right for the right reasons. In medical imaging, saliency maps have become the standard tool for determining whether a neural model has learned relevant robust features, rather than artefactual noise. However, saliency maps are limited to local model explanation because they interpret predictions on an image-by-image basis. We propose aggregating saliency globally, using semantic segmentation masks, to provide quantitative measures of model bias across a dataset. To evaluate global saliency methods, we propose two metrics for quantifying the validity of saliency explanations. We apply the global saliency method to skin lesion diagnosis to determine the effect of artefacts, such as ink, on model bias.
MoreTranslated text
AI Read Science
Must-Reading Tree
Example
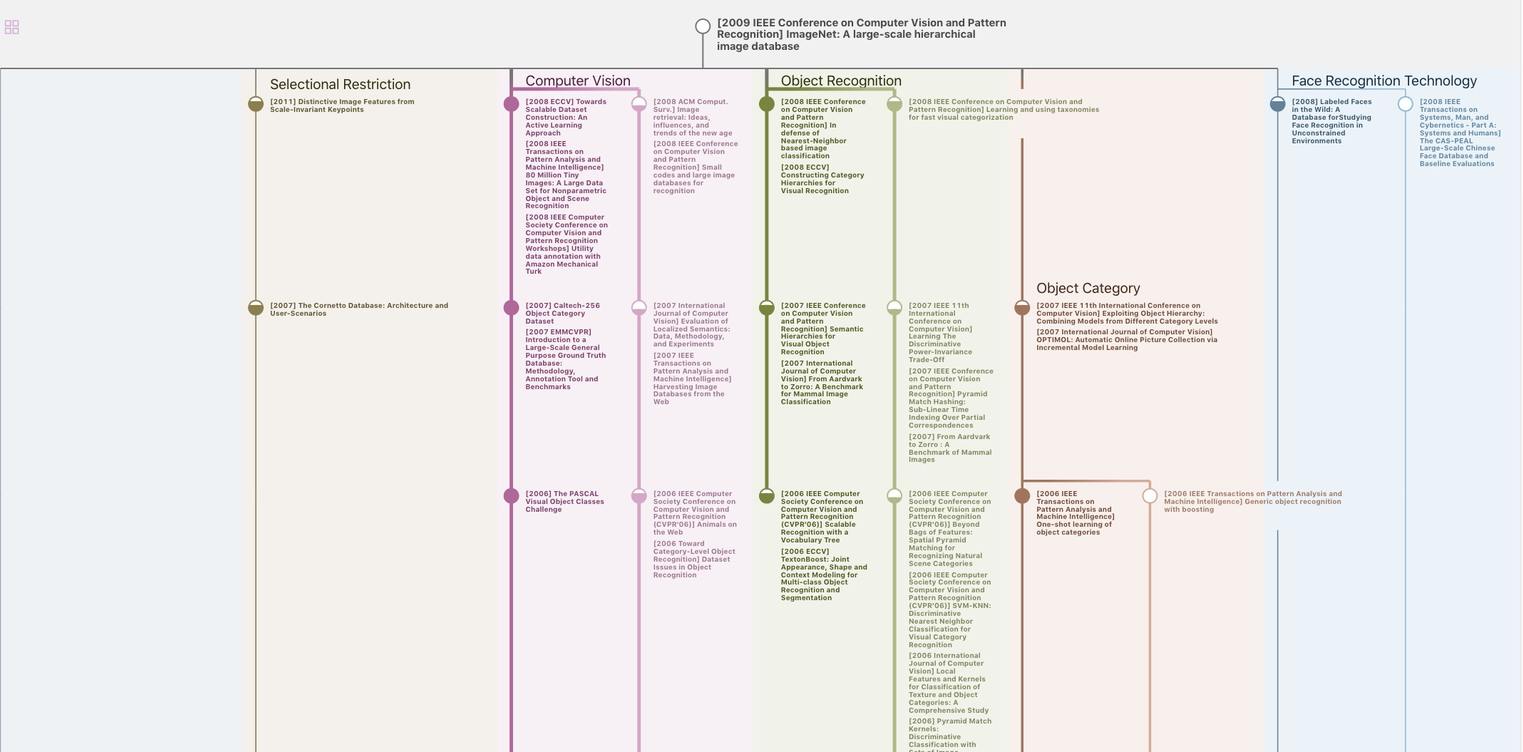
Generate MRT to find the research sequence of this paper
Chat Paper
Summary is being generated by the instructions you defined