Weighted Sparse Representation For Classification Of Motor Imagery Eeg Signals
2019 41ST ANNUAL INTERNATIONAL CONFERENCE OF THE IEEE ENGINEERING IN MEDICINE AND BIOLOGY SOCIETY (EMBC)(2019)
Abstract
Motor imagery (MI) based brain-computer interface systems (BCIs) are highly in demand for many real-time applications such as hands and touch-free text entry, prosthetic arms, virtual reality, movement of wheelchairs, etc. Traditional sparse representation based classification (SRC) is a thriving technique in recent years and has been a successful approach for classifying MI EEG signals. To further improve the capability of SRC, in this paper, a weighted SRC (WSRC) has been proposed for classifying two-class MI tasks (right-hand, right-foot). WSRC constructs a weighted dictionary according to the dissimilarity information between the test data and the training samples. Then for the given test data the sparse coefficients are computed over the weighted dictionary using l(0)-minimization problem. The sparse solution obtained using WSRC gives better discriminative information than SRC and as a consequence, WSRC proves to be superior for MI EEG classification. The experimental results substantiate that WSRC is more efficient and accurate than SRC.
MoreTranslated text
Key words
Electroencephalography, motor imagery, sparsity-based classification, weighted sparse representation
AI Read Science
Must-Reading Tree
Example
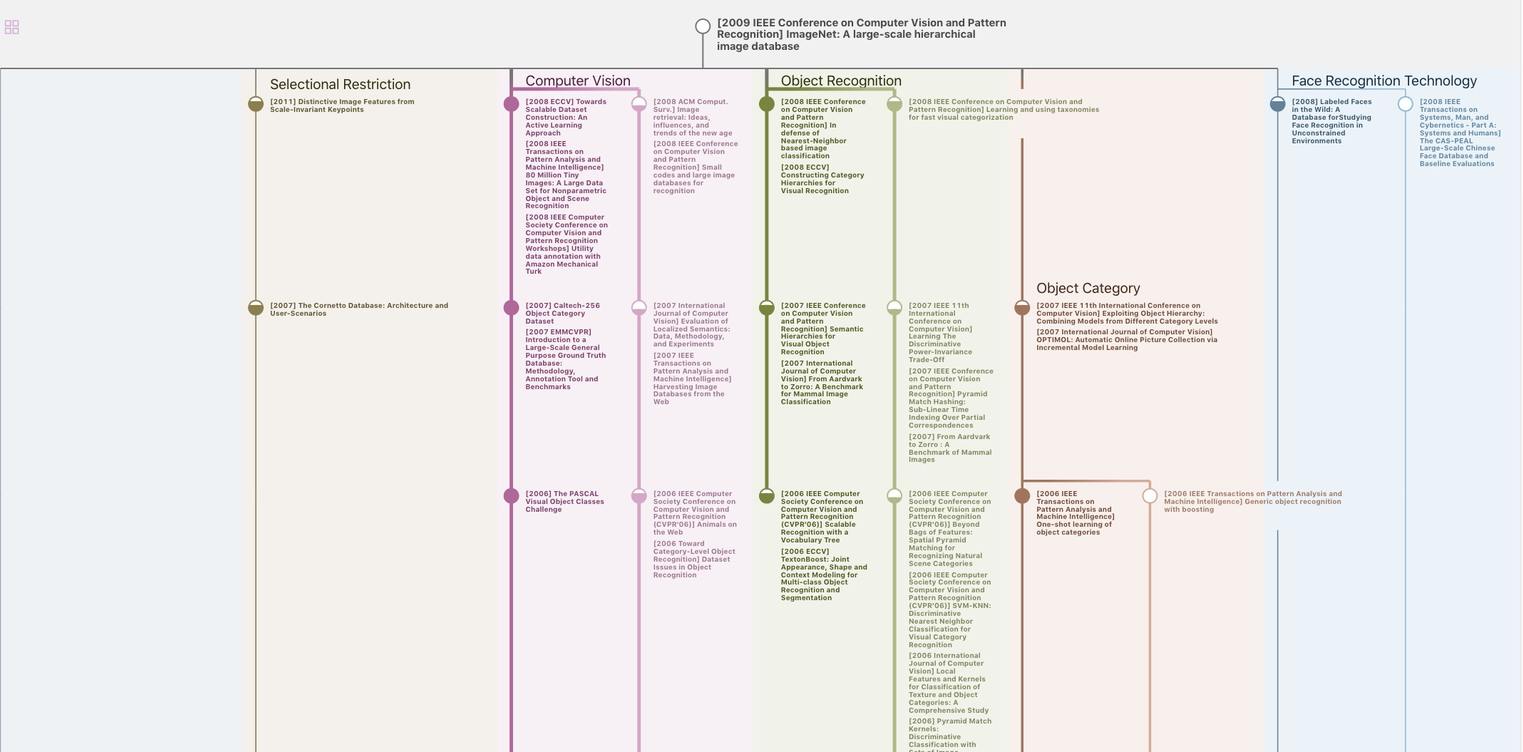
Generate MRT to find the research sequence of this paper
Chat Paper
Summary is being generated by the instructions you defined