Machine Learning For Classification Of Uterine Activity Outside Pregnancy
2019 41ST ANNUAL INTERNATIONAL CONFERENCE OF THE IEEE ENGINEERING IN MEDICINE AND BIOLOGY SOCIETY (EMBC)(2019)
Abstract
The objective of this study was to investigate the use of classification methods by a machine-learning approach for discriminating the uterine activity during the four phases of the menstrual cycle. Four different classifiers, including support vector machine (SVM), K-nearest neighbors (KNN), Gaussian mixture model (GMM) and naive Bayes are here proposed. A set of amplitude- and frequency-features were extracted from signals measured by two different quantitative and non-invasive methods, such as electrohysterography and ultrasound speckle tracking. The proposed classifiers were trained using all possible feature combinations. The method was applied on a database (24 measurements) collected in different phases of the menstrual cycle, comprising uterine active and quiescent phases. The SVM classifier showed the best performance for discrimination between the different menstrual phases. The classification accuracy, sensitivity, and specificity were 90%, 79%, 93%, respectively. Similar methods can in the future contribute to the diagnosis of infertility or other common uterine diseases such as endometriosis.
MoreTranslated text
Key words
Algorithms,Bayes Theorem,Female,Humans,Machine Learning,Menstrual Cycle,Normal Distribution,Support Vector Machine,Uterus
AI Read Science
Must-Reading Tree
Example
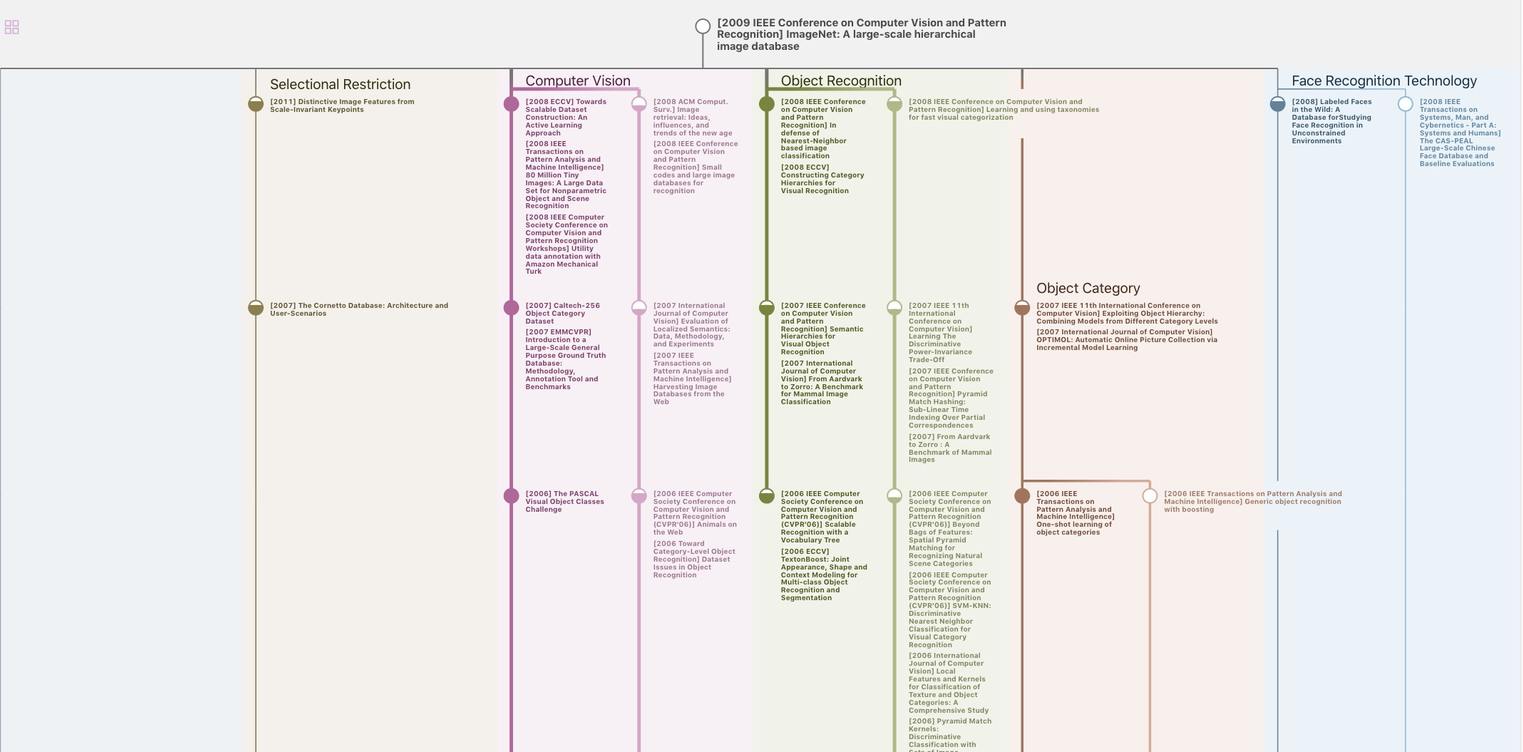
Generate MRT to find the research sequence of this paper
Chat Paper
Summary is being generated by the instructions you defined