DP-CGAN: Differentially Private Synthetic Data and Label Generation
2019 IEEE/CVF Conference on Computer Vision and Pattern Recognition Workshops (CVPRW)(2020)
摘要
Generative Adversarial Networks (GANs) are one of the well-known models to generate synthetic data including images, especially for research communities that cannot use original sensitive datasets because they are not publicly accessible. One of the main challenges in this area is to preserve the privacy of individuals who participate in the training of the GAN models. To address this challenge, we introduce a Differentially Private Conditional GAN (DP-CGAN) training framework based on a new clipping and perturbation strategy, which improves the performance of the model while preserving privacy of the training dataset. DP-CGAN generates both synthetic data and corresponding labels and leverages the recently introduced Renyi differential privacy accountant to track the spent privacy budget. The experimental results show that DP-CGAN can generate visually and empirically promising results on the MNIST dataset with a single-digit epsilon parameter in differential privacy.
更多查看译文
关键词
single-digit epsilon parameter,MNIST dataset,differentially private conditional GAN training framework,Renyi differential privacy accountant,training dataset,GAN models,original sensitive datasets,research communities,generative adversarial networks,label generation,differentially private synthetic data,DP-CGAN,spent privacy budget
AI 理解论文
溯源树
样例
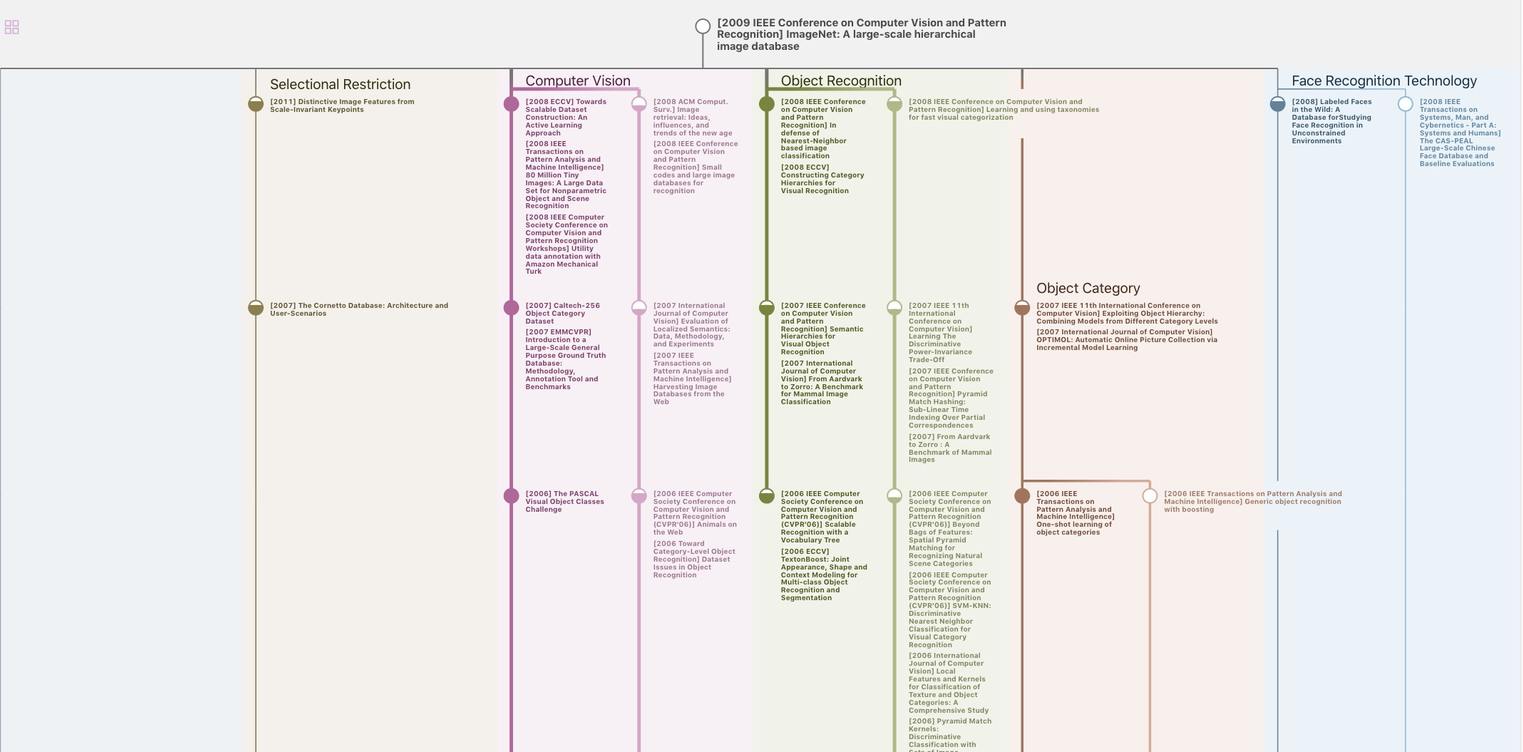
生成溯源树,研究论文发展脉络
Chat Paper
正在生成论文摘要