Three-Stream Convolutional Neural Network With Multi-Task and Ensemble Learning for 3D Action Recognition
IEEE Computer Society Conference on Computer Vision and Pattern Recognition Workshops(2019)
摘要
In this paper, we propose a three-stream convolutional neural network (3SCNN) for action recognition from skeleton sequences, which aims to thoroughly and fully exploit the skeleton data by extracting, learning, fusing and inferring multiple motion-related features, including 3D joint positions and joint displacements across adjacent frames as well as oriented bone segments. The proposed 3SCNN involves three sequential stages. The first stage enriches three independently extracted features by co-occurrence feature learning. The second stage involves multi-channel pairwise fusion to take advantage of the complementary and diverse nature among three features. The third stage is a multi-task and ensemble learning network to further improve the generalization ability of 3SCNN. Experimental results on the standard dataset show the effectiveness of our proposed multi-stream feature learning, fusion and inference method for skeleton-based 3D action recognition.
更多查看译文
关键词
motion-related feature extraction,3D joint positions,joint displacements,oriented bone segments,co-occurrence feature learning,multichannel pairwise fusion,ensemble learning network,multistream feature learning,three-stream convolutional neural network,skeleton data,multitask learning,skeleton-based 3D action recognition,3SCNN generalization ability,multiple motion-related feature fusion,multiple motion-related feature learning
AI 理解论文
溯源树
样例
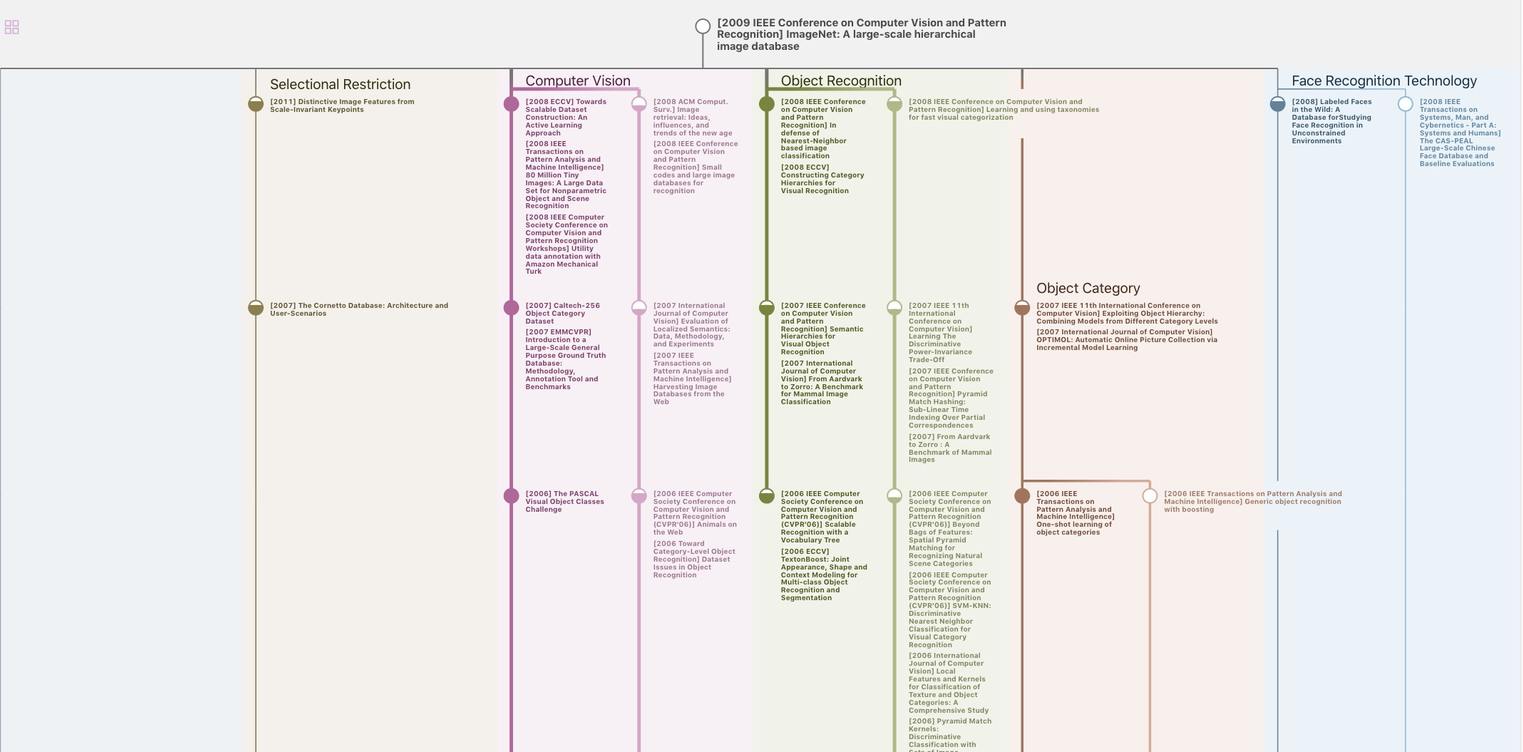
生成溯源树,研究论文发展脉络
Chat Paper
正在生成论文摘要