Towards Rich Feature Discovery With Class Activation Maps Augmentation For Person Re-Identification
2019 IEEE/CVF CONFERENCE ON COMPUTER VISION AND PATTERN RECOGNITION (CVPR 2019)(2019)
摘要
The fundamental challenge of small inter-person variation requires Person Re-Identification (Re-ID) models to capture sufficient fine-grained features. This paper proposes to discover diverse discriminative visual cues without extra assistance, e.g., pose estimation, human parsing. Specifically, a Class Activation Maps (CAM) augmentation model is proposed to expand the activation scope of baseline Re-ID model to explore rich visual cues, where the backbone network is extended by a series of ordered branches which share the same input but output complementary CAM. A novel Overlapped Activation Penalty is proposed to force the current branch to pay more attention to the image regions less activated by the previous ones, such that spatial diverse visual features can be discovered. The proposed model achieves state-of-the-art results on three Re-ID datasets. Moreover, a visualization approach termed ranking activation map (RAM) is proposed to explicitly interpret the ranking results in the test stage, which gives qualitative validations of the proposed method.
更多查看译文
关键词
Biometrics,Recognition: Detection,Categorization,Retrieval,Representation Learning
AI 理解论文
溯源树
样例
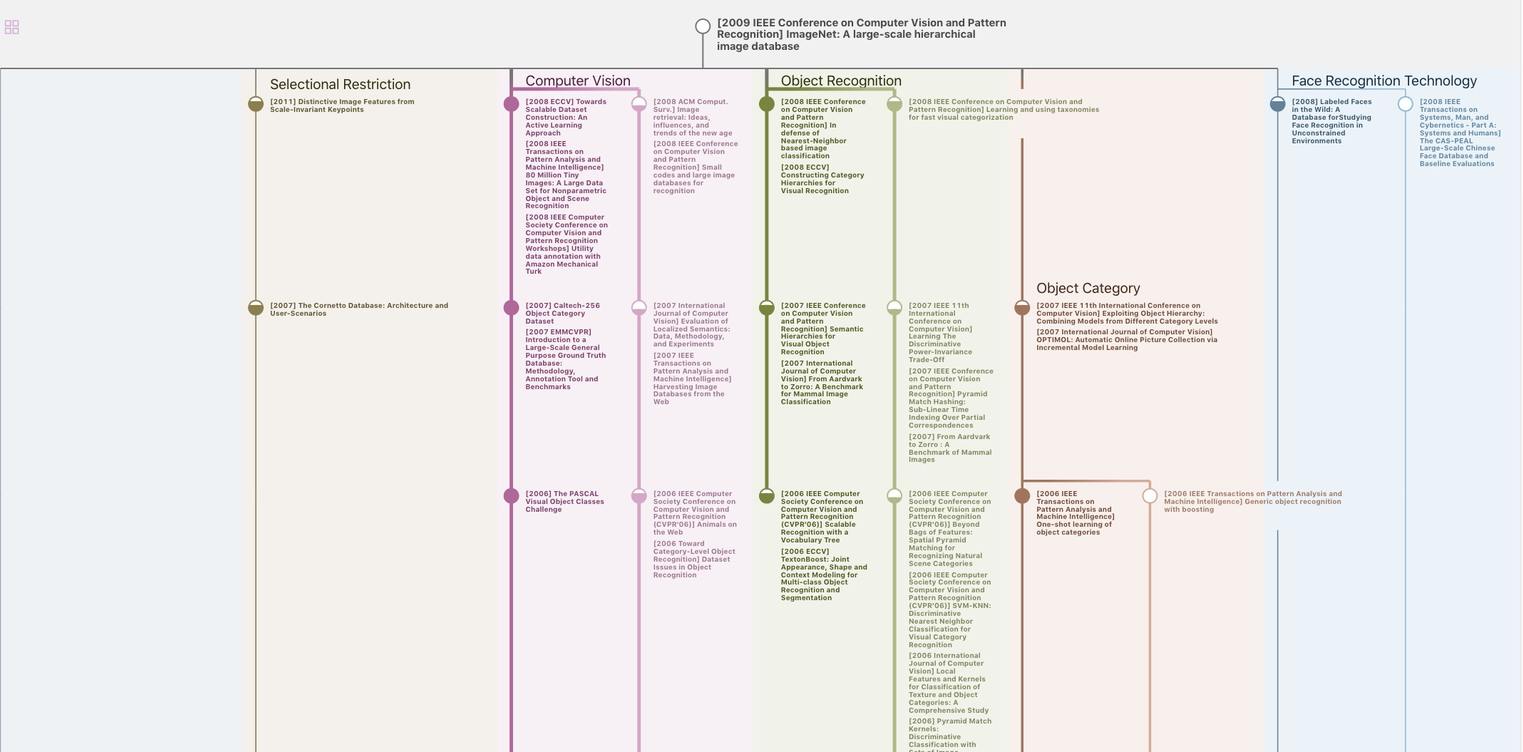
生成溯源树,研究论文发展脉络
Chat Paper
正在生成论文摘要