Maxpoolnms: Getting Rid Of Nms Bottlenecks In Two-Stage Object Detectors
2019 IEEE/CVF CONFERENCE ON COMPUTER VISION AND PATTERN RECOGNITION (CVPR 2019)(2019)
摘要
Modern convolutional object detectors have improved the detection accuracy significantly, which in turn inspired the development of dedicated hardware accelerators to achieve real-time performance by exploiting inherent parallelism in the algorithm. Non-maximum suppression (NMS) is an indispensable operation in object detection. In stark contrast to most operations, the commonly-adopted GreedyNMS algorithm does not foster parallelism, which can be a major performance bottleneck. In this paper, we introduce MaxpoolNMS, a parallelizable alternative to the NMS algorithm, which is based on max-pooling classification score maps. By employing a novel multi-scale multi-channel max-pooling strategy, our method is 20 x faster than GreedyNMS while simultaneously achieves comparable accuracy, when quantified across various benchmarking datasets, i.e., MS COCO, KI17I and PASCAL VOC. Furthermore, our method is better suited for hardware-based acceleration than GreedyNMS.
更多查看译文
关键词
Recognition: Detection,Categorization,Retrieval,Robotics + Driving, Vision Applications and Systems
AI 理解论文
溯源树
样例
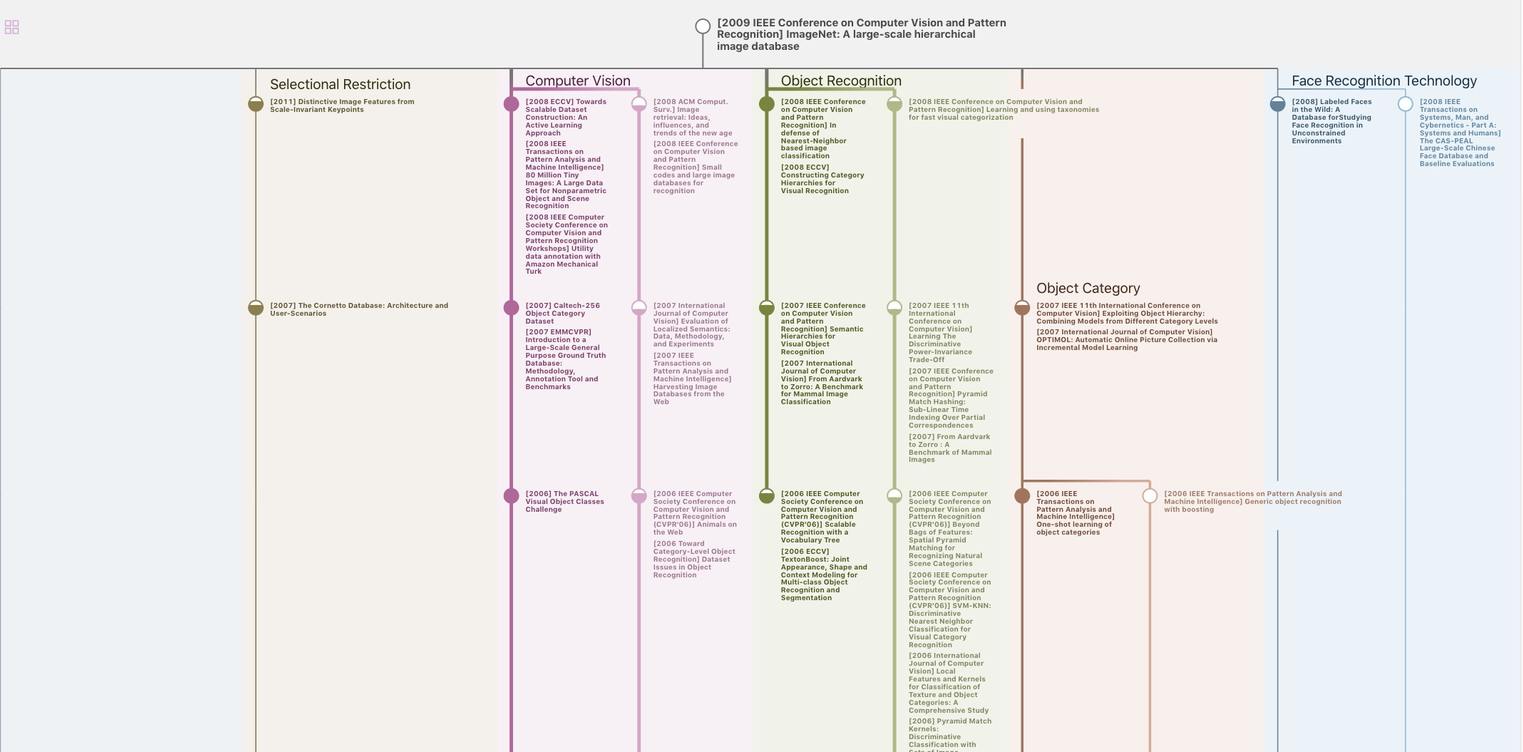
生成溯源树,研究论文发展脉络
Chat Paper
正在生成论文摘要