Machine Learning Models Improve the Diagnostic Yield of Peripheral Blood Flow Cytometry
AMERICAN JOURNAL OF CLINICAL PATHOLOGY(2019)
摘要
Objectives: Peripheral blood flow cytometry (PBFC) is useful for evaluating circulating hematologic malignancies (HM) but has limited diagnostic value for screening. We used machine learning to evaluate whether clinical history and CBC/differential parameters could improve PBFC utilization. Methods: PBFC cases with concurrent/recent CBC/differential were split into training (n = 626) and test (n = 159) cohorts. We classified PBFC results with abnormal blast/lymphoid populations as positive and used two models to predict results. Results: Positive PBFC results were seen in 58% and 21% of training cases with and without prior HM (P < .001). % neutrophils, absolute lymphocyte count, and % blasts/other cells differed significantly between positive and negative PBFC groups (areas under the curve [AUC] > 0.7). Among test cases, a decision tree model achieved 98% sensitivity and 65% specificity (AUC = 0.906). A logistic regression model achieved 100% sensitivity and 54% specificity (AUC = 0.919). Conclusions: We outline machine learning-based triaging strategies to decrease unnecessary utilization of PBFC by 35% to 40%.
更多查看译文
关键词
Peripheral blood,Flow cytometry,Machine learning,Test utilization,Hematologic malignancy,Decision tree,Triage algorithm,Clinical decision support
AI 理解论文
溯源树
样例
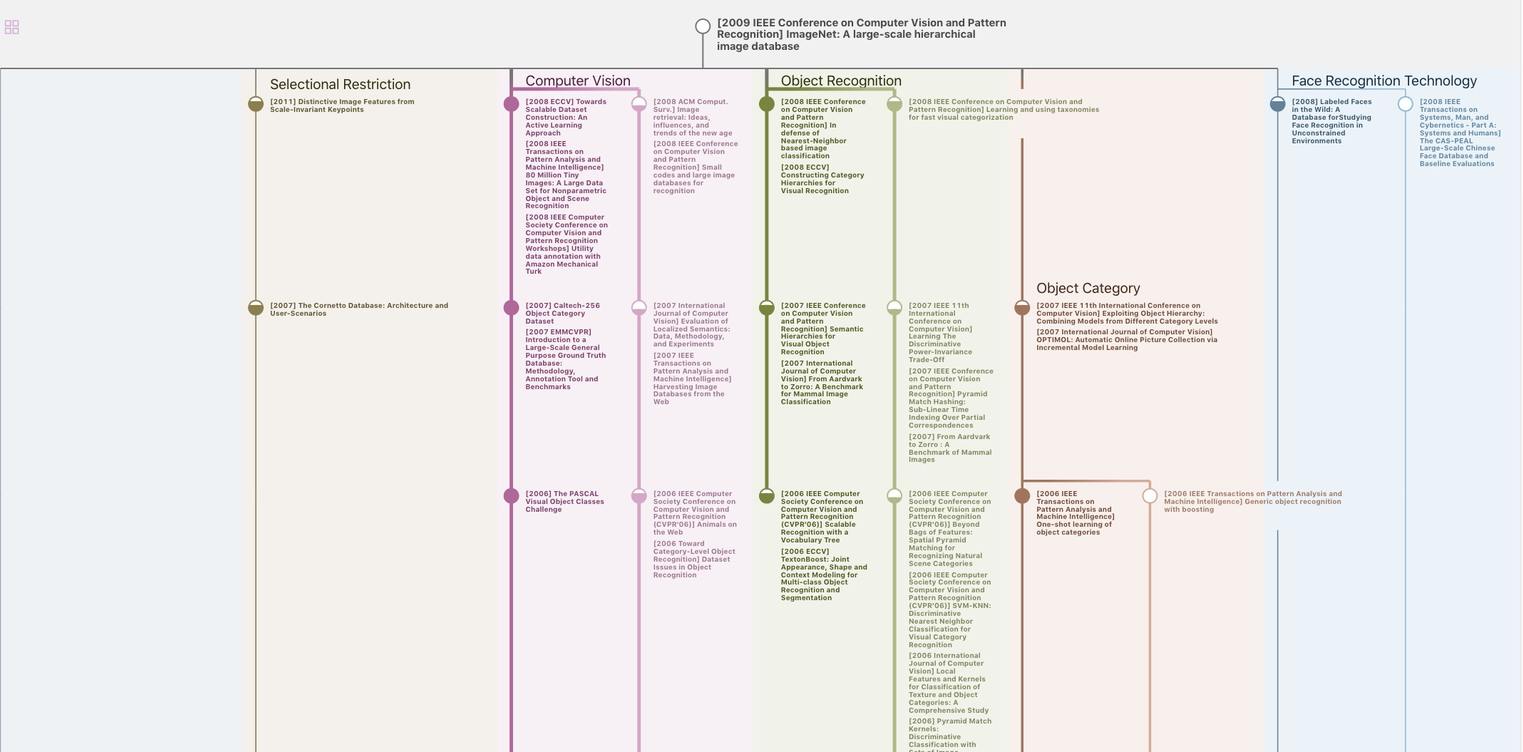
生成溯源树,研究论文发展脉络
Chat Paper
正在生成论文摘要