Uncertainty Quantification for Space Situational Awareness and Traffic Management.
SENSORS(2019)
摘要
This paper presents a sensor-orientated approach to on-orbit position uncertainty generation and quantification for both ground-based and space-based surveillance applications. A mathematical framework based on the least squares formulation is developed to exploit real-time navigation measurements and tracking observables to provide a sound methodology that supports separation assurance and collision avoidance among Resident Space Objects (RSO). In line with the envisioned Space Situational Awareness (SSA) evolutions, the method aims to represent the navigation and tracking errors in the form of an uncertainty volume that accurately depicts the size, shape, and orientation. Simulation case studies are then conducted to verify under which sensors performance the method meets Gaussian assumptions, with a greater view to the implications that uncertainty has on the cyber-physical architecture evolutions and Cognitive Human-Machine Systems required for Space Situational Awareness and the development of a comprehensive Space Traffic Management framework.
更多查看译文
关键词
Space Traffic Management,Cyber-Physical Systems,Resident Space Object,Space-Based Surveillance,Radar Performance,Gauss-Helmert Method,Space Situational Awareness,Uncertainty Quantification,Covariance Realism,Cognitive Human-Machine Interaction
AI 理解论文
溯源树
样例
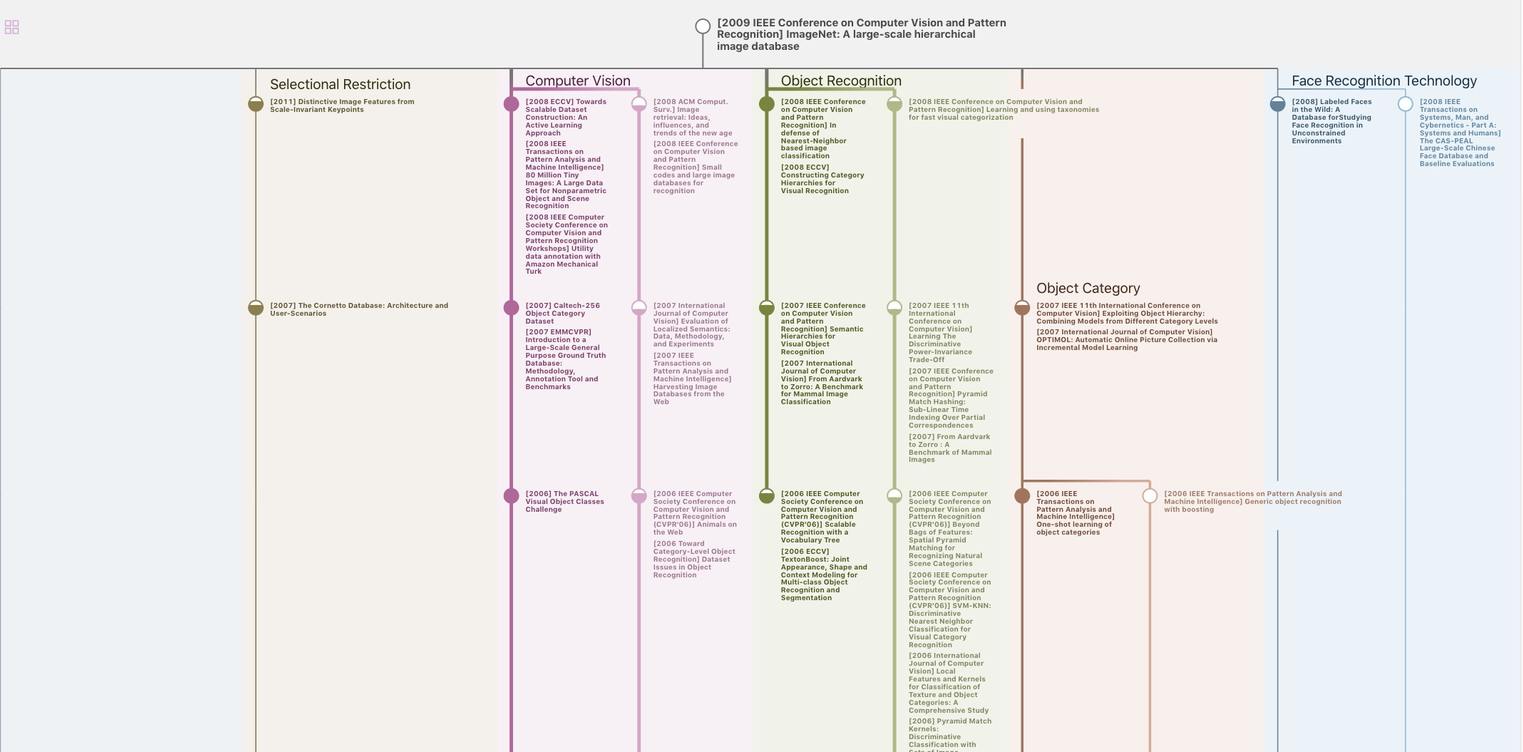
生成溯源树,研究论文发展脉络
Chat Paper
正在生成论文摘要