Multi-task learning based survival analysis for multi-source block-wise missing data.
Neurocomputing(2019)
摘要
There are many major diseases that remain incurable, such as cancer and Alzheimer’s disease (AD). Therefore, the prevention for these diseases has more impact than diagnosis and treatment. Survival analysis aims at predicting the time of occurrence of specific events of interest. It can be used to identify patients of high risk, which helps healthcare system effectively allocate limited medical resources. In many real-world applications, such as healthcare analysis, a lot of datasets are collected from multiple data sources and exhibit a block-wise missing pattern, i.e., each patient takes different types of tests and receives various treatments, and each test/treatment associates with a set of corresponding features. However, the existing survival analysis methods such as the Cox proportional hazards model and its extensions are designed for fully observed datasets and can not be directly applied when such block-wise missing pattern presents. This paper addresses this challenge and enables the survival prediction models to deal with high-dimensional multi-source block-wise missing data. Specifically, we employ a partition method that decomposes the multi-source block-wise missing data into multiple completed sub-matrix; thus, the original problem is transformed into a series of related multi-source survival analysis problems. To deal with these problems, we propose a two-layer multi-task learning framework that achieves both feature-level and source-level analysis, and the proposed framework is able to take advantage of the structure information in the block-wise missing pattern. Based on the proposed framework, we formulate two concrete models for survival analysis to handle multi-source block-wise missing data. We apply the proposed method in the real-world benchmark Alzheimer’s Disease Neuroimaging Initiative (ADNI) dataset and The Cancer Genome Atlas (TCGA) dataset to study the stage conversion of AD patients and survival time of cancer patients, respectively. The results from our comprehensive evaluations show that our methods outperform the state-of-the-art methods.
更多查看译文
关键词
Survival analysis,Multi-source,Multi-task learning,Block-wise missing data,Alzheimer’s disease,Cancer
AI 理解论文
溯源树
样例
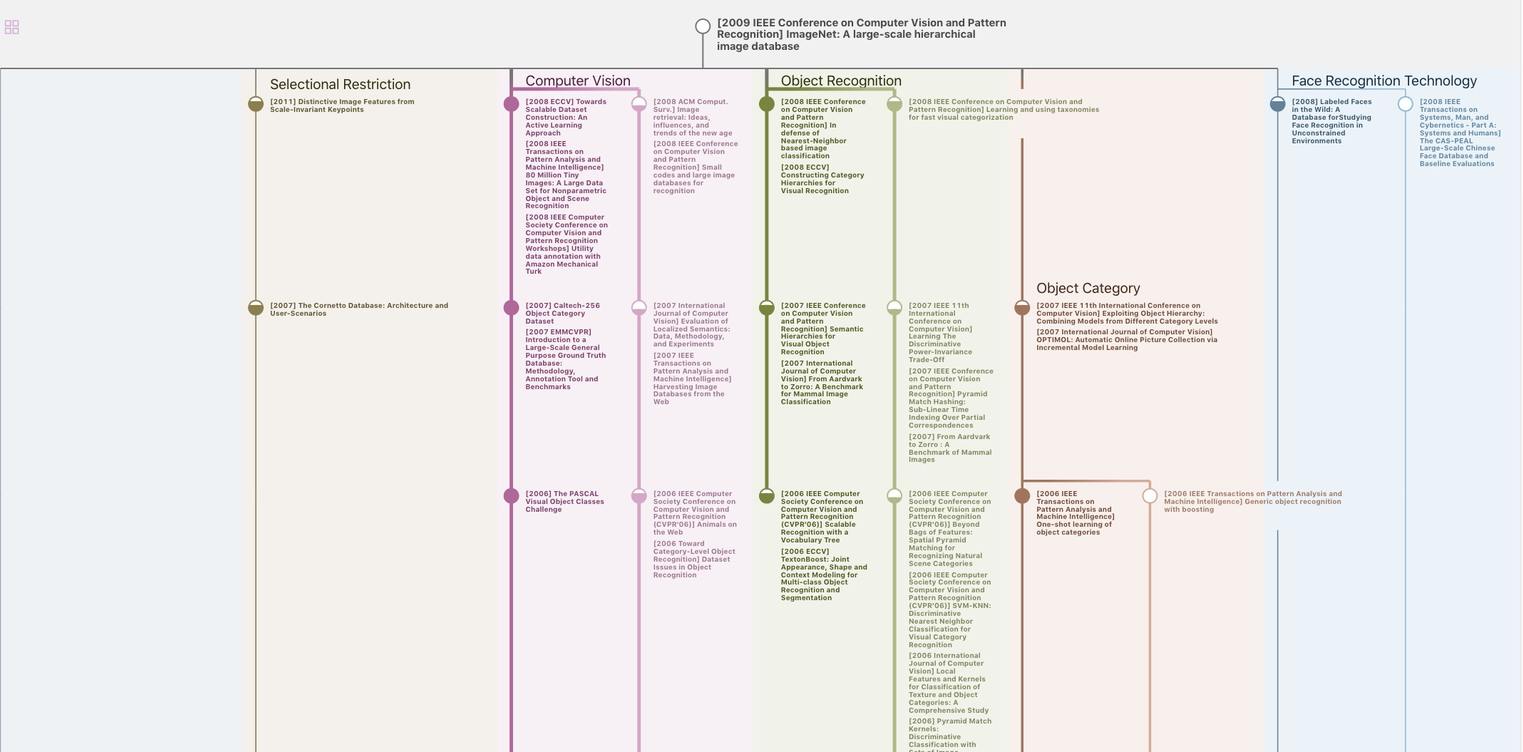
生成溯源树,研究论文发展脉络
Chat Paper
正在生成论文摘要