A risk model to predict severe postpartum hemorrhage in patients with placenta previa: a single-center retrospective study.
ANNALS OF PALLIATIVE MEDICINE(2019)
Abstract
Background: The study aimed to establish a predictive risk model for severe postpartum hemorrhage in placenta previa using clinical and placental ultrasound imaging performed prior to delivery. Methods: Postpartum hemorrhage patients were retrospectively enrolled. Severe postpartum hemorrhage was defined as exceeding 1,500 mL. Data collected included clinical and placental ultrasound images. Results: Age of pregnancy, time of delivery, time of miscarriage, history of vaginal delivery, gestational weeks at pregnancy termination, depth of placenta invading the uterine muscle wall were independent risk factors for severe postpartum hemorrhage in placenta previa. A model to predict severe postpartum hemorrhage in placenta previa was established: P=Log(Y/1-Y), where Y =-6.942 + 0.075 X-1 (age) +1.531 X-2 (times of delivery) + 0.223 X-3 (time of miscarriage) - 3.557X(4) (vaginal delivery: 1 for yes, 0 for no) + 1.753 X-5 (0 for <37 weeks, 1 for >= 37 weeks) + 1.574 X-6 (Depth of placenta invading uterine muscle wall: 0 for normal, 1 for placenta adhesion, 2 for placenta implantation, 3 for placenta penetration); discriminant boundary value of the prediction model (probability: P) was 0.268. Predicting sensitivity (<(S)over cap>(e)) =0.765 (negative predicting accuracy rate), specificity ((S) over cap (p)) =0.900 (positive predicting accuracy rate), total accuracy rate =0.795, and AUC of ROC curve =0.8419. Conclusions: The risk prediction model which had clinical and ultrasound imaging information prior to delivery had a high decision accuracy. However, before it can be used in the clinic, multicenter large-sample clinical studies should be performed to verify its accuracy and reliability.
MoreTranslated text
Key words
placenta previa,severe postpartum hemorrhage,prediction model,clinical information,placental ultrasound image characteristics
AI Read Science
Must-Reading Tree
Example
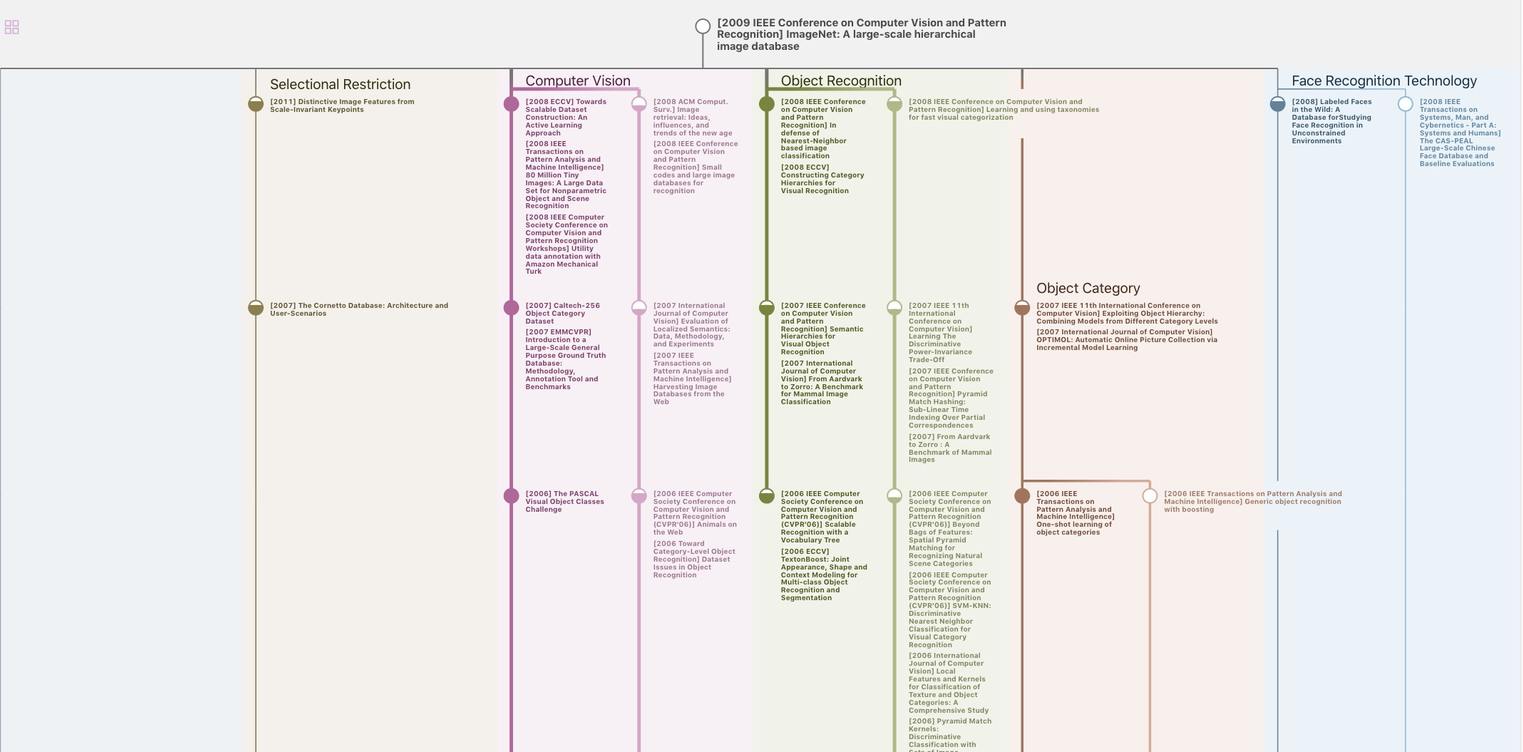
Generate MRT to find the research sequence of this paper
Chat Paper
Summary is being generated by the instructions you defined