Multi-Stage Pathological Image Classification using Semantic Segmentation
2019 IEEE/CVF INTERNATIONAL CONFERENCE ON COMPUTER VISION (ICCV 2019)(2019)
Abstract
Histopathological image analysis is an essential process for the discovery of diseases such as cancer. However, it is challenging to train CNN on whole slide images (WSIs) of gigapixel resolution considering the available memory capacity. Most of the previous works divide high resolution WSIs into small image patches and separately input them into the model to classify it as a tumor or a normal tissue. However, patch-based classification uses only patch-scale local information but ignores the relationship between neighboring patches. If we consider the relationship of neighboring patches and global features, we can improve the classification performance. In this paper, we propose a new model structure combining the patch-based classification model and whole slide-scale segmentation model in order to improve the prediction performance of automatic pathological diagnosis. We extract patch features from the classification model and input them into the segmentation model to obtain a whole slide tumor probability heatmap. The classification model considers patch-scale local features, and the segmentation model can take global information into account. We also propose a new optimization method that retains gradient information and trains the model partially for end-to-end learning with limited GPU memory capacity. We apply our method to the tumor/normal prediction on WSIs and the classification performance is improved compared with the conventional patch-based method.
MoreTranslated text
Key words
multistage pathological image classification,semantic segmentation,histopathological image analysis,CNN,gigapixel resolution,high resolution WSIs,image patches,patch-based classification model,slide-scale segmentation model,automatic pathological diagnosis,patch feature extraction,whole slide tumor probability heatmap,GPU memory capacity,normal tissue,gradient information,optimization method,cancer
AI Read Science
Must-Reading Tree
Example
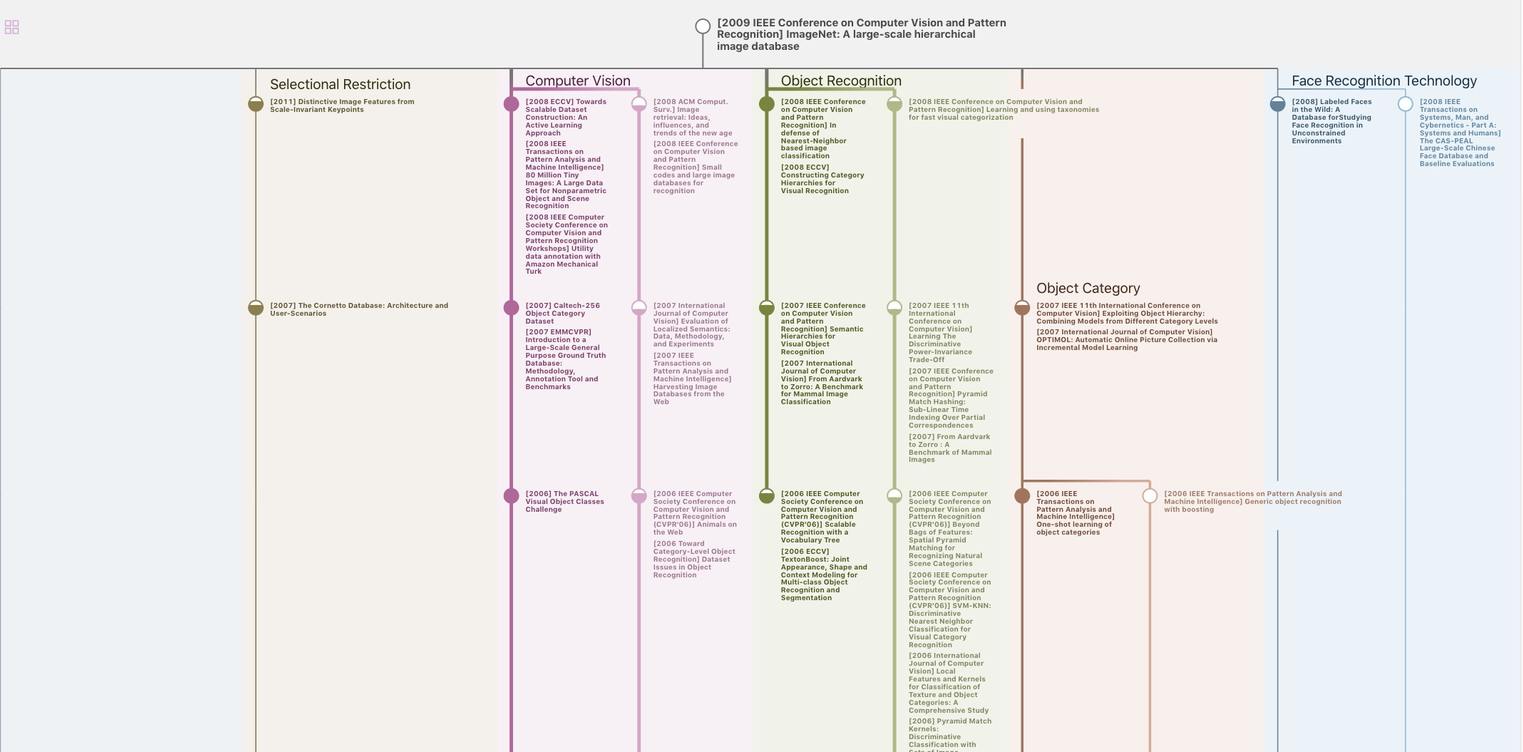
Generate MRT to find the research sequence of this paper
Chat Paper
Summary is being generated by the instructions you defined