Efficient Semi-Supervised Learning For Natural Language Understanding By Optimizing Diversity
2019 IEEE AUTOMATIC SPEECH RECOGNITION AND UNDERSTANDING WORKSHOP (ASRU 2019)(2019)
摘要
Expanding new functionalities efficiently is an ongoing challenge for single-turn task-oriented dialogue systems. In this work, we explore functionality-specific semi-supervised learning via self-training. We consider methods that augment training data automatically from unlabeled data sets in a functionality-targeted manner. In addition, we examine multiple techniques for efficient selection of augmented utterances to reduce training time and increase diversity. First, we consider paraphrase detection methods that attempt to find utterance variants of labeled training data with good coverage. Second, we explore sub-modular optimization based on n-grams features for utterance selection. Experiments show that functionality-specific self-training is very effective for improving system performance. In addition, methods optimizing diversity can reduce training data in many cases to 50% with little impact on performance.
更多查看译文
关键词
Dialog system, Data efficient learning, Paraphrase learning
AI 理解论文
溯源树
样例
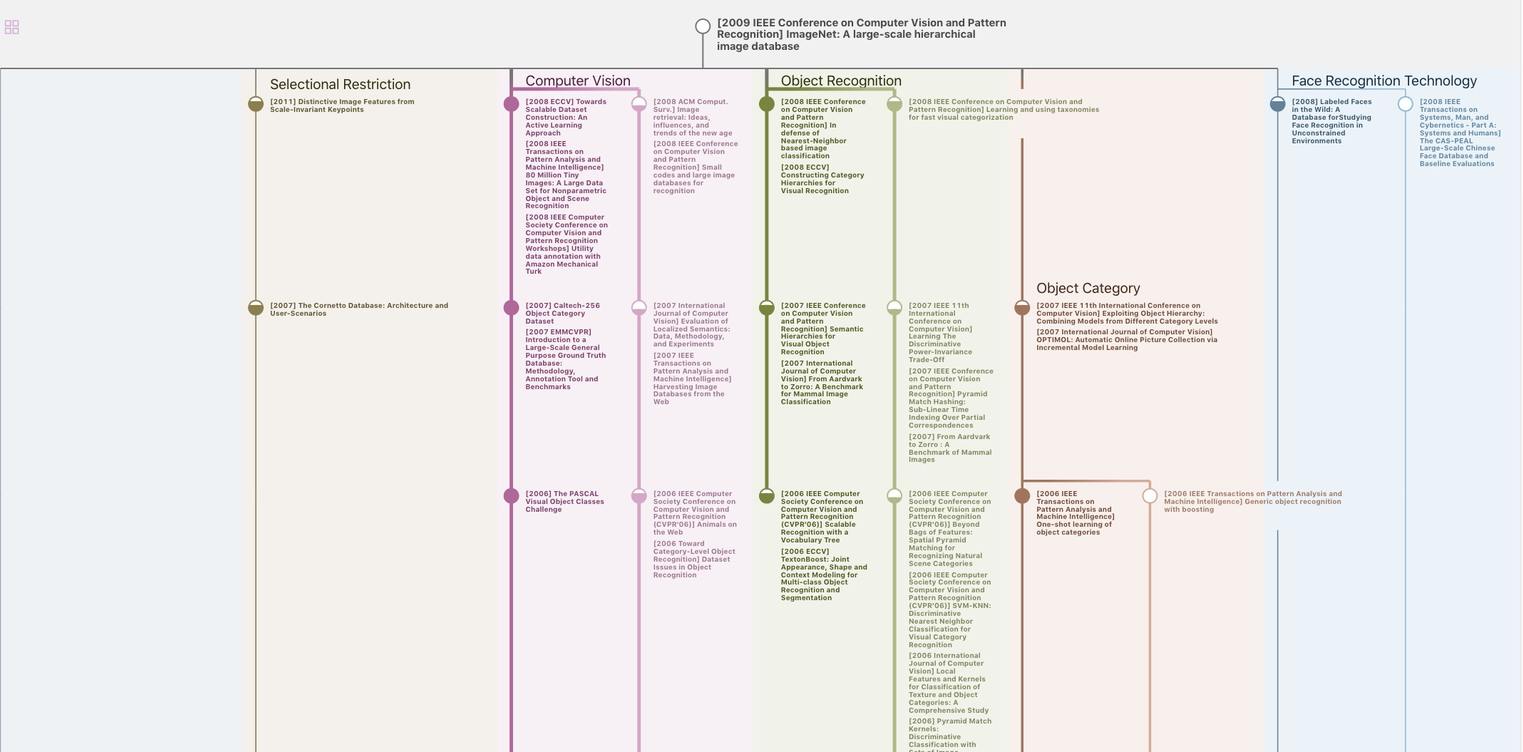
生成溯源树,研究论文发展脉络
Chat Paper
正在生成论文摘要