Backscatter-Assisted Computation Offloading for Energy Harvesting IoT Devices via Policy-based Deep Reinforcement Learning
2019 IEEE/CIC International Conference on Communications Workshops in China (ICCC Workshops)(2019)
摘要
Wireless Internet of Things (IoT) devices can be deployed for data acquisition and decision making, e.g., the wearable sensors used for healthcare monitoring. Due to limited computation capability, the low-power IoT devices can optionally offload power-consuming computation to a nearby computing server. To balance power consumption in data offloading and computation, we propose a novel hybrid data offloading scheme that allows each device to offload data via either the conventional RF communications or low-power backscatter communications. Such a flexibility makes it more complicated to optimize the offloading strategy with uncertain workload and energy supply at each device. As such, we propose the deep reinforcement learning (DRL) to learn the optimal offloading policy from past experience. In particular, we rely on the policy-based DRL approach for continuous control problems in the actor-critic framework. By interacting with the network environment, we can optimize each user's energy harvesting time and the workload allocation among different offloading schemes. The numerical results show that the proposed DRL approach can achieve much higher reward and learning speed compared to the conventional deep Q-network method.
更多查看译文
关键词
Deep reinforcement learning,DDPG,DQN,mobile data offloading,wireless backscatter
AI 理解论文
溯源树
样例
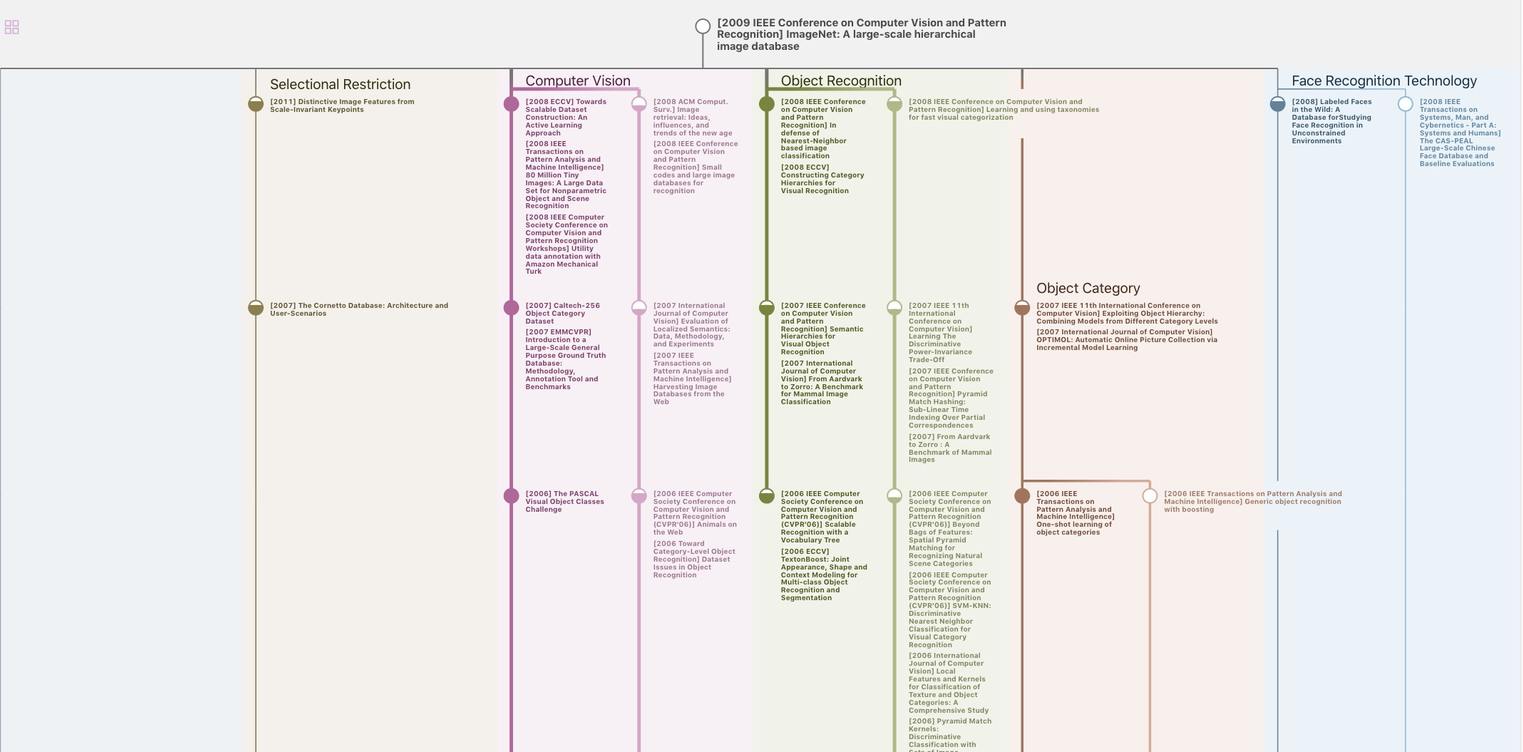
生成溯源树,研究论文发展脉络
Chat Paper
正在生成论文摘要