T-LSTM: A Long Short-Term Memory Neural Network Enhanced by Temporal Information for Traffic Flow Prediction
IEEE Access(2019)
摘要
Short-term traffic flow prediction is one of the most important issues in the field of intelligent transportation systems. It plays an important role in traffic information service and traffic guidance. However, complex traffic systems are highly nonlinear and stochastic, making short-term traffic flow prediction a challenging issue. Although long short-term memory (LSTM) has a good performance in traffic flow prediction, the impact of temporal features on prediction has not been exploited by existing studies. In this paper, a temporal information enhancing LSTM (T-LSTM) is proposed to predict traffic flow of a single road section. In view of the similar characteristics of traffic flow at the same time each day, the model can improve prediction accuracy by capturing the intrinsic correlation between traffic flow and temporal information. The experimental results demonstrate that our method can effectively improve the prediction performance and obtain higher accuracy compared with other state-of-the-art methods. Furthermore, we propose a novel missing data processing technique based on T-LSTM. According to the experimental results, this technique can well restore the characteristics of original data and improve the accuracy of traffic flow prediction.
更多查看译文
关键词
Traffic flow prediction,missing data repair,temporal features,deep learning,LSTM
AI 理解论文
溯源树
样例
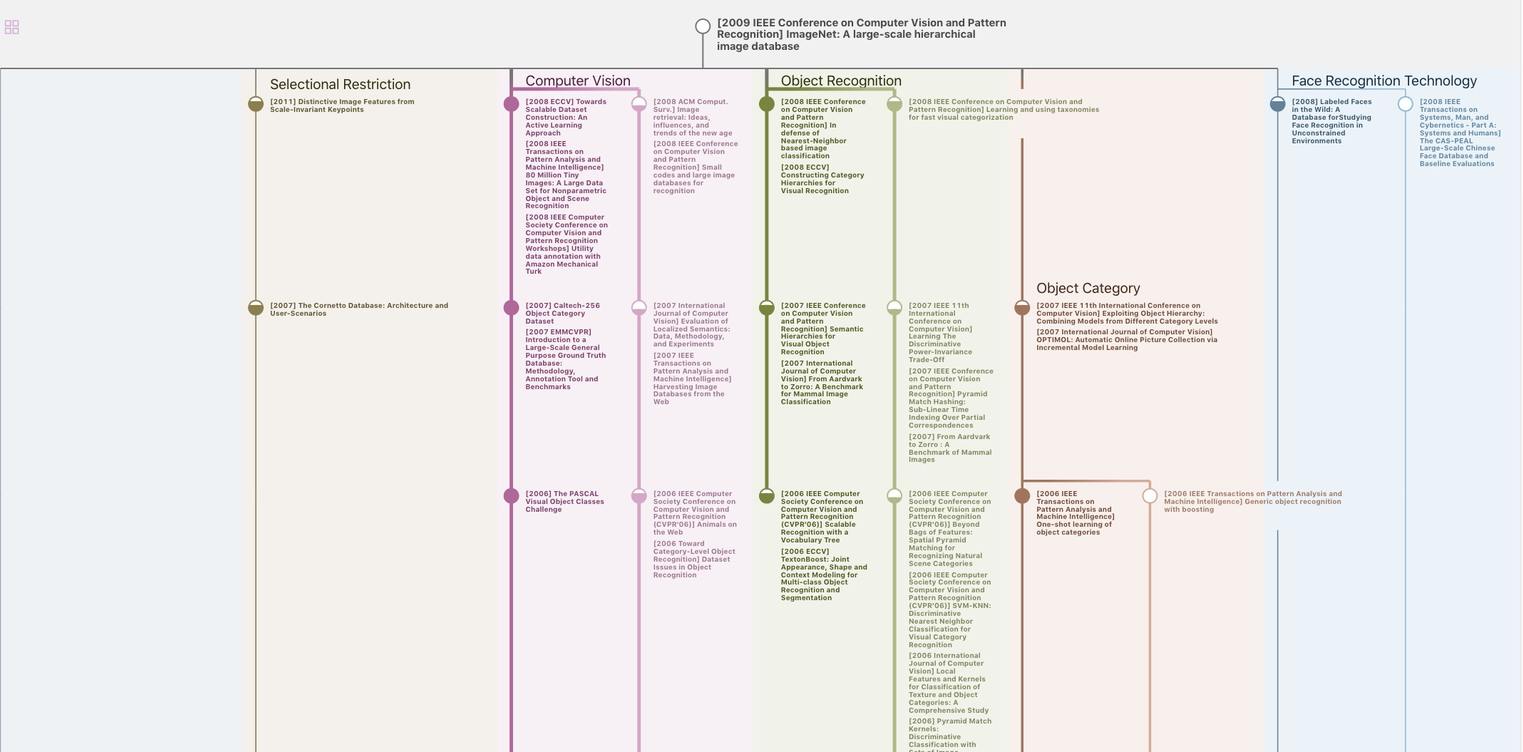
生成溯源树,研究论文发展脉络
Chat Paper
正在生成论文摘要