A Potts‐mixture spatiotemporal joint model for combined magnetoencephalography and electroencephalography data
CANADIAN JOURNAL OF STATISTICS-REVUE CANADIENNE DE STATISTIQUE(2019)
摘要
Abstract We develop a new methodology for determining the location and dynamics of brain activity from combined magnetoencephalography (MEG) and electroencephalography (EEG) data. The resulting inverse problem is ill-posed and is one of the most difficult problems in neuroimaging data analysis. In our development we propose a solution that combines the data from three different modalities, magnetic resonance imaging (MRI), MEG and EEG, together. We propose a new Bayesian spatial finite mixture model that builds on the mesostate-space model developed by Daunizeau & Friston [Daunizeau and Friston, NeuroImage 2007; 38, 67-81]. Our new model incorporates two major extensions: (i) We combine EEG and MEG data together and formulate a joint model for dealing with the two modalities simultaneously; (ii) we incorporate the Potts model to represent the spatial dependence in an allocation process that partitions the cortical surface into a small number of latent states termed mesostates. The cortical surface is obtained from MRI. We formulate the new spatiotemporal model and derive an efficient procedure for simultaneous point estimation and model selection based on the iterated conditional modes algorithm combined with local polynomial smoothing. The proposed method results in a novel estimator for the number of mixture components and is able to select active brain regions, which correspond to active variables in a high-dimensional dynamic linear model. The methodology is investigated using synthetic data and simulation studies and then demonstrated on an application examining the neural response to the perception of scrambled faces. R software implementing the methodology along with several sample datasets are available at the following GitHub repository . The Canadian Journal of Statistics 47: 688-711; 2019 (c) 2019 Statistical Society of Canada
更多查看译文
关键词
Bayesian mixture model,electromagnetic inverse problem,iterated conditional modes,Maxwell's equations,Potts model,simultaneous point estimation and model selection,spatiotemporal model
AI 理解论文
溯源树
样例
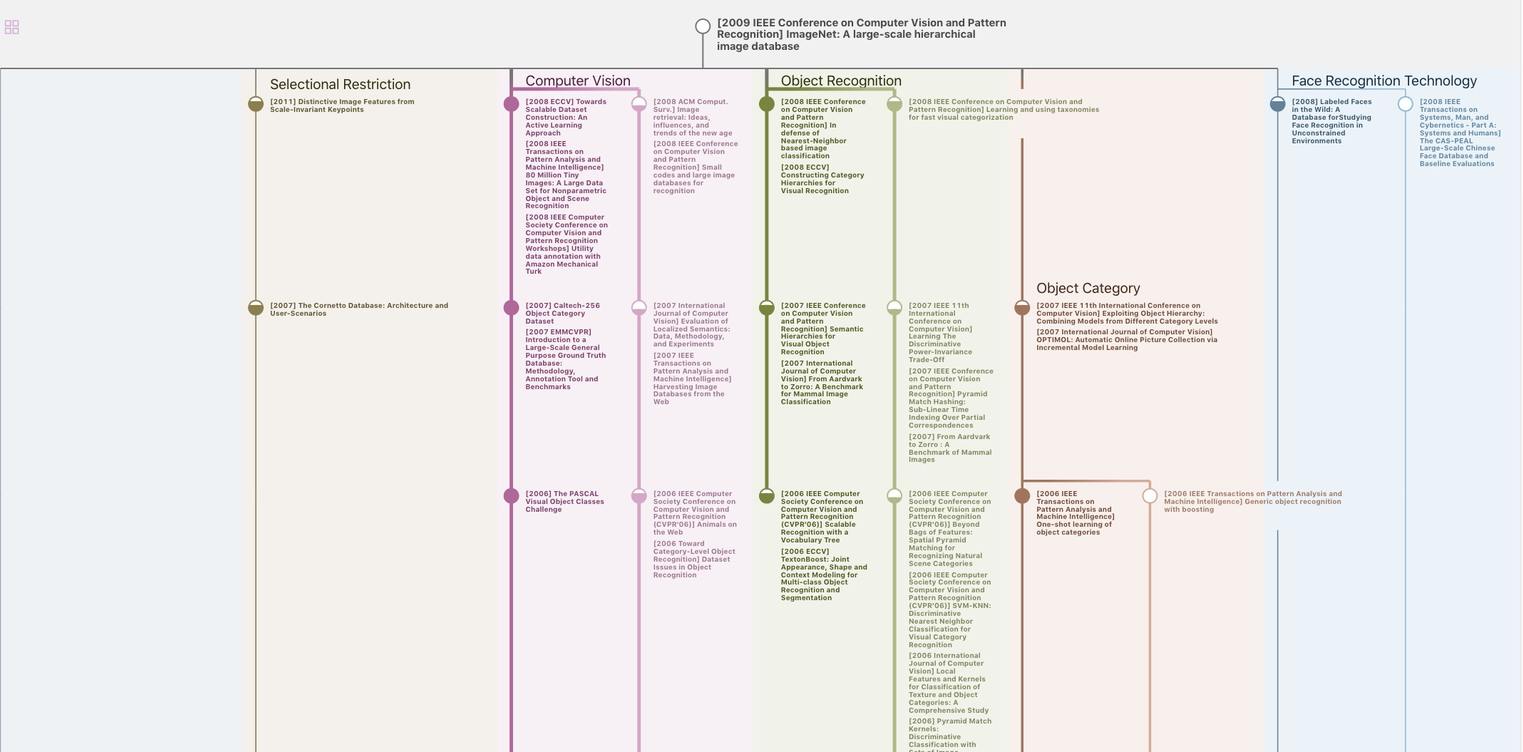
生成溯源树,研究论文发展脉络
Chat Paper
正在生成论文摘要