A nonparametric Bayesian methodology for regression discontinuity designs
Journal of Statistical Planning and Inference(2019)
摘要
One of the most popular methodologies for estimating the average treatment effect at the threshold in a regression discontinuity design is local linear regression (LLR), which places larger weight on units closer to the threshold. We propose a Gaussian process regression methodology that acts as a Bayesian analog to LLR for regression discontinuity designs. Our methodology provides a flexible fit for treatment and control responses by placing a general prior on the mean response functions. Furthermore, unlike LLR, our methodology can incorporate uncertainty in how units are weighted when estimating the treatment effect. We prove our method is consistent in estimating the average treatment effect at the threshold. Furthermore, we find via simulation that our method exhibits promising coverage, interval length, and mean squared error properties compared to standard LLR and state-of-the-art LLR methodologies. Finally, we explore the performance of our method on a real-world example by studying the impact of being a first-round draft pick on the performance and playing time of basketball players in the National Basketball Association.
更多查看译文
关键词
Regression discontinuity designs,Gaussian process regression,Bayesian nonparametrics,Coverage,Posterior consistency
AI 理解论文
溯源树
样例
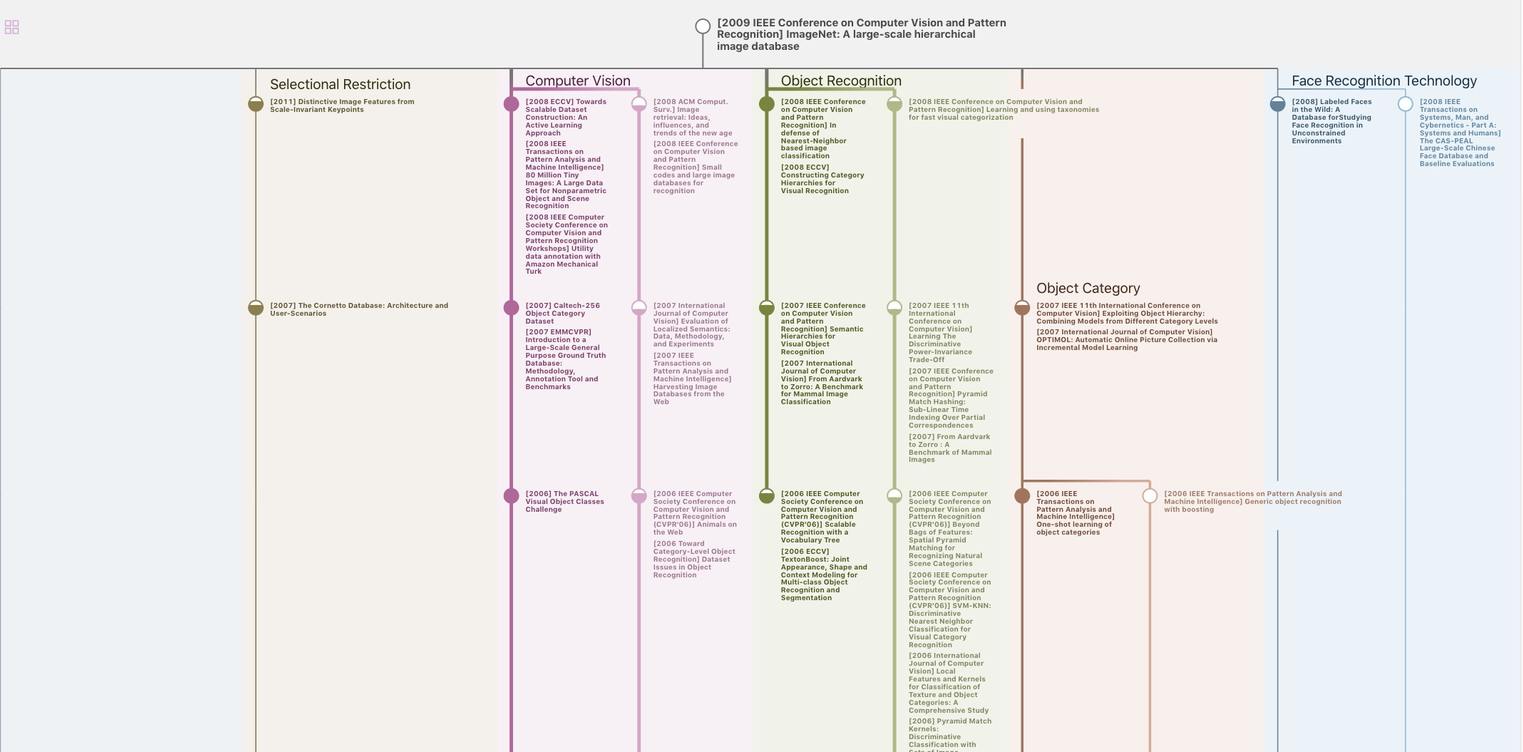
生成溯源树,研究论文发展脉络
Chat Paper
正在生成论文摘要