A class of multi-resolution approximations for large spatial datasets
STATISTICA SINICA(2020)
摘要
Gaussian processes are popular and flexible models for spatial, temporal, and functional data, but they are computationally infeasible for large data sets. We discuss Gaussian-process approximations that use basis functions at multiple resolutions to achieve fast inference and that can (approximately) represent any spatial covariance structure. We consider two special cases of this multi-resolution approximation framework, namely a taper version and a domain-partitioning (block) version. We describe theoretical properties and inference procedures, and study the computational complexity of the methods. Numerical comparisons and an application to satellite data are also provided.
更多查看译文
关键词
Basis functions,Gaussian process,kriging,predictive process,satellite data,sparsity
AI 理解论文
溯源树
样例
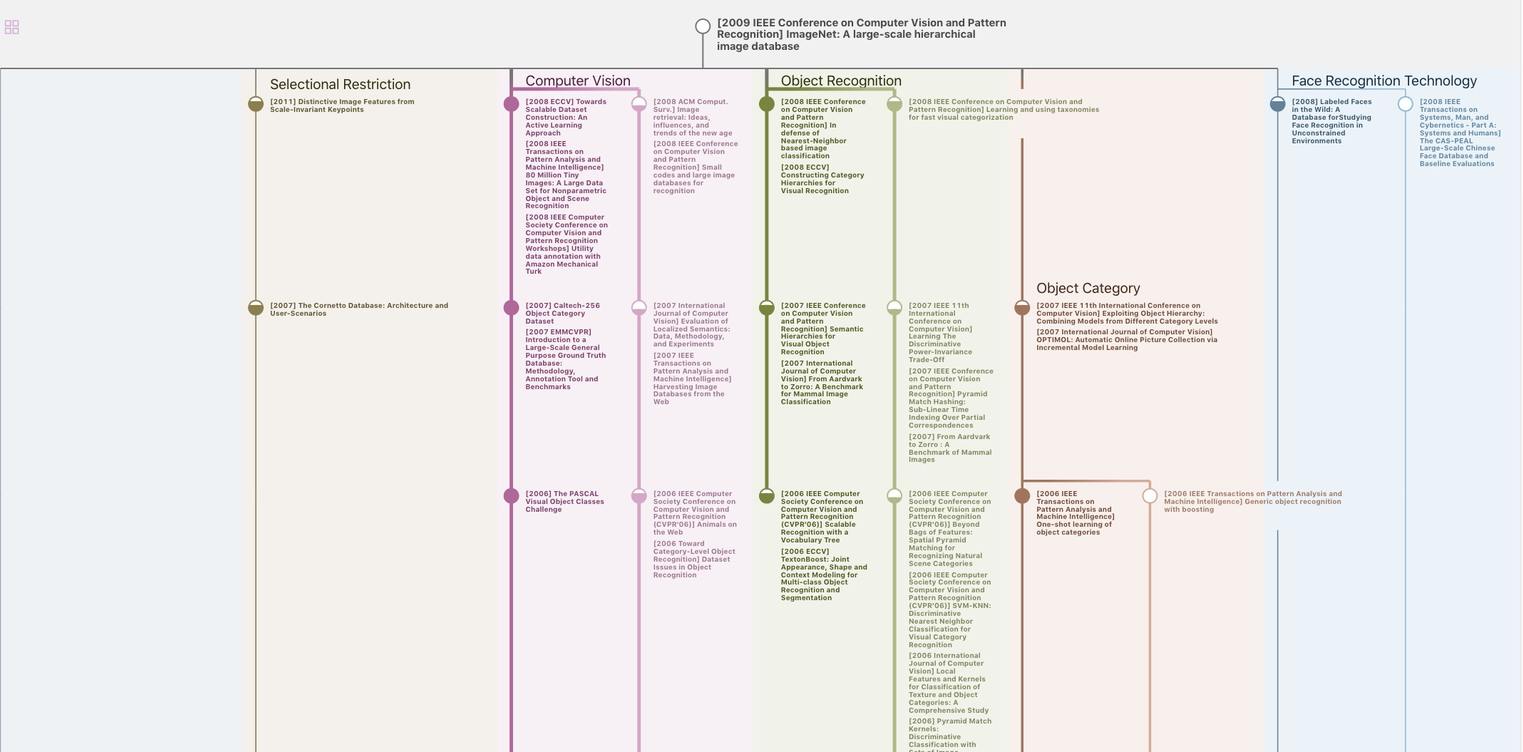
生成溯源树,研究论文发展脉络
Chat Paper
正在生成论文摘要