A Novel Image Fusion Framework based on Sparse Representation and Pulse Coupled Neural Network
IEEE Access(2019)
摘要
Image fusion techniques are applied to the synthesis of two or more images captured in the same scene to obtain a high-quality image. However, most of the existing fusion algorithms are aimed at single-mode images. To improve the fusion quality of multi-modal images, a novel multi-sensor image fusion framework based on non-subsampled shearlet transform (NSST) is proposed. First, the proposed solution uses NSST to decompose source images into high- and low-frequency components. Then, an improved pulse coupled neural network (PCNN) is proposed to process high-frequency components. Thus, the feature extraction effect of the high-frequency component is meliorated. After that, a sparse representation (SR) based measure, including compact dictionary learning and Max-L1 fusion rule, is designed to enhance the detailed features of the low-frequency component. Finally, the final image is obtained by the reconstruction of high- and low-frequency components via NSST inverse transformation. The proposed method is compared with several existing fusion methods. The experiment results show that the proposed algorithm outperforms other algorithms in both subjective and objective evaluation.
更多查看译文
关键词
Multi-sensor fusion,NSST,PCNN,sparse representation,dictionary learning,image fusion
AI 理解论文
溯源树
样例
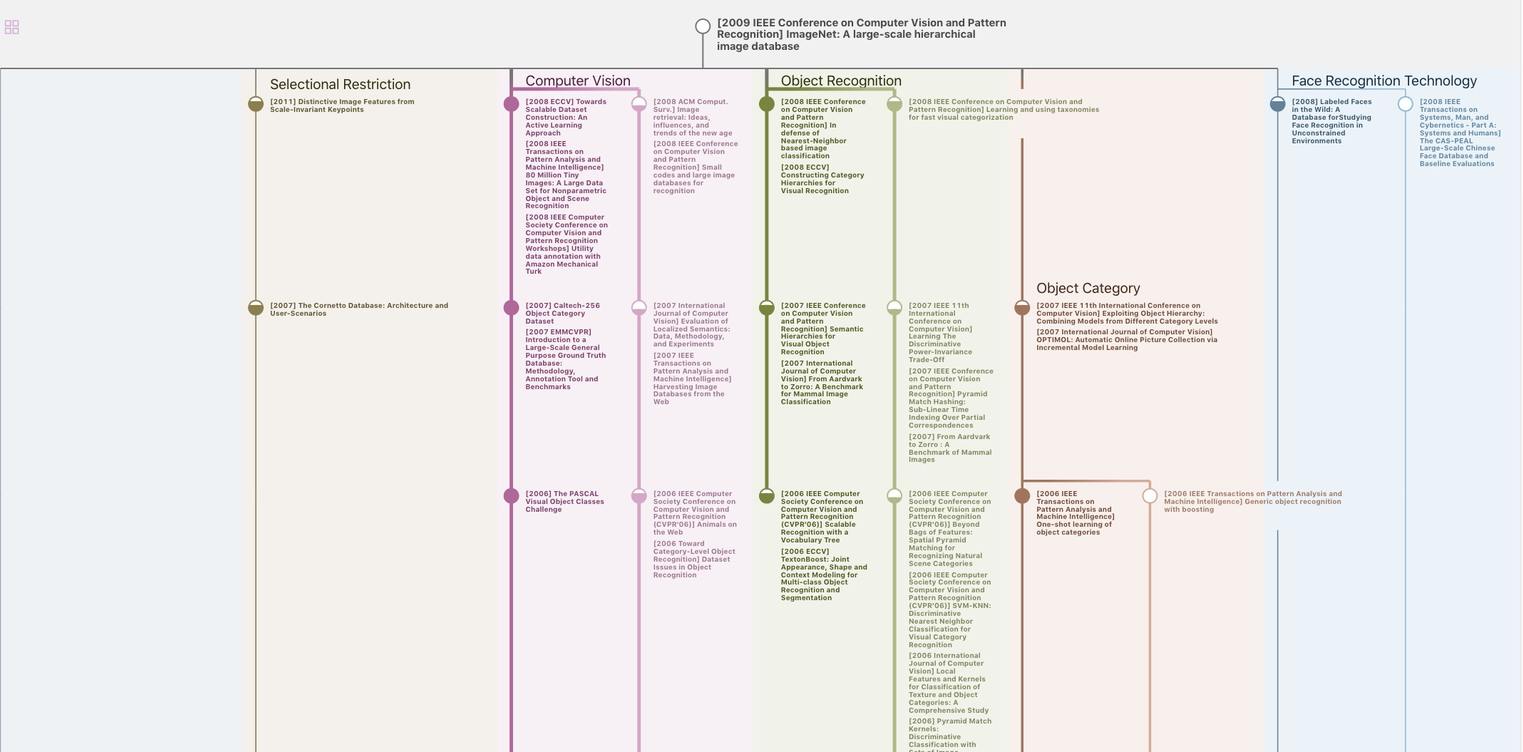
生成溯源树,研究论文发展脉络
Chat Paper
正在生成论文摘要