A structure with density-weighted active learning-based model selection strategy and meteorological analysis for wind speed vector deterministic and probabilistic forecasting
Energy(2019)
摘要
Accurate wind speed forecasting ensures the controllability for the wind power system. In this paper, a structure with density-weighted active learning (DWAL)-based model selection strategy from the perspective of meteorological factors is proposed to improve the accuracy and stability for wind speed deterministic and probabilistic forecasting. To improve training efficiency and accelerate the sample selection process, DWAL is employed. The multi-objective flower pollination algorithm is used to combine best models selected from model space with optimal weights for higher accuracy and stability. Except deterministic forecasts, as large-scale wind power generation integrated into power grid, the wind direction should also be forecasted and the estimation of the wind speed and direction uncertainty is vital, offering various aspects of forecasts for risk management. Thus, both deterministic and probabilistic forecasting for the wind speed vector are included in this paper. Eight datasets from Ontario Province, Canada, are utilized to evaluate forecasting performance of the model selection and the proposed structure. Results demonstrated: (a) the proposed structure is suitable for wind speed vector forecasting; (b) the proposed structure obtains more precise and stable forecasting performance; (c) the proposed structure improves the accuracy of deterministic forecasting and provides probabilistic information for wind speed vector forecasting.
更多查看译文
关键词
Density-weighted active learning strategy,Deterministic forecasting,Meteorological factors,Model selection,Probabilistic forecasting
AI 理解论文
溯源树
样例
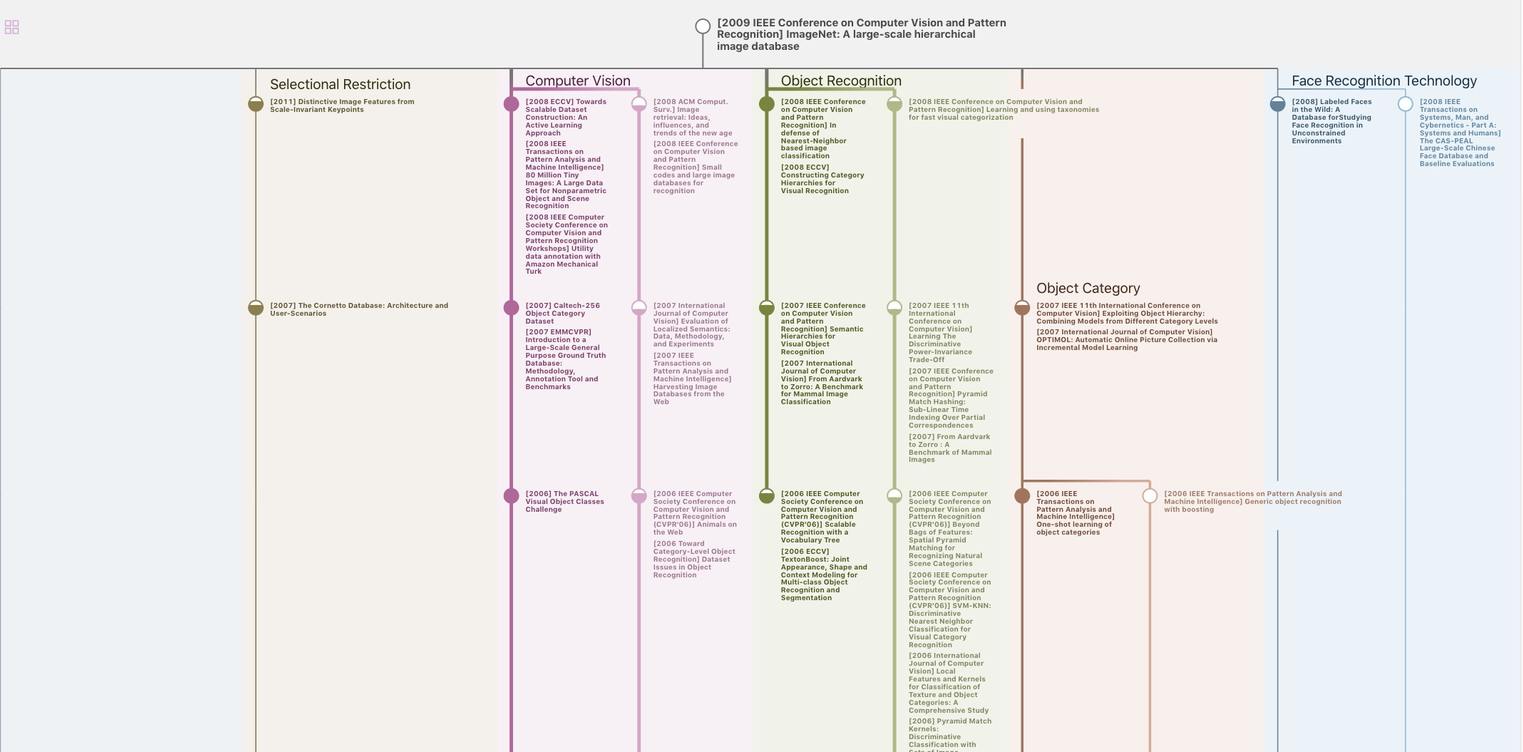
生成溯源树,研究论文发展脉络
Chat Paper
正在生成论文摘要