Learning Spectral Normalized Adversarial Systems With Stacked Structure For High-Quality 3d Object Generation
CONCURRENCY AND COMPUTATION-PRACTICE & EXPERIENCE(2021)
摘要
This paper proposes a new method for generating 3D objects based on generative adversarial networks (GANs). Recently, GANs have been used in 3D object generation, but it is still very challenging to generate high-quality 3D objects because of the complex data distribution over 3D objects. In this paper, we propose a system based on GAN that makes the generated objects more realistic. We use multiple generators and discriminators to enhance the ability of the model for learning complex distributions. Such a stacked structure can be considered as a coarse-to-fine or low-to-high-resolution mechanism. We employ the spectral normalization technology to control the Lipschitz constant of the discriminators by literally constraining the spectral norm of each layer to get a more stable training process. In this way, the proposed model can generate realistic and high-quality 3D objects. Moreover, our system can also recover incomplete 3D objects into complete 3D objects. Experiments demonstrate that our model performs better in the quality of the generated objects than the baselines.
更多查看译文
关键词
computer vision, GANs, model learning, 3D object generation, 3D shapes
AI 理解论文
溯源树
样例
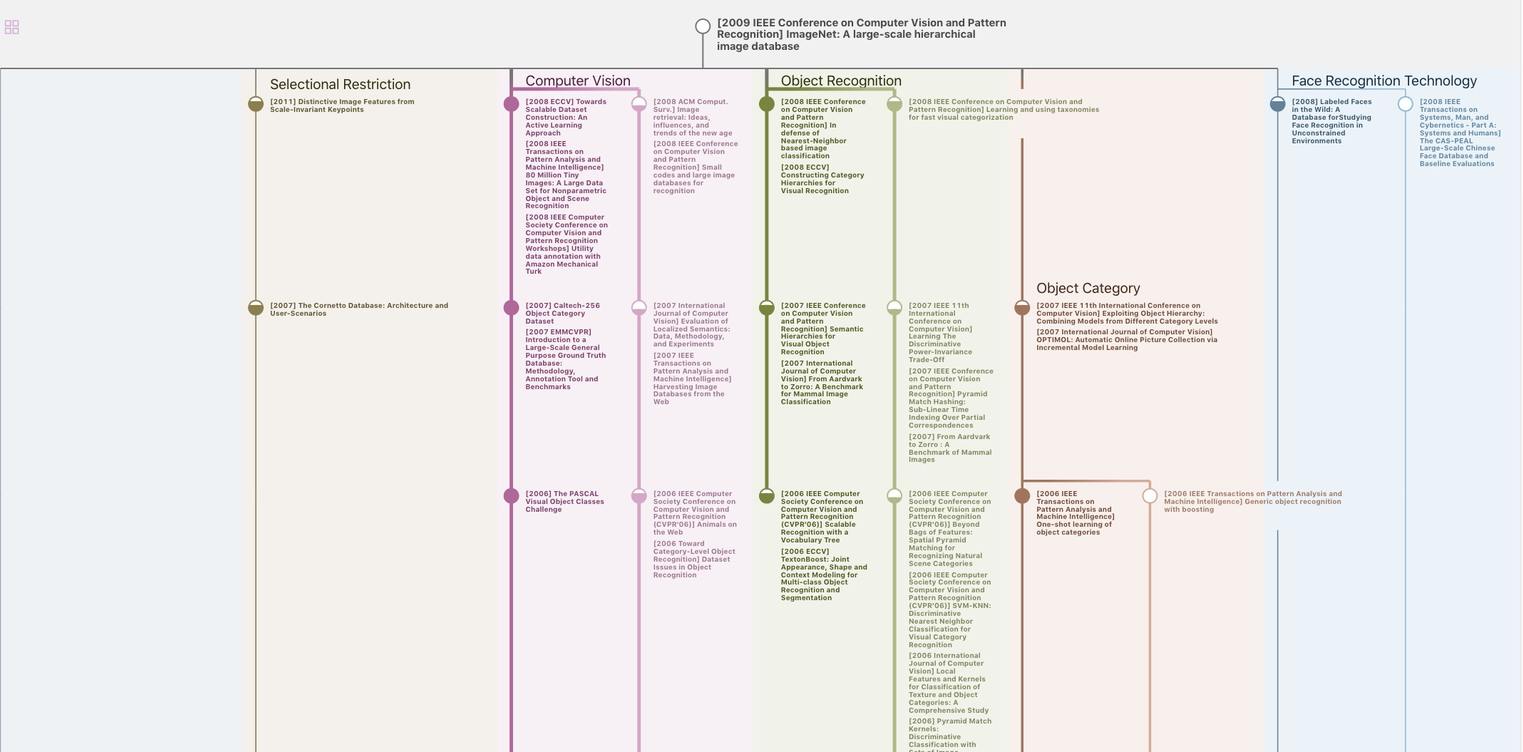
生成溯源树,研究论文发展脉络
Chat Paper
正在生成论文摘要