A reliable traffic prediction approach for bike‐sharing system by exploiting rich information with temporal link prediction strategy
TRANSACTIONS IN GIS(2019)
Abstract
Bike-sharing systems have been widely used in major cities across the world. As bike borrowing and return at different stations in different periods are not balanced, the bikes in a bike-sharing system need to be redistributed frequently to rebalance the system. Therefore, traffic flow forecasting of the bike-sharing system is an important issue, as this is conducive to achieving rebalancing of the bike system. In this article, we present a new traffic flow prediction approach based on the temporal links in dynamic traffic flow networks. A station clustering algorithm is first introduced to cluster stations into groups. A temporal link prediction method based on the dynamic traffic flow network method (STW+M) is then proposed to predict the traffic flow between stations. In our method, the non-negative tensor decomposition and time-series analysis model capture the rich information (temporal variabilities, spatial characteristics, and weather information) of the across-clusters transition. Then, a temporal link prediction strategy is used to forecast potential links and weights in the traffic flow network by investigating both the network structure and the results of tensor computations. In order to assess the methods proposed in this article, we have used the data of bike-sharing systems in New York and Washington, DC to conduct bike traffic prediction and the experimental results have shown that our method produces the lowest root mean square error (RMSE) and mean square error (MSE). Compared to four prediction methods from the literature, our RMSE and MSE of the two datasets have been lowered by an average of 2.55 (Washington, DC) and 2.41 (New York) and 3.35 (Washington, DC) and 2.96 (New York), respectively. The results show that the proposed approach improves predictions of traffic flow.
MoreTranslated text
Key words
reliable traffic prediction approach
AI Read Science
Must-Reading Tree
Example
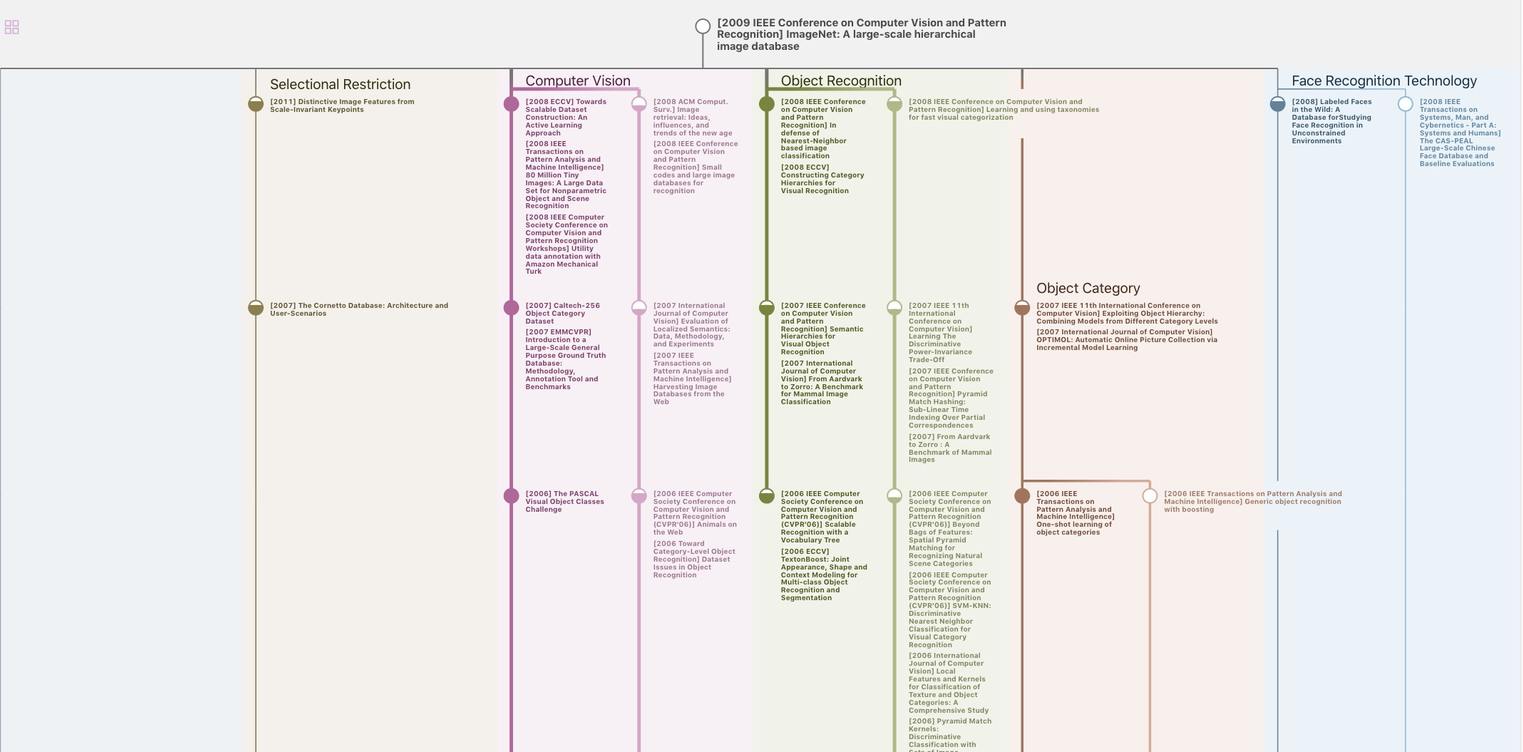
Generate MRT to find the research sequence of this paper
Chat Paper
Summary is being generated by the instructions you defined