Spectral clustering-based community detection using graph distance and node attributes
Computational Statistics(2019)
Abstract
Community detection is one of the main research topics in network analysis. Most network data reveal a certain structural relationship between nodes and provide attributes describing them. Utilizing available node attributes can help uncover latent communities from an observed network. In this paper, we propose a method of uncovering latent communities using both network structural information and node attributes so that the nodes within each community not only connect to other nodes in similar patterns but also share homogeneous attributes. The proposed method transforms the graph distance of nodes to structural similarity via the Gaussian kernel function. The attribute similarity between nodes is also measured by the Gaussian kernel function. Our method takes advantage of spectral clustering by appending node attributes to the node representation obtained from the network structure. Further, the proposed method has the ability to automatically learn the degree to which different attributes contribute. The solid performance of the proposed method is demonstrated in simulated data and four real-world networks.
MoreTranslated text
Key words
Network analysis,Assortative network,Structural similarity,Laplacian matrix,Stochastic block model
AI Read Science
Must-Reading Tree
Example
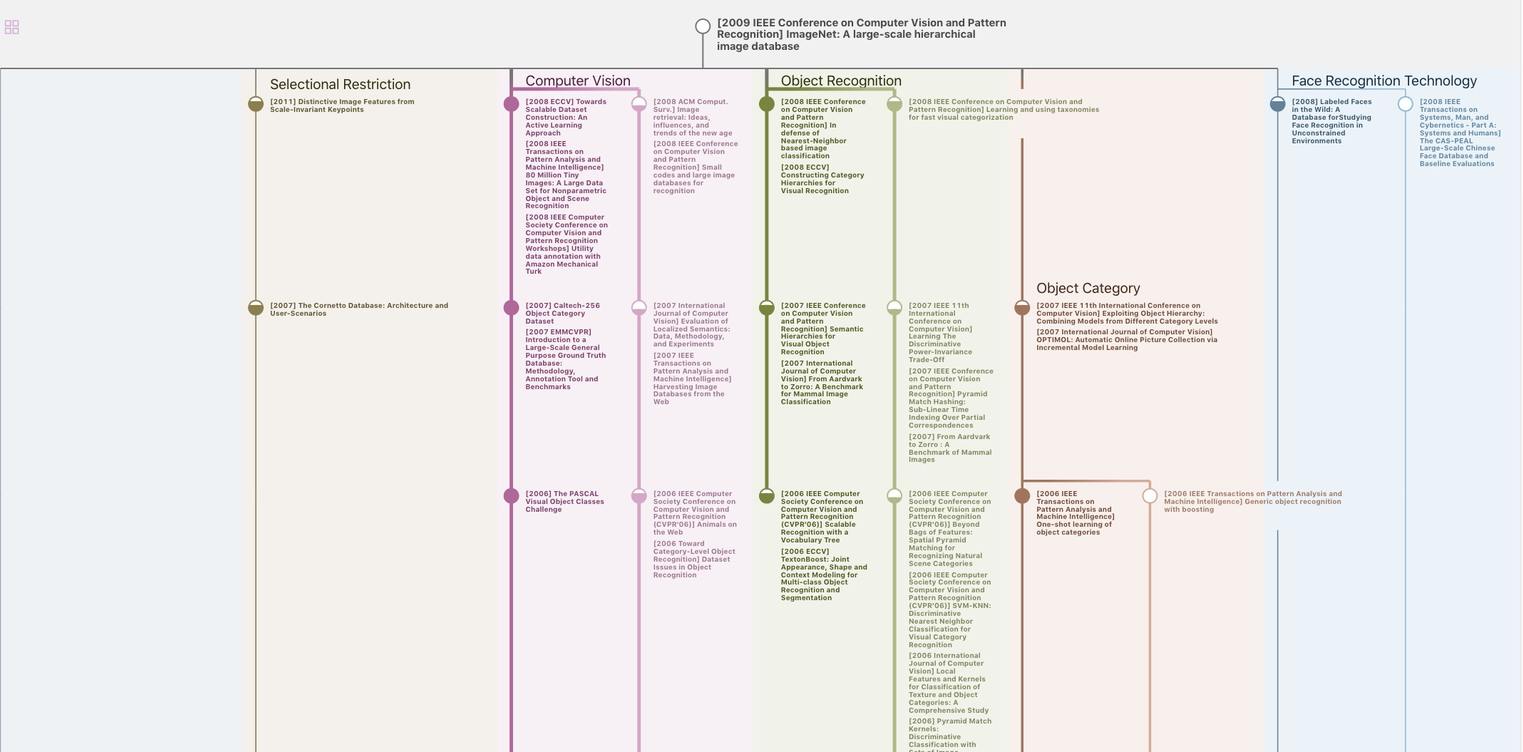
Generate MRT to find the research sequence of this paper
Chat Paper
Summary is being generated by the instructions you defined