Non-overlapping classification of hyperspectral imagery
REMOTE SENSING LETTERS(2019)
摘要
Because of high spectral resolution, hyperspectral imagery (HSI) is well suited for classifying land cover types. Spectral information and spatial context are often combined to improve classification performance. For several publicly available hyperspectral datasets, the classification accuracies reach almost 100%. However, we think this high accuracy is mainly due to heavy overlap between training and test samples generated during training and test stages. Because the training samples and test samples are randomly sampled from the same images and spatially adjacent to each other, overly optimistic results are produced. However, in real-life applications, the training samples and test samples may be collected from different locations or at different times. In order to improve the classification performance when training samples and test samples have low correlation, a low correlation sampling method and two non-overlapping classification methods are introduced. Experimental results show that, although the reduced correlation between training and test samples reduces the classification performance dramatically, the spectral-spatial combined method is still an effective way to improve the classification accuracy of HSI.
更多查看译文
AI 理解论文
溯源树
样例
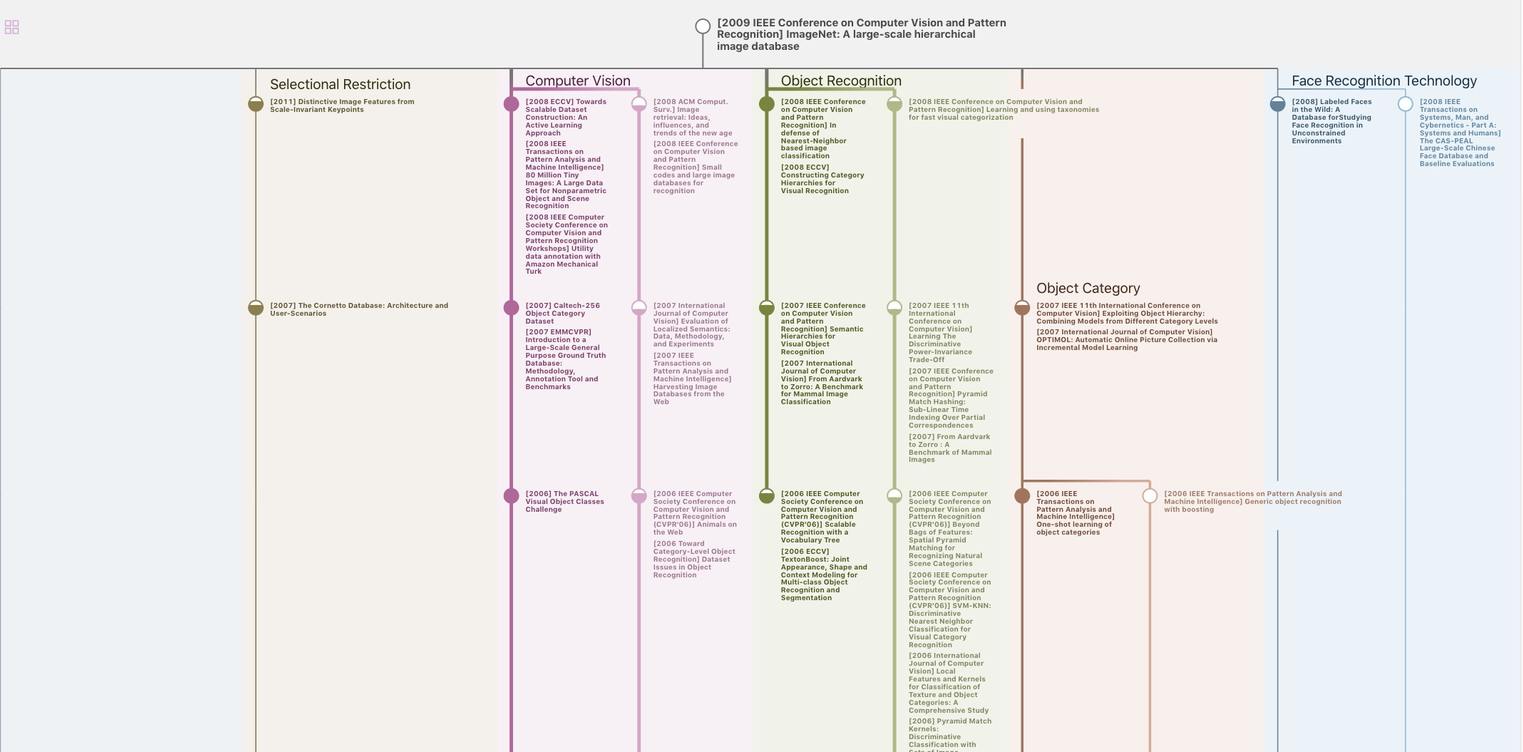
生成溯源树,研究论文发展脉络
Chat Paper
正在生成论文摘要