Improved state of charge estimation for Li-ion batteries using fractional order extended Kalman filter
Journal of Power Sources(2019)
Abstract
An accurate state of charge (SõC) estimation by the battery management system (BMS) is crucial for efficient and non-destructive battery operation in automotive applications. The model identification of these batteries has consistently been the critical point to meet good accuracy. To that extent, a fractional order model (FOM) is derived, which provides a more meaningful insight into the battery physical phenomena without increasing the number of parameters as opposed to electrochemical models. This paper proposes FOM identification for Li-ion batteries in both frequency domain based on recorded impedance spectroscopy (EIS) data and time domain using a recursive least squares (RLS) algorithm. Fractional derivatives are overly sensitive to the value of their fractional order. A straightforward and efficient way to identify the fractional orders based on recorded EIS data is proposed in this paper. Furthermore, an extended Kalman filter (EKF) is also designed based on the derived model to estimate the SõC. The designed fractionasl order filter provides a higher accuracy level in comparison to the classical equivalent electric circuit (EEC). Various results at several temperatures and driving profiles for both PHEV and EV batteries confirm that the FOM provides better accuracy and robustness compared to the classical integer order model.
MoreTranslated text
Key words
Li-ion battery,Fractional order model,Electrochemical impedance spectroscopy,EKF,SõC estimation,Recursive identification
AI Read Science
Must-Reading Tree
Example
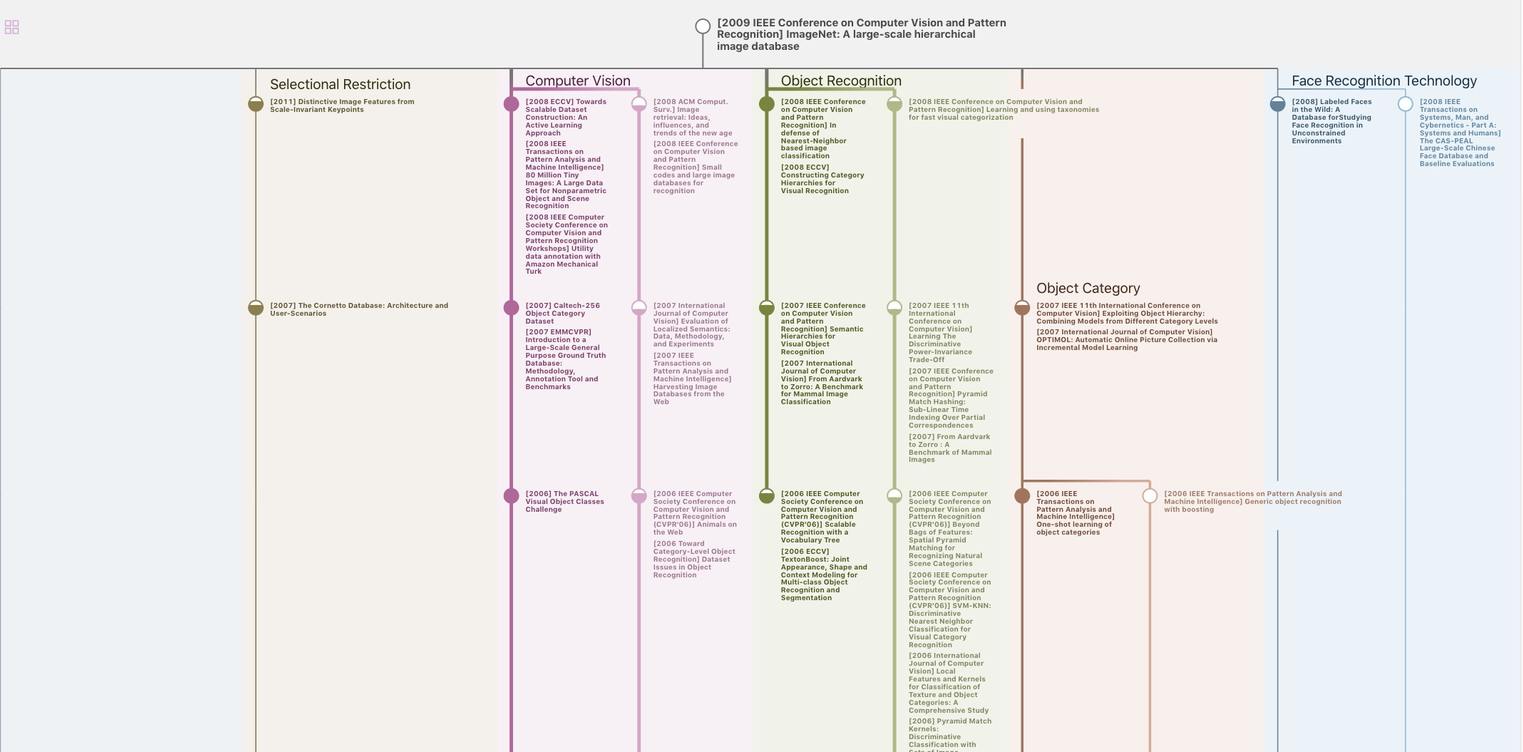
Generate MRT to find the research sequence of this paper
Chat Paper
Summary is being generated by the instructions you defined