Indoor scene understanding via RGB-D image segmentation employing depth-based CNN and CRFs
MULTIMEDIA TOOLS AND APPLICATIONS(2019)
摘要
With the availability of low-cost depth-visual sensing devices, such as Microsoft Kinect, we are experiencing a growing interest in indoor environment understanding, at the core of which is semantic segmentation in RGB-D image. The latest research shows that the convolutional neural network (CNN) still dominates the image semantic segmentation field. However, down-sampling operated during the training process of CNNs leads to unclear segmentation boundaries and poor classification accuracy. To address this problem, in this paper, we propose a novel end-to-end deep architecture, termed FuseCRFNet, which seamlessly incorporates a fully-connected Conditional Random Fields (CRFs) model into a depth-based CNN framework. The proposed segmentation method uses the properties of pixel-to-pixel relationships to increase the accuracy of image semantic segmentation. More importantly, we formulate the CRF as one of the layers in FuseCRFNet to refine the coarse segmentation in the forward propagation, in meanwhile, it passes back the errors to facilitate the training. The performance of our FuseCRFNet is evaluated by experimenting with SUN RGB-D dataset, and the results show that the proposed algorithm is superior to existing semantic segmentation algorithms with an improvement in accuracy of at least 2%, further verifying the effectiveness of the algorithm.
更多查看译文
关键词
Sematic segmentation, CNNs, RGB-D, Fully-connected conditional random field
AI 理解论文
溯源树
样例
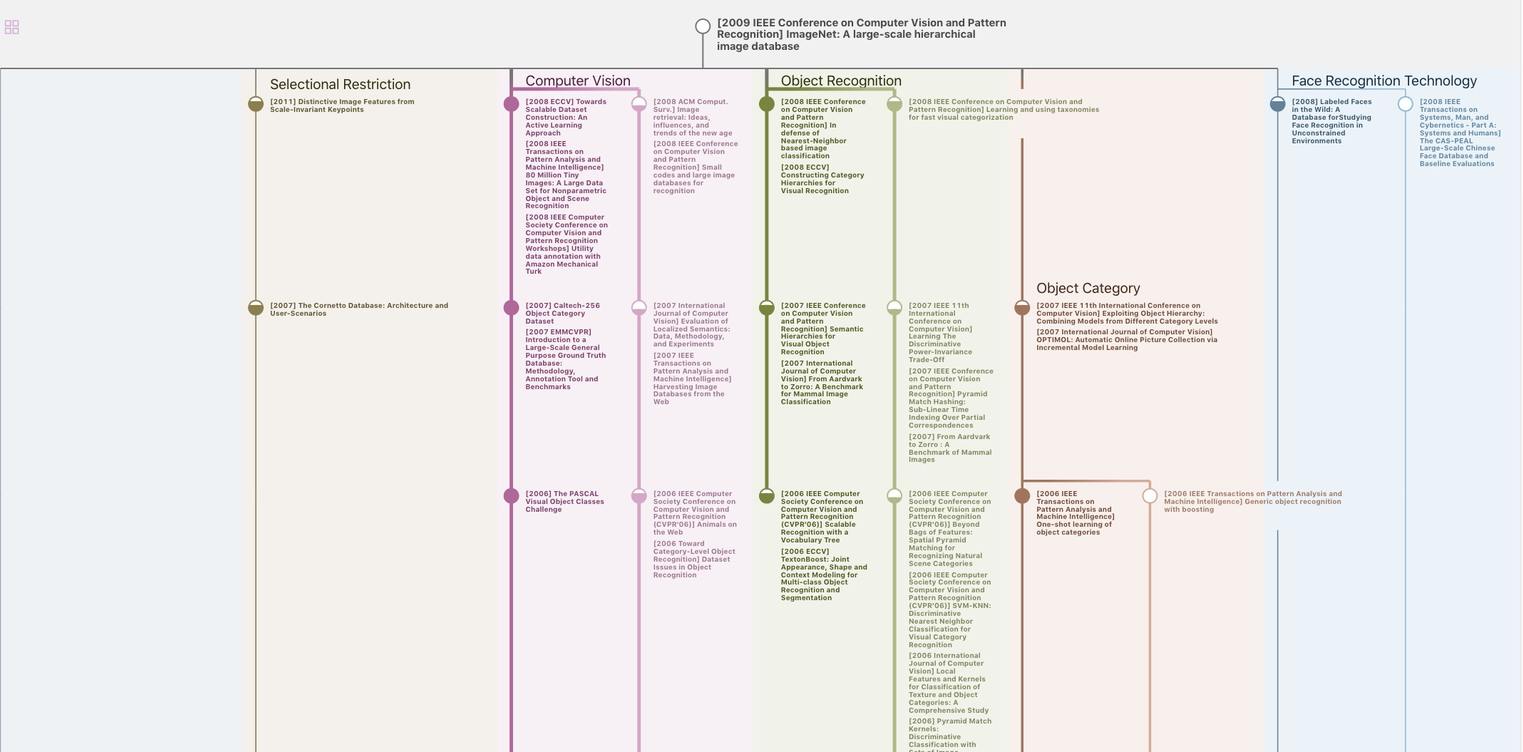
生成溯源树,研究论文发展脉络
Chat Paper
正在生成论文摘要