Machine Learning, Markov Chain Monte Carlo, And Optimal Algorithms To Characterize The Advact Kilopixel Transition-Edge Sensor Arrays
IEEE TRANSACTIONS ON APPLIED SUPERCONDUCTIVITY(2019)
摘要
Next-generation focal planes comprising dozens of kilopixel transition-edge sensor (TES) arrays require new methods to rapidly screen candidate arrays, evaluate array non-idealities in the field, identify outlier devices for removal, and optimize the array performance in the field. We demonstrate robust methods to estimate TES parameters (critical temperatures and thermal conductivity parameters) and their uncertainties using a custom Markov Chain Monte Carlo (MCMC) algorithm. We also constrain systematic effects in estimating the TES parameters from non-isothermal current-voltage curves (IVs) at approximately a similar to 3% level. Additionally, for the first time, we have applied Machine Learning (ML) algorithms to tune detector arrays and optimize their performance.
更多查看译文
关键词
Kilopixel focal planes, Machine Learning, Markov Chain Monte Carlo, Transition-Edge Sensor
AI 理解论文
溯源树
样例
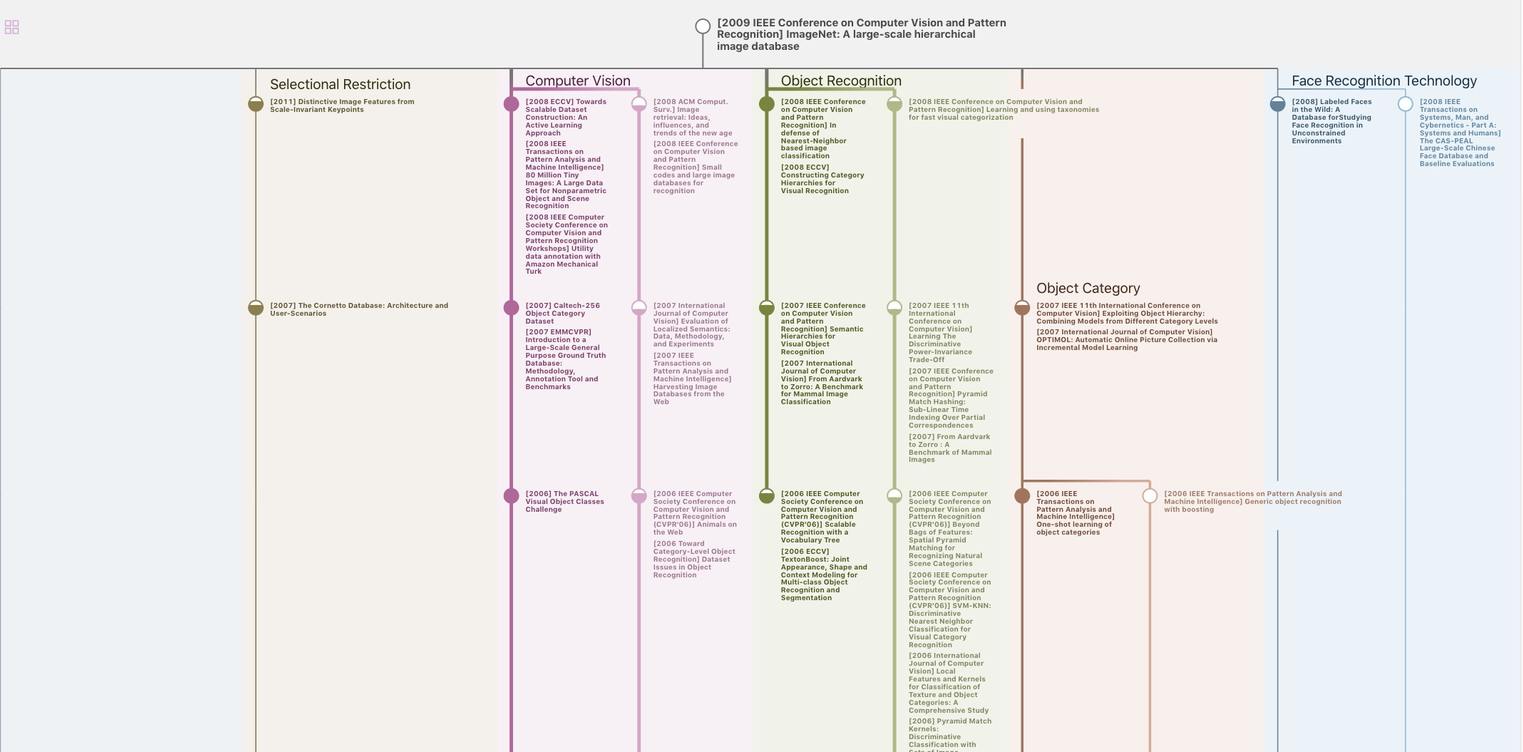
生成溯源树,研究论文发展脉络
Chat Paper
正在生成论文摘要