Improved svrg for finite sum structure optimization with application to binary classification
JOURNAL OF INDUSTRIAL AND MANAGEMENT OPTIMIZATION(2020)
Abstract
This paper looks at a stochastic variance reduced gradient (SVRG) method for minimizing the sum of a finite number of smooth convex functions, which has been involved widely in the field of machine learning and data mining. Inspired by the excellent performance of two-point stepsize gradient method in batch learning, in this paper we present an improved SVRG algorithm, named stochastic two-point stepsize gradient method. Under some mild conditions, the proposed method achieves a linear convergence rate O(rho(k)) for smooth and strongly convex functions, where rho is an element of (0.68, 1). Simulation experiments on several benchmark data sets are reported to demonstrate the performance of the proposed method.
MoreTranslated text
Key words
Machine leaning,online learning,stochastic optimization,variance reduction,two-point stepsize
AI Read Science
Must-Reading Tree
Example
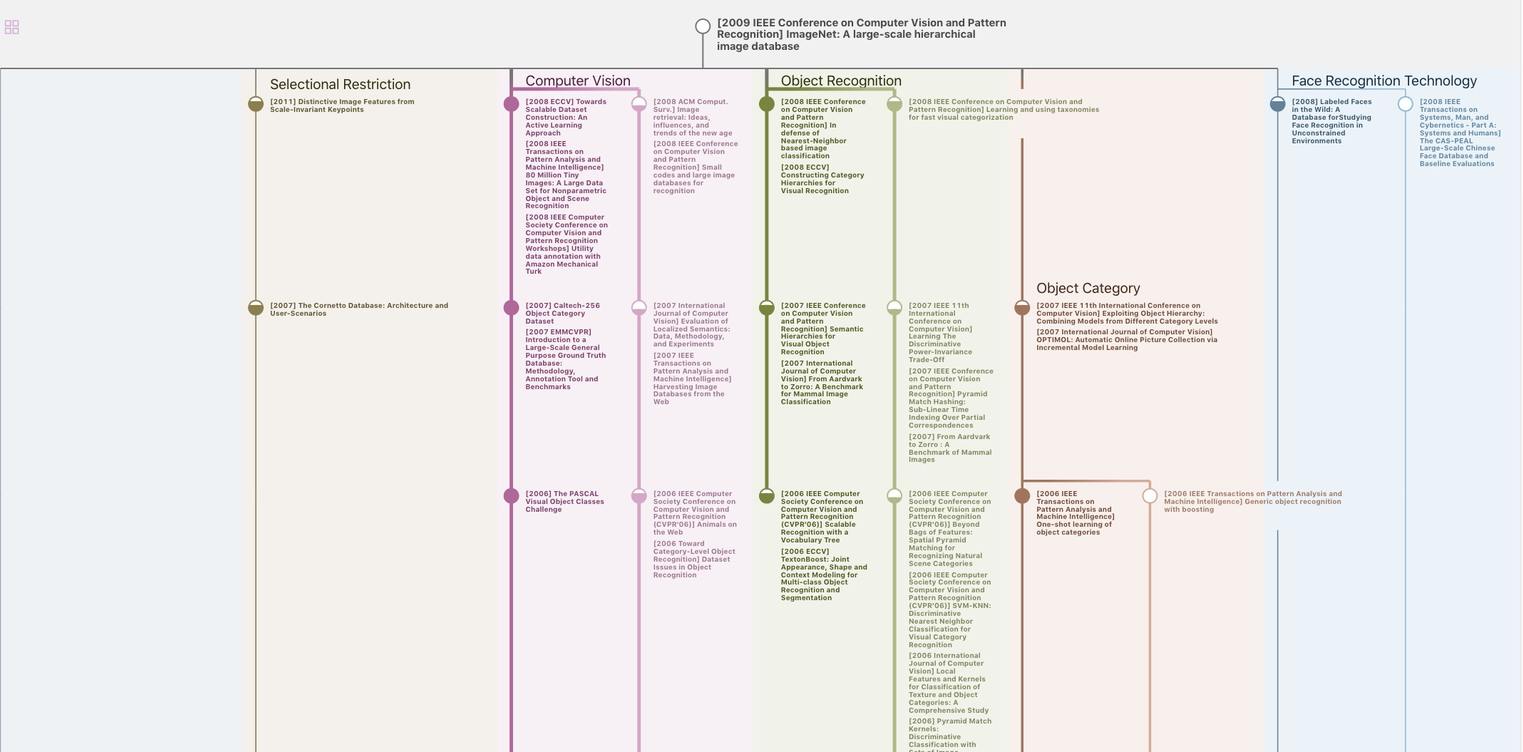
Generate MRT to find the research sequence of this paper
Chat Paper
Summary is being generated by the instructions you defined