Reversing Gradients In Adversarial Domain Adaptation For Question Deduplication And Textual Entailment Tasks
57TH ANNUAL MEETING OF THE ASSOCIATION FOR COMPUTATIONAL LINGUISTICS (ACL 2019)(2019)
摘要
Adversarial domain adaptation has been recently introduced as an effective technique for textual matching tasks, such as question deduplication (Shah et al., 2018). Here we investigate the use of gradient reversal on adversarial domain adaptation to explicitly learn both shared and unshared (domain specific) representations between two textual domains. In doing so, gradient reversal learns features that explicitly compensate for domain mismatch, while still distilling domain specific knowledge that can improve target domain accuracy. We evaluate reversing gradients for adversarial adaptation on multiple domains, and demonstrate that it significantly outperforms other methods on question deduplication as well as on recognizing textual entailment (RTE) tasks, achieving up to 7% absolute boost in base model accuracy on some datasets.
更多查看译文
AI 理解论文
溯源树
样例
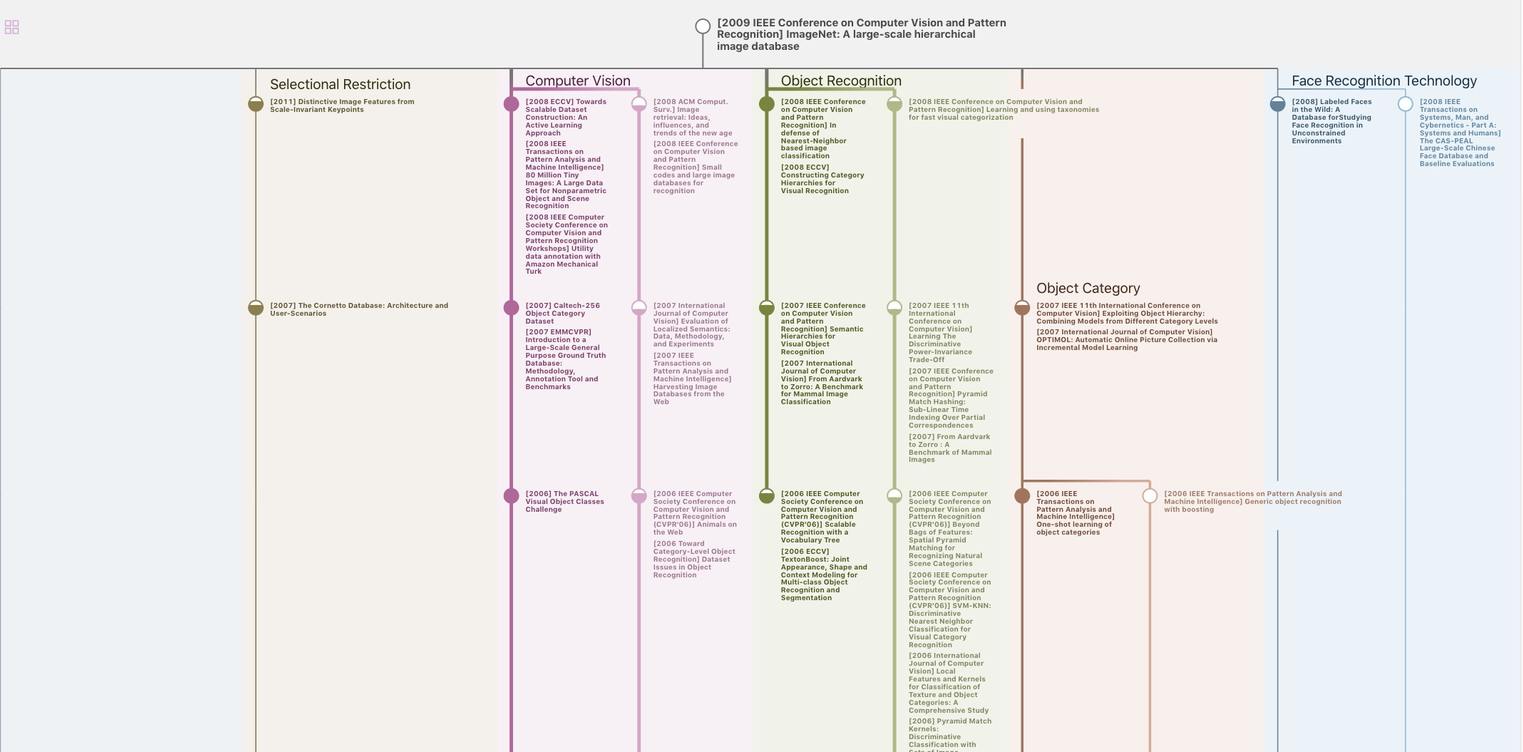
生成溯源树,研究论文发展脉络
Chat Paper
正在生成论文摘要