On the optimal solution to maximum margin projection pursuit
MULTIMEDIA TOOLS AND APPLICATIONS(2019)
摘要
Most existing dimensionality reduction methods have been applied as a separable data preprocessing step before classification algorithms. This reduces the flexibility of classification algorithms. To handle this limitation, recently, a novel method, namely maximum margin projection pursuit (MMPP), was developed by simultaneously taking into account dimensionality reduction and classification in the criterion function. MMPP alternatively updates the projection matrix and normal vector of classifications hyperplane by optimizing the min-max problem. This results in the following two problems: (1) It does not guarantee both the convergence of the proposed iterative algorithm in real applications and minimization of the objective function; (2) It heavily depends on learning rate and does not get the global optimal solution. In this paper, we simultaneously solve both the projection matrix and norm vector of classification hyperplane by non-iterative method which not only optimizes the criterion function but also is faster than traditional MMPP algorithm. Furthermore, we extend our method to solve multiclass classification problems. Experiments on the Yale, ORL, AR and COIL20 databases have been conducted to evaluate our method. The results illustrate that, compared with the iterative algorithm, our no-iteration algorithm achieves higher efficiency, more stable recognition, and smaller objective value.
更多查看译文
关键词
Maximum margin projections, Support vector machines, Dimensionality reduction, Face recognition
AI 理解论文
溯源树
样例
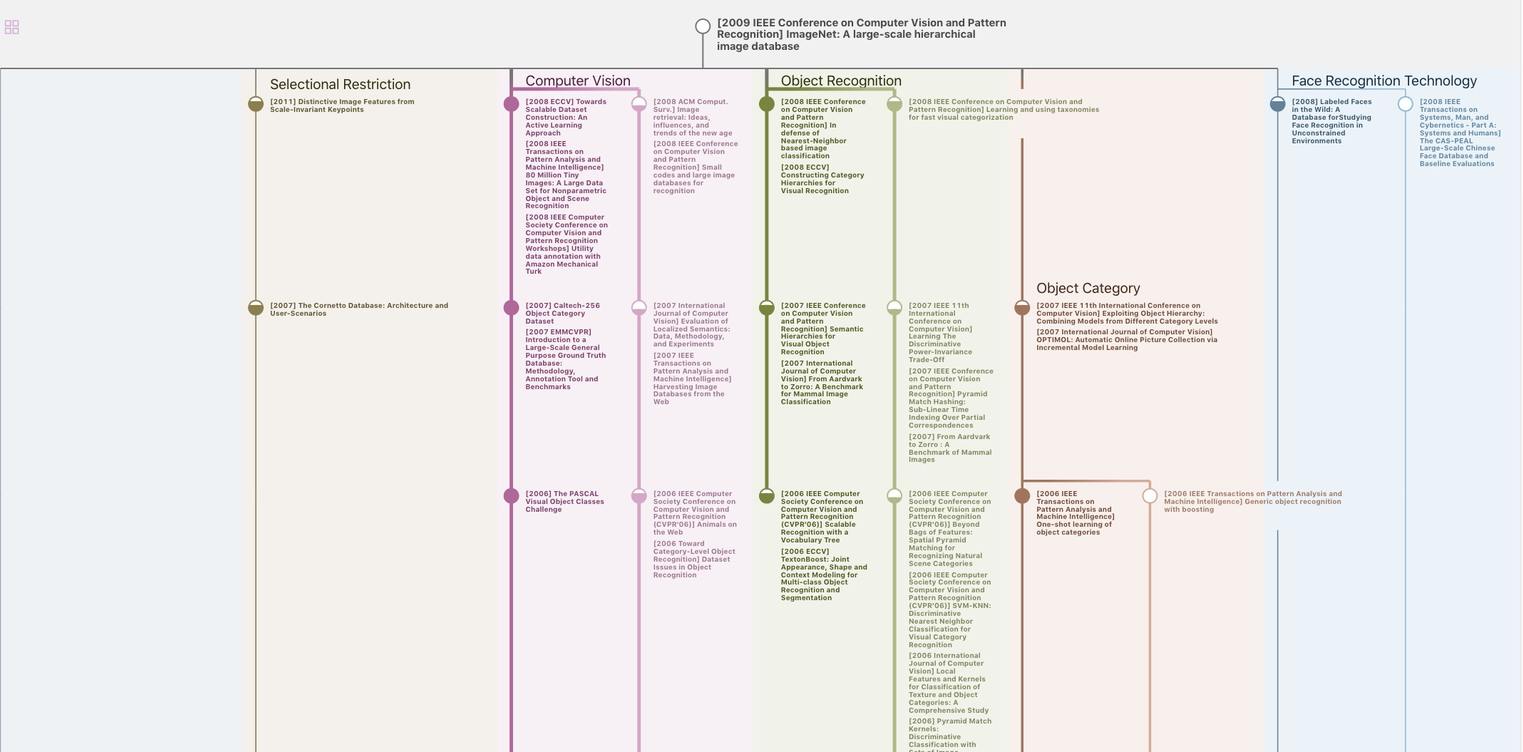
生成溯源树,研究论文发展脉络
Chat Paper
正在生成论文摘要